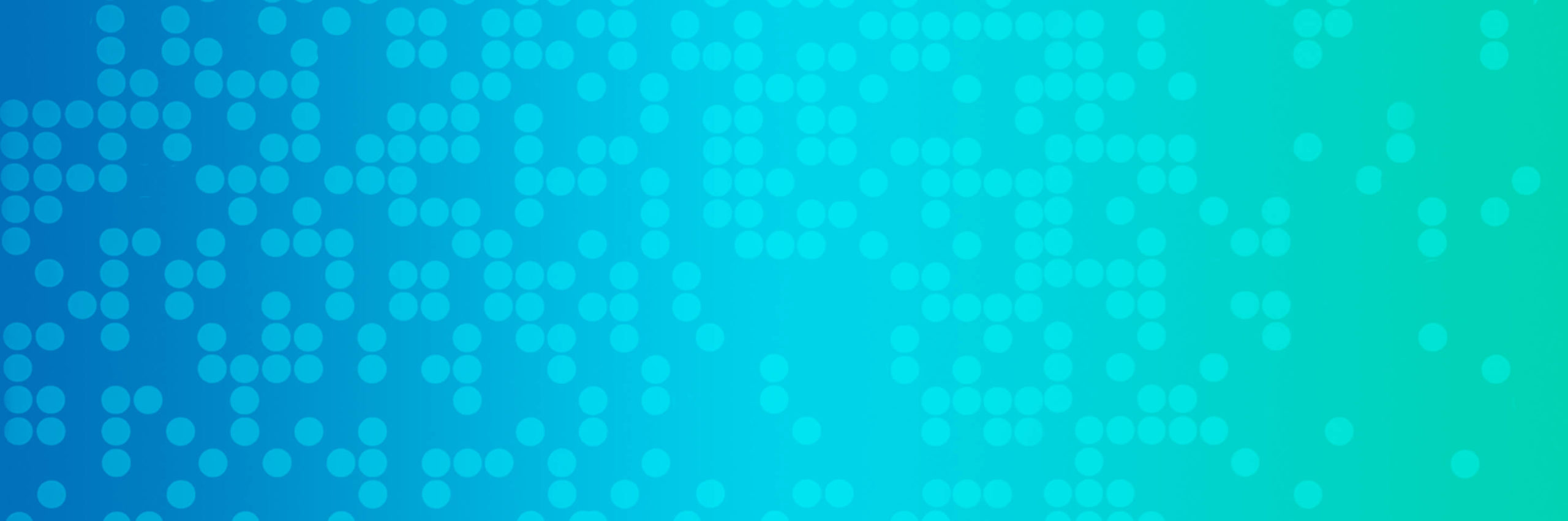
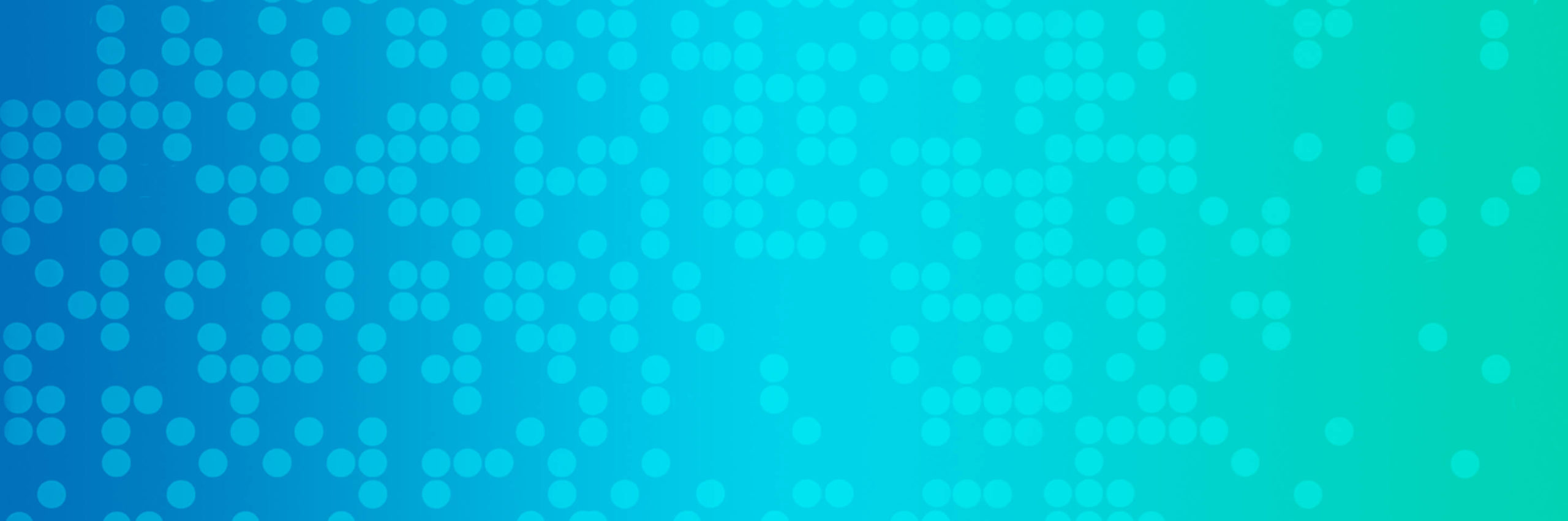
ReCon: Revealing and Controlling Privacy Leaks in Mobile Network Traffic
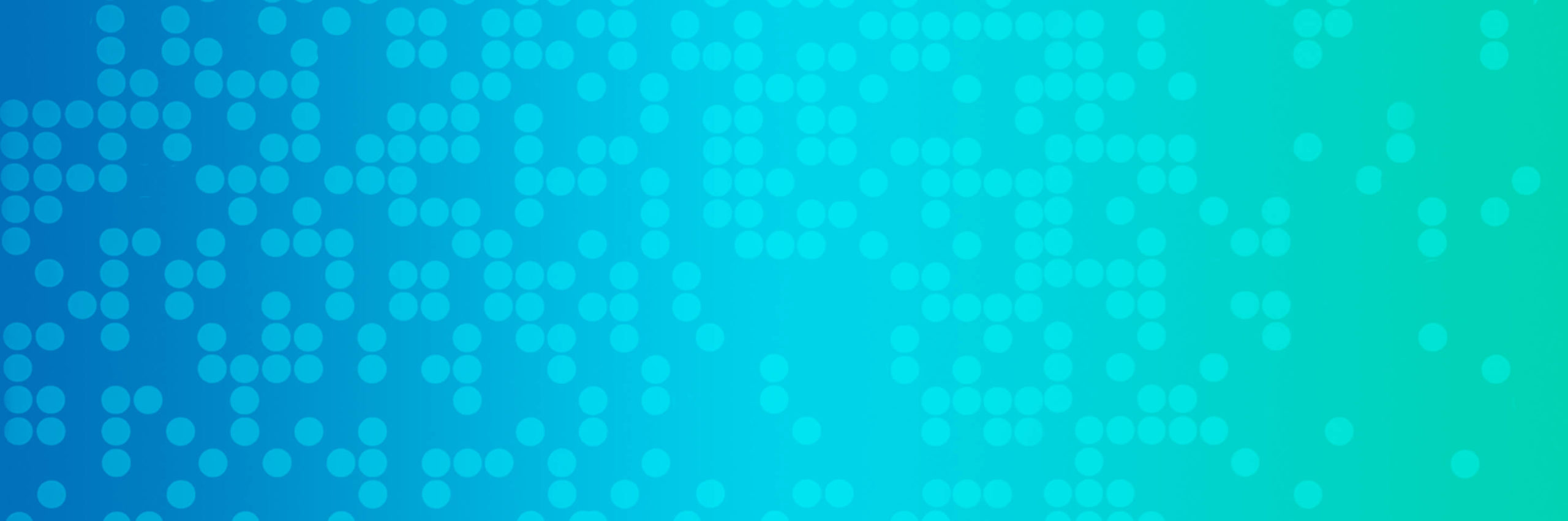
Fri 06.30.17
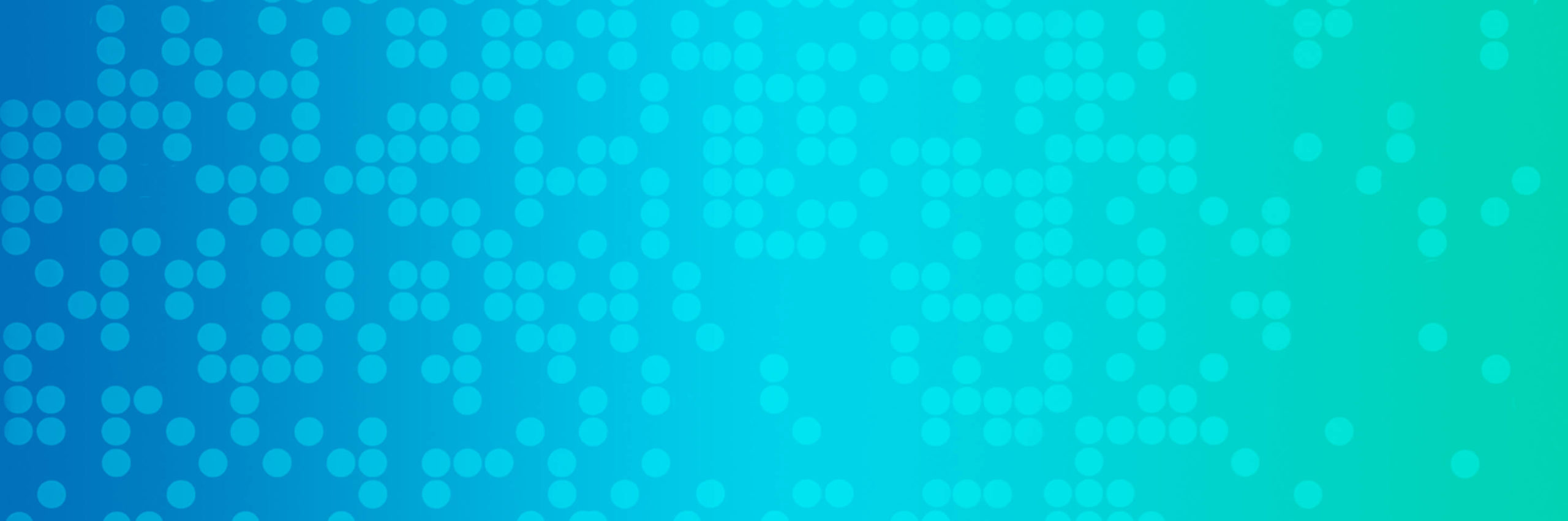
ReCon: Revealing and Controlling Privacy Leaks in Mobile Network Traffic
Fri 06.30.17
Fri 06.30.17
Fri 06.30.17
Fri 06.30.17
Fri 06.30.17
This project focuses on the auditing and control of personally identifiable information leaks, addressing the key challenges of how to identify and control personal identifying information (PII) leaks when users’ PII is not known a priori, nor is the set of apps or devices that leak this information. To enable auditing through improved transparency, we are investigating how to use machine learning to reliably identify PII from network flows, and identify algorithms that incorporate user feedback to adapt to the changing landscape of privacy leaks. We are also investigating the extent to which our approach extends to privacy leaks from IoT devices. Besides adapting our system to the unique format for leaks across a variety of IoT devices, our work investigates PII exposed indirectly through time-series data produced by IoT-generated monitoring.
