The solution to mental disorder misdiagnosis may lie in machine learning
Author: Juliana George
Date: 10.21.24
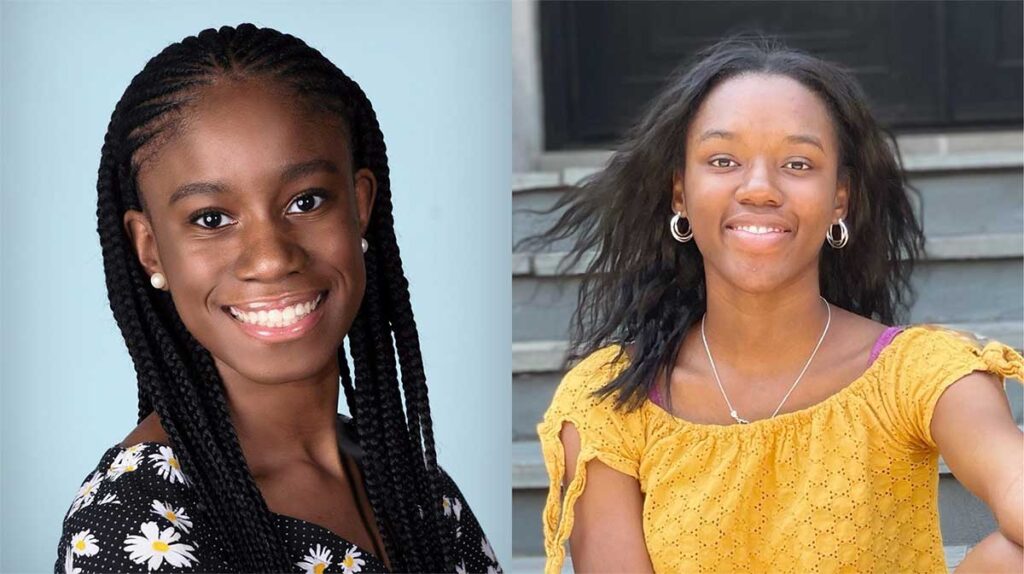
On paper, bipolar disorder (BD) and major depressive disorder (MDD) share many symptoms, including debilitating depressive episodes and low energy. These commonalities result in frequent misdiagnoses, and a key difference between the two makes an incorrect diagnosis disastrous — BD also entails manic episodes of increased activity and recklessness, which are exacerbated by the antidepressants typically used to treat MDD.
Since bipolar patients are more likely to seek treatment for depressive symptoms, they can easily be confused for depressed patients. Luckily, distinctions in the blood biomarkers — blood molecules that can indicate abnormal conditions — of patients with different disorders may hold the solution to pervasive misdiagnosis.
Over the past year, fourth-year undergraduate students Alyssa Lee and Britney Ise Okhiria developed a machine learning model that draws from blood biomarker data to identify which disorder a patient suffers from. Their research culminated in the acceptance of their paper, Enhancing Psychiatric Diagnosis with Machine Learning Models for Blood Biomarker Analysis, at the 2024 IEEE MIT Undergraduate Research Technology Conference (URTC).
“We wanted to use machine learning, which has more power than other statistical methods, to discriminate between the two disorders based on biology rather than symptoms, because symptoms can overlap,” Lee said.
Lee is a behavioral neuroscience major at the College of Science, while Okhiria is a computer science major at Khoury College. The two have been friends since their first year at Northeastern and have wanted to collaborate on a research project for about as long. Then Okhiria saw an opportunity to combine disciplines after taking a class with Fatemeh Ghoreishi, an assistant professor at Khoury College and the College of Engineering. Ghoreishi had come across misdiagnosis problems during a previous project, and once she heard about the pair’s interest in neuroscience, she suggested the topic and agreed to serve as their advisor.
In fall 2023, Lee and Okhiria received a Northeastern PEAK Experiences Ascent Award, which provides $1,500 stipends to support semester-long projects under the guidance of a faculty mentor. Ghoreishi, Lee, and Okhiria all credit the program as an essential research resource.
“We didn’t really have experience with applying or writing research questions, but they had workshops the semester before we applied,” Lee said. “They had assignments to read through the literature and find gaps, and I think having that structure helped us to have an idea of what we wanted to do before we went to Professor Ghoreishi, who helped us create an actual research proposal.”
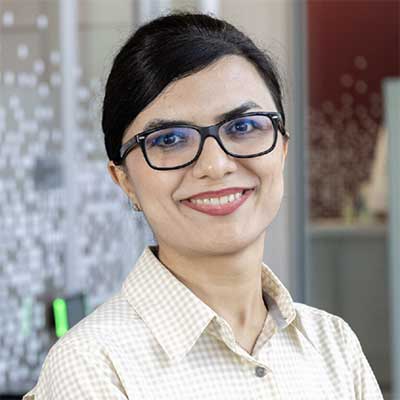
For Ghoreishi, who joined Northeastern in 2021, this was her first experience advising the research of undergraduate students. Her research generally uses machine learning and Bayesian statistics to enhance decision-making and design in complex problems ranging from transportation systems (e.g. autonomous vehicles) to systems biology (e.g. biomarker selection).
“I would like to acknowledge and thank the Northeastern PEAK Award program for its support for this project,” she said. “I enjoyed working with Alyssa and Ise; it was a very good experience for me. The confidence that Ise and Alyssa gained throughout the project and seeing their happiness after the paper’s acceptance gave me a lot of joy.”
The research began with a comprehensive, months-long literature review, during which Lee and Okhiria searched for a data set that would suit their needs. Once they found one, they began writing code for a support vector machine (SVM) algorithm that would classify patients by disorder based on differences in blood biomarkers. SVMs are machine learning models that render data points as coordinates in a higher-dimensional space to classify them into different categories.
“On a normal 2D graph, the slope is the rate of change. But once you get into higher dimensions, the dimension below that will be the rate of change that you need to classify the biomarkers,” Okhiria explained. “We had 106 different proteins that we were looking at, and each of those become a different dimension until we could slowly categorize them into fewer dimensions.”
This process allowed the SVM model to refine itself from 106 dimensions to 30, ensuring that only the biomarkers that best differentiated the disorders would factor into the diagnosis. Lee and Okhiria then created a receiver operating characteristic curve that showed the false positive rate of binary classifier models; by calculating the area under this curve, they concluded that their model distinguished BD from MDD with a 92% accuracy rate.
Lee, Okhiria, and Ghoreishi presented their paper at the MIT URTC in mid-October. With graduation approaching, Lee and Okhiria aren’t planning to continue researching this topic, but are optimistic that their findings represented a step toward reducing the misdiagnosis of psychiatric patients.
“[Our model] is specific to this diagnosis, but it could open the door for more. You could create a model for other mental disorders,” Okhiria said. “I think it could be really useful for therapists to double-check their diagnosis and ask themselves ‘What does the model think? Does it disagree?’ and then make a more informed decision based on that.”