Slow-motion replay is a cornerstone of sports viewing. Now, AI is poised to revolutionize it
Author: Matty Wasserman
Date: 03.18.24
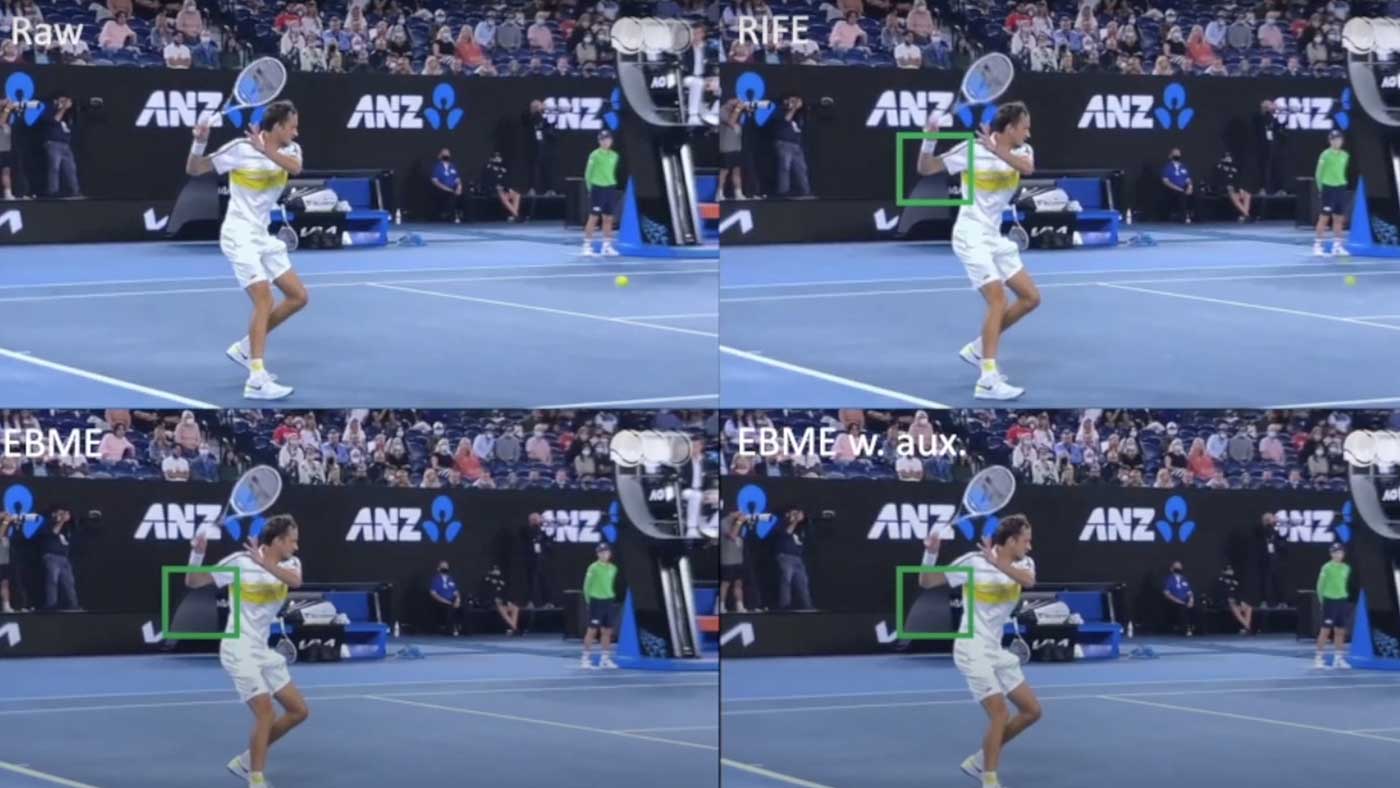
When Huaizu Jiang thinks of where he might apply his marriage of slow-motion AI with sports videography, his daughter’s golf training comes to mind. What if Jiang could film her swing from his phone, set the video in super-slow motion, and her golf coach could analyze every detail without the video ever becoming grainy or choppy, as super-slow videos usually do?
With his new research, Jiang is striving to make that future possible. The Khoury College assistant professor recently co-authored a paper with UC San Diego master’s student Jiaben Chen titled “SportsSloMo: A New Benchmark and Baselines for Human-centric Video Frame Interpolation.” Their work builds on the growing field of slow-motion AI models, which can recreate super-slow video content by “filling in the blanks” of choppy or low-resolution videos, all with full clarity and smoothness. To apply the technology to sports videos, the AI must accurately synthesize and reflect human motions common to physical activity — a uniquely difficult, but promising use case.
“In sports, the main content will be humans. And those fast-moving athletes in the videos pose significant challenges for existing slow-motion AI models,” Jiang said. “The athletes move a lot with their hands, their feet, and their entire body. And there’s heavy interference between different athletes in the same frame.”
These slow-motion AI models have been in development for years, but Jiang’s desire to extend them into the sports sphere dates back to his time as a graduate research assistant at UMass Amherst, where he completed his doctorate in 2020. Shortly after arriving at Northeastern in 2021, he began to focus on the niche area.
“As a professor, you have more freedom to work on what you’re interested in,” Jiang explained. “And this is what I’ve been set on … Understanding human movements in this way is a new challenge for the research community, but it will unlock a lot of applications.”
