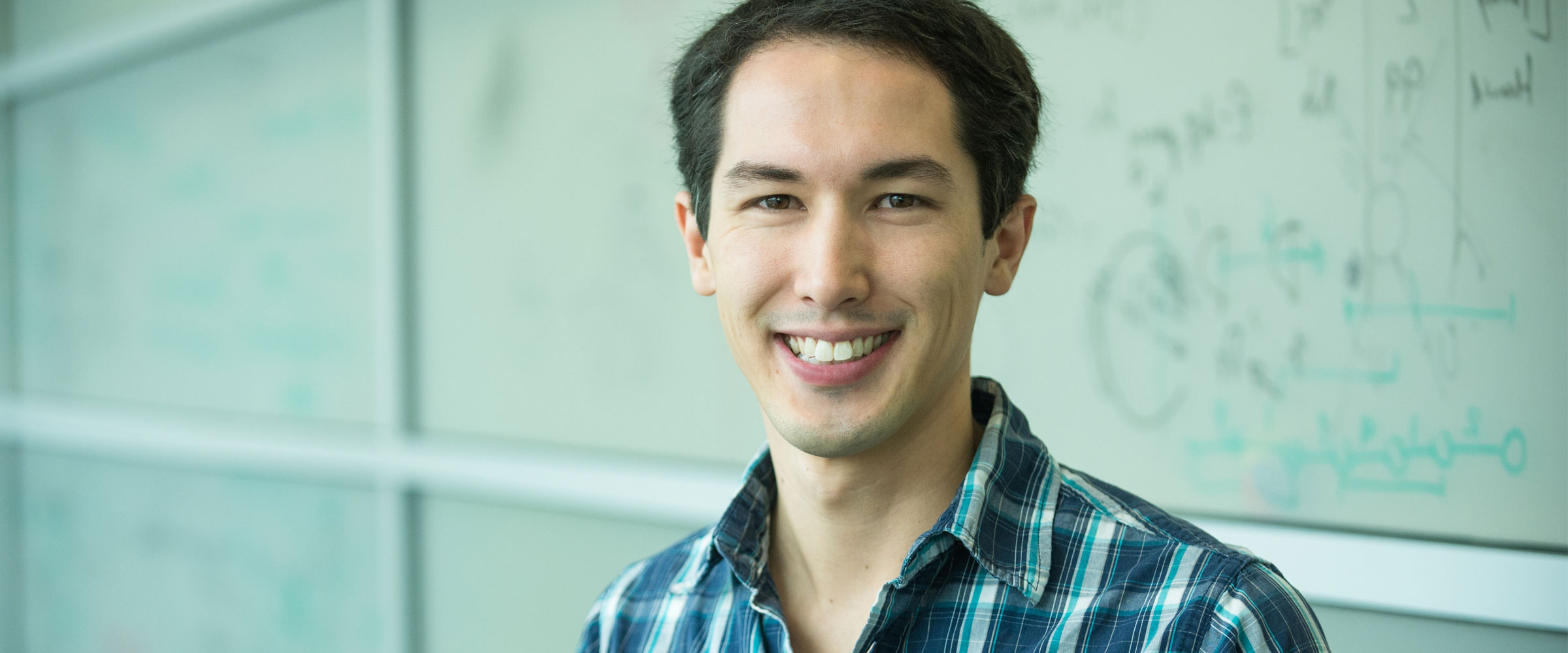
Matthew Ekstrand-Abueg
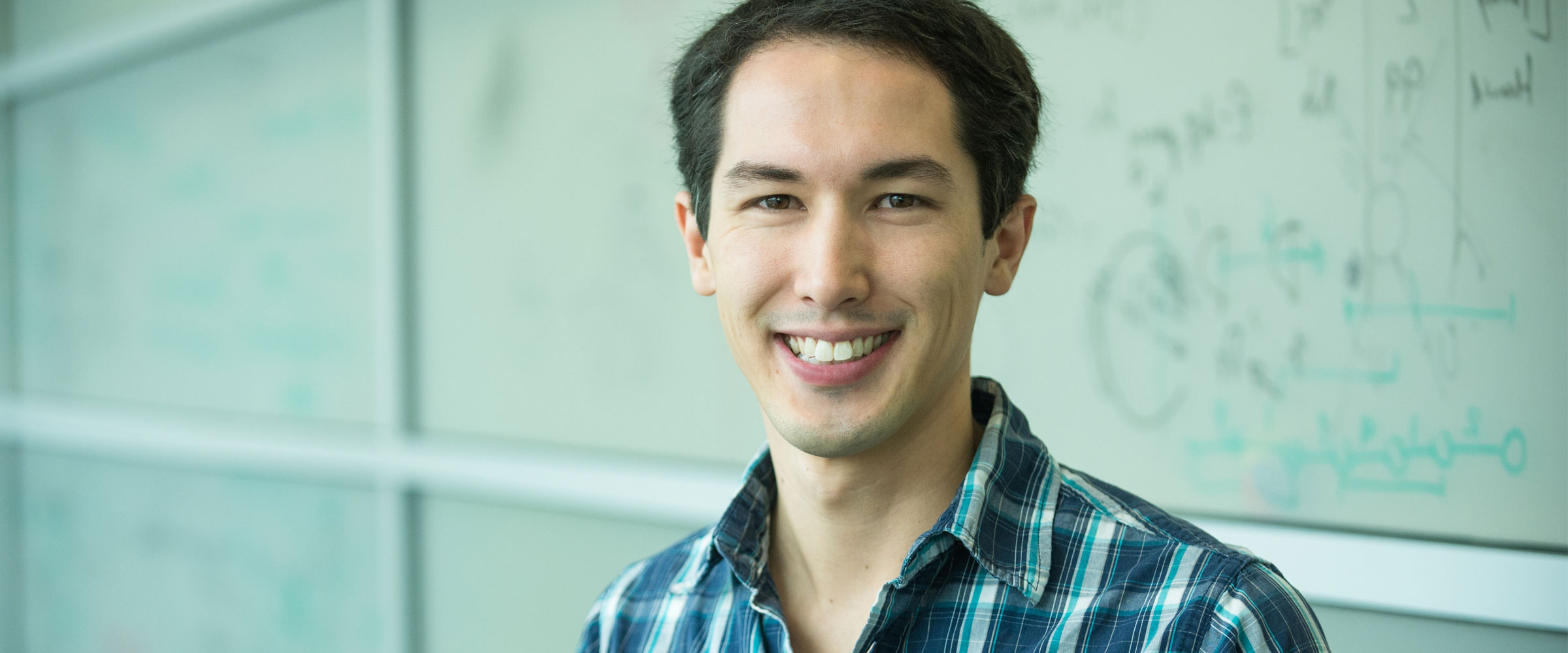
Matthew Ekstrand-Abueg is a PhD student at Northeastern University’s College of Computer and Information Science, advised by Professor Javed Aslam. He focuses on information retrieval and summarization, with