Using results from those investigations, we are building tools that allow users to control how their information is (or not) shared with other parties. Our tool ReCon analyzes your network traffic to tell if personal information is being transmitted, without needing to know a user’s personal information to work. It detects device/user identifiers used in tracking, geolocation leaks, unsafe password transmissions, and personal information such as name, address, gender, and relationship status. This information is made available to the user via a private Web page, and allows them to tell us if we found important leaks, and whether we should block or modify the leaks.
DHS, Comcast Innovation Fund, AWS Cloud Credits for Research
This project focuses on the auditing and control of personally identifiable information leaks, addressing the key challenges of how to identify and control personal identifying information (PII) leaks when users’ PII is not known a priori, nor is the set of apps or devices that leak this information. To enable auditing through improved transparency, we are investigating how to use machine learning to reliably identify PII from network flows, and identify algorithms that incorporate user feedback to adapt to the changing landscape of privacy leaks. We are also investigating the extent to which our approach extends to privacy leaks from IoT devices. Besides adapting our system to the unique format for leaks across a variety of IoT devices, our work investigates PII exposed indirectly through time-series data produced by IoT-generated monitoring.
Using results from those investigations, we are building tools that allow users to control how their information is (or not) shared with other parties. Our tool ReCon analyzes your network traffic to tell if personal information is being transmitted, without needing to know a user’s personal information to work. It detects device/user identifiers used in tracking, geolocation leaks, unsafe password transmissions, and personal information such as name, address, gender, and relationship status. This information is made available to the user via a private Web page, and allows them to tell us if we found important leaks, and whether we should block or modify the leaks.
DHS, Comcast Innovation Fund, AWS Cloud Credits for Research