The technology is still in its early stages, and requires honing before those applications can be made available and usable for everyday consumers. But Jiang sees endless applications — everything from TV broadcasts of live sports to high school recruiting tapes or even just transforming normal action videos.
“Let’s say your friend is doing a cool skateboard trick and you want to check all those stunning details that you can clearly see in slow-motion video,” Jiang said. “But typically, you would just record it in plain view. Later, AI models can help you to recreate those slow motion contents at a professional level.”
Jiang’s study highlighted two key insights to help AI capture the human body during athletic activity. The first is segmentation, where the technology picks out each person and object from the rest of the frame, then tracks their movements. This enables the AI to locate the precise boundaries of each athlete and prevent them from overlapping one another in the slow-motion recreation of the event. Secondly, Jiang incorporated AI technology that can “recover” movements of the human body, even without those movements being visible in the frame. For example, it can estimate the trajectories of human joints, or how a person’s hands or feet would move in a sequence.
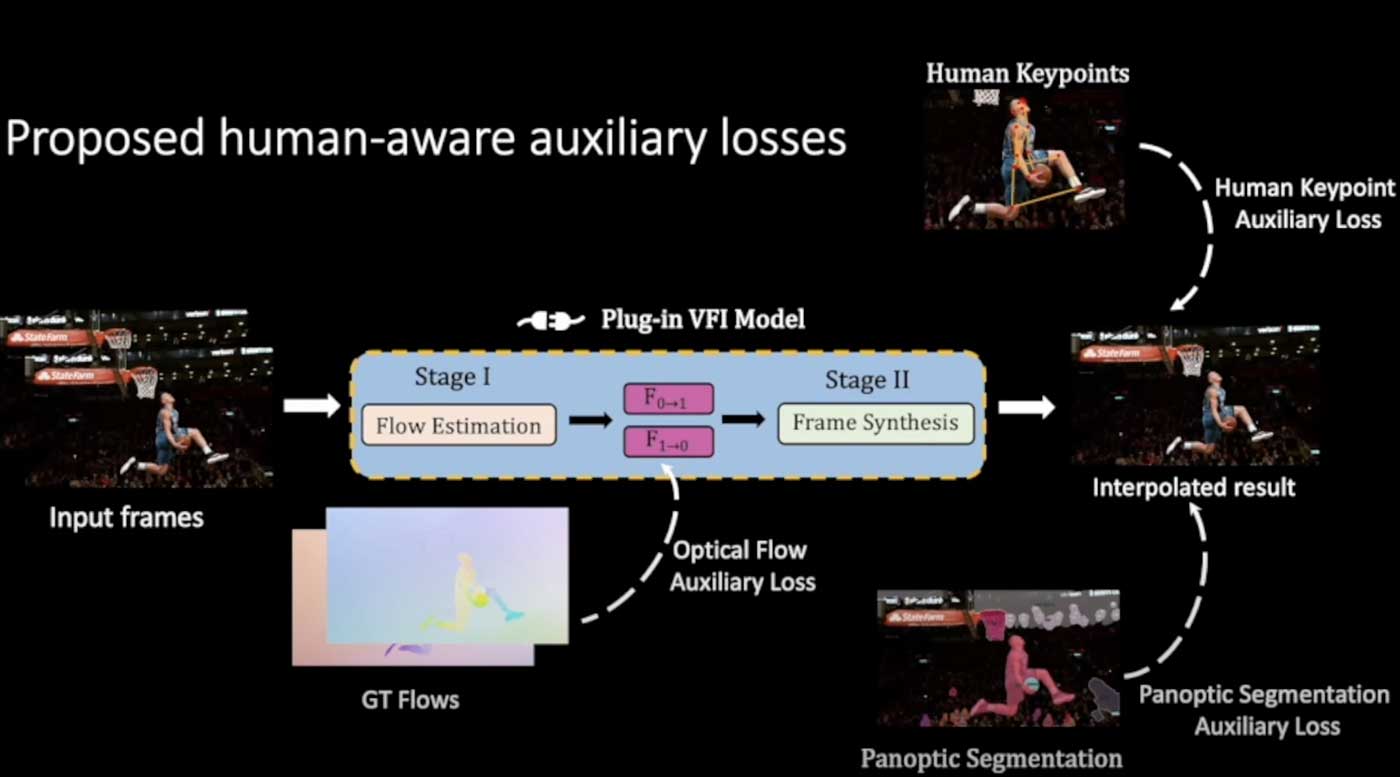
“The idea is that based on those trajectories and understanding each individual person and movement, you can insert human joints in between frames,” Jiang said, “so that the generated videos and slow-motion content will be more authentic.”
While small-scale videos are the simplest application, Jiang envisions a future where the technology is used in large-scale settings. For example, if a sporting event were filmed with just one or two cameras, the AI could create slow-motion replays from angles that the cameras never saw, simply by understanding the dynamics of human movement. Likewise, viewers of live sports could have instant access to multiple slow-motion replay angles, even those that the broadcast did not provide on the air.
Jiang’s new research is just the beginning of his exploration into the topic. His data set is open to other researchers, and he hopes they will also continue to innovate in the exciting new space alongside him.
“Even if you have just one camera or just a couple of cameras scattered around a large field, with this kind of data, we can develop another type of AI technology allowing you to freely watch the game from angles that are not even captured by real devices,” Jiang said. “It’s just synthesis. And that’s really exciting.”
Subscribe to Khoury News
The Khoury Network: Be in the know
Subscribe now to our monthly newsletter for the latest stories and achievements of our students and faculty