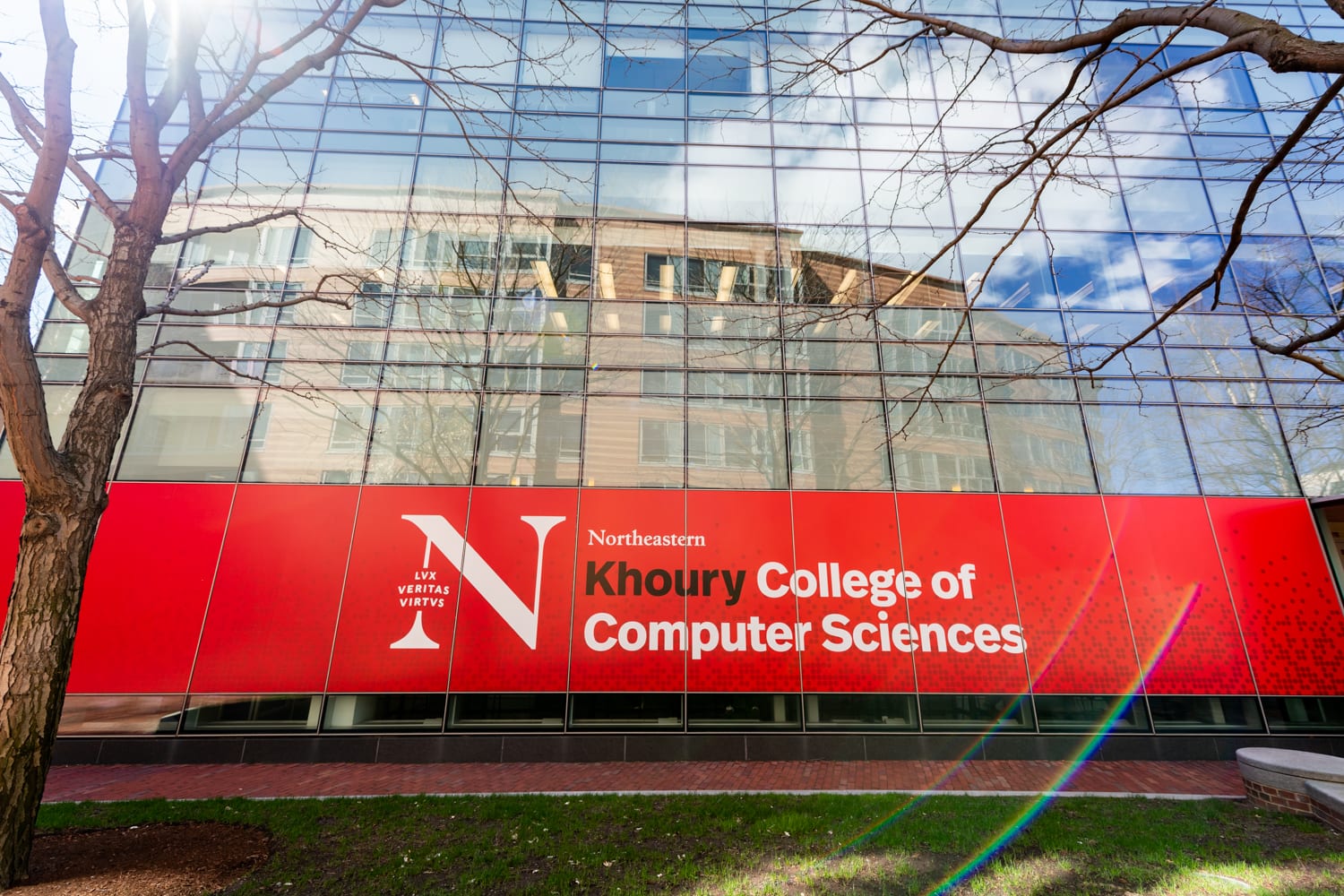
Mobots
Lead PI
Co PI
- Seth Cooper
- Aditya Ponnada
Abstract
Robust activity recognition algorithms depend on how well their training datasets have been prepared. An important part of preparing this training data set is to have high-quality annotations/labels on the entire dataset. However, if the dataset gets bigger and bigger, adding annotations to it becomes even more cumbersome for the research team. Often, these tasks are done manually on small datasets by researchers. In order to solve this computational problem, we have designed “Mobots” – a human-computation (i.e. crowdsourcing) game to annotate large accelerometer datasets (e.g., NHANES and UK BioBank datasets). In Mobots, players are shown snippets of accelerometer data that they match with a lab-based ground truth data (as shown below). This way, we can gather annotations on a very large dataset with the quality of lab-based ground truth.
For more information and to watch our detailed CHI PLAY presentation, visit the project page.
(Credit: Aditya Ponnada)
Funding
Related publications
- Aditya Ponnada, Seth Cooper, Binod Thapa-Chhetry, Josh Aaron Miller, Dinesh John, and Stephen Intille. 2019. Designing Videogames to Crowdsource Accelerometer Data Annotation for Activity Recognition Research. In Proceedings of the Annual Symposium on Computer-Human Interaction in Play (CHI PLAY ’19). Association for Computing Machinery, New York, NY, USA, 135–147. DOI: 10.1145/3311350.3347153