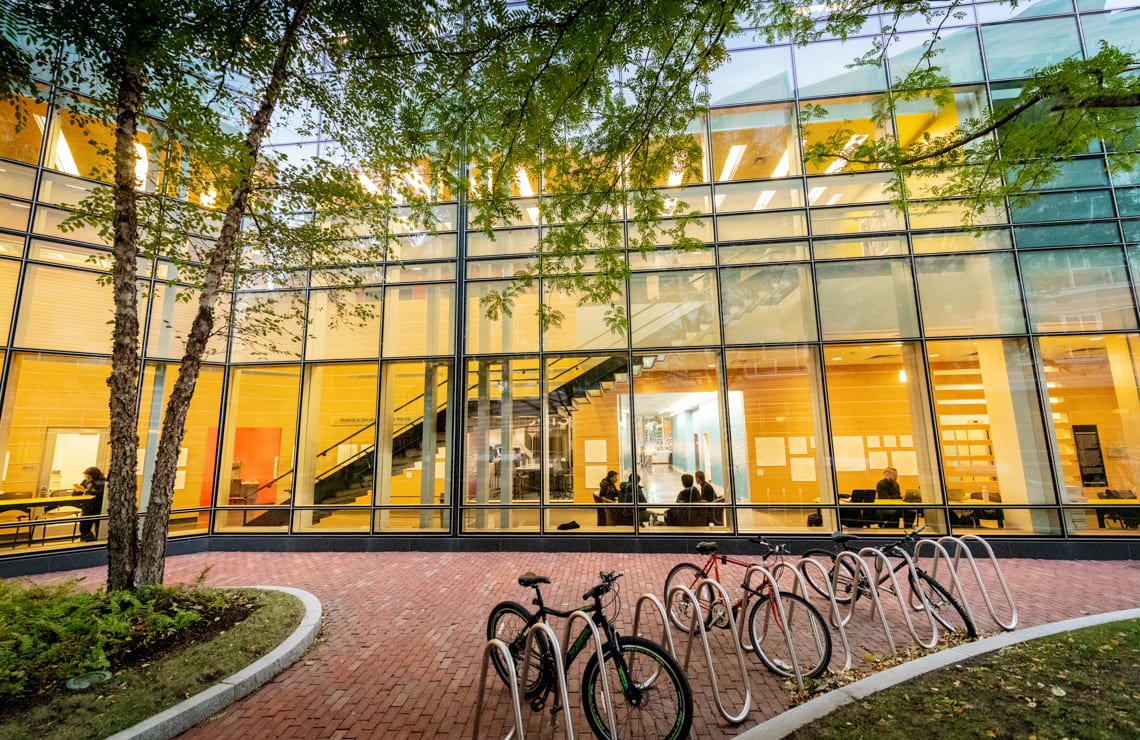
Visualization of Event Sequences for Decision Making
Lead PI
Abstract
Many datasets of interest to scientists, analysts, clinicians, and patients include events that take place over time. Events can come from electronic monitoring devices or manual data entry, and all together form an event sequence. By analyzing event sequences, we can make inferences about complex behaviors over time. Interactive visualization tools are an important tool for human decision makers to use for exploring and understanding data. However, the existing visualization techniques need to be improved to support interpretation of data in applications with a large number of events that vary in frequency, accuracy, and timing. This project will create new visualization encoding and interaction design techniques that will advance the state of the art for understanding long streams of event sequences. Techniques will be validated for general uses as well as in a case study on type 1 diabetes treatment decision support. This research will afford more effective data exploration and decision-making tools for analyzing temporal data, applicable to many domains. The resulting visualization techniques will benefit clinicians and patients performing intensive insulin management for type 1 diabetes by enabling them to reduce the burden of care and improve outcomes. In addition, there are immediate applications to the treatment of chronic heart failure as well as non-health domains such as data centers monitoring cyber security. The outreach efforts in this research will disseminate the findings to the type 1 diabetes community, including both caregivers and patients, as well as encourage teenage girls to pursue careers in STEM.
This research will advance the state of the art in visualization and visual analytics for long streams of temporal event sequences with multidimensional, interrelated data. It will create methodologies for: (1) folding and reconfiguring long event sequences to align by dual non-periodic sentinel events; (2) scaling time axes between dual aligned events to show time distributions; (3) dual-event alignment of overlapping units of folded and reconfigured event sequences with data duplication; (4) compositing data from multiple event sequence sources to enable temporal inference with uncertain, erroneous, and missing data; and (5) displaying rapidly changing time series scalar values composited with point and interval event sequences at different time granularities and at different aggregation levels. The domain case study problem will be characterized and refined through semi-structured interviews with certified diabetes educators (CDEs). The visual encodings and interaction designs will be iteratively designed and evaluated through formative usability studies, visualization expert review, controlled task-based experiments, and qualitative studies with CDEs.
For more information, including relevant publications, visualization software, data analysis tools, documentation, deidentified data from user studies, and deidentified domain data for reproducibility and demonstration, please visit the project website.
Funding
Related Publications
- Yixuan Zhang, Kartik Chanana, and Cody Dunne. “IDMVis: Temporal Event Sequence Visualization for Type 1 Diabetes Treatment Decision Support.” IEEE Transactions on Visualization and Computer Graphics (Proc. Information Visualization 2018), vol. 25, no. 1, 2019-01. doi: 10.1109/TVCG.2018.2865076
- Yixuan Zhang, Sara Di Bartolomeo, Fangfang Sheng, and Cody Dunne. “Evaluating Alignment Approaches in Superimposed Time-Series and Temporal Event Sequence Visualizations.” Proc. IEEE Visualization Conference (VIS), 1 – 5, 2019-10. doi: 10.1109/VISUAL.2019.8933584
- Sara Di Bartolomeo, Aditeya Pandey, Aristotelis Leventidis, David Saffo, Uzma Haque Syeda, Elin Carstensdottir, Magy El-Nasr, Michelle Borkin, and Cody Dunne, “Evaluating the Effect of Timeline Shape on Visualization Task Performance.” Proc. CHI Conference on Human Factors in Computing Systems (CHI ’20), 1 – 12, 2020-04. doi: 10.1145/3313831.3376237. Consumable writeup on Medium.
- Sara Di Bartolomeo, Yixuan Zhang, Fangfang Sheng, and Cody Dunne. “Sequence Braiding: Visual Overviews of Temporal Event Sequences and Attributes”, IEEE Transactions on Visualization and Computer Graphics (Proc. VIS 2020), vol. 27, no. 2, 2021-02. doi: 10.1109/TVCG.2020.3030442