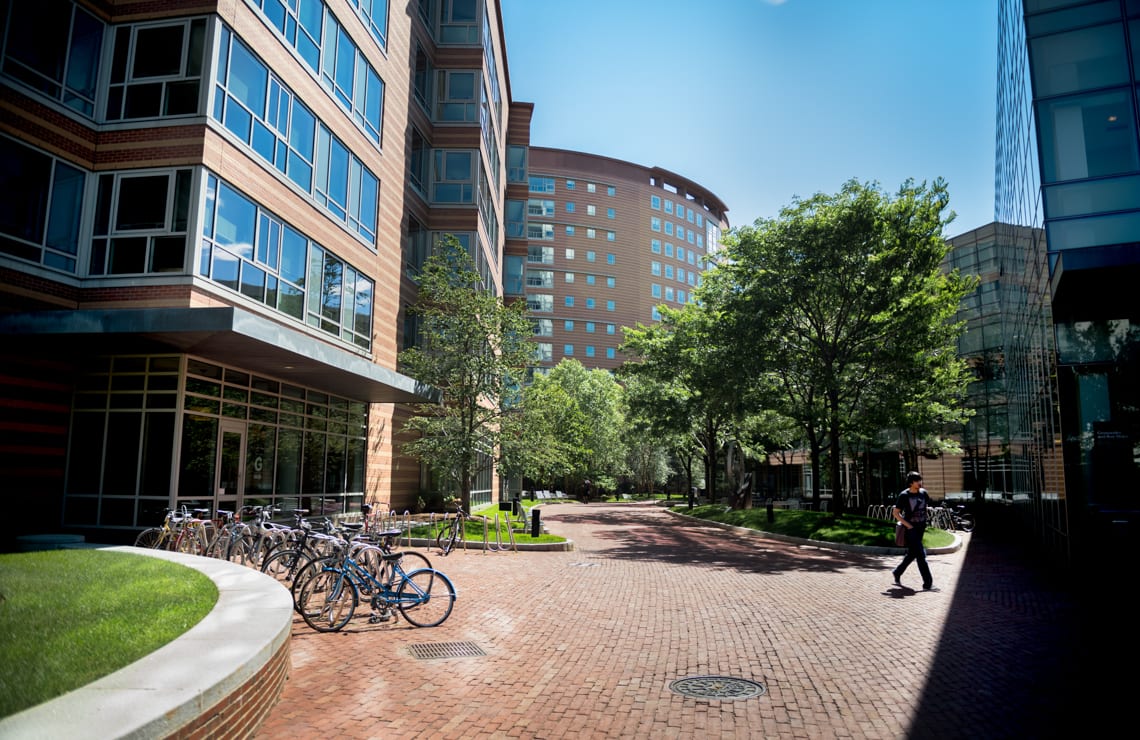
Crowd-Sourced Annotation of Longitudinal Sensor Data to Enhance Data-Driven Precision Medicine for Behavioral Health
Lead PI
Co PIs
Abstract
Longitudinal sensor data collected passively from wearable activity monitors and mobile phones will transform behavioral science by allowing researchers to use “big data,” but at the person-level, to understand how behavior and related environmental exposures impact health outcomes and personalize health intervention and research. We have been exploring whether typical mobile application game players might help scientists improve this type of data, by adding additional annotations that enrich the data, making it more useful for behavioral science and more amenable to automatic processing. This could help researchers to better understand how individual-level behaviors relate to health outcomes in current research studies that collect personal-level sensor data such as the NHANES, and future big data ventures such as the All of Us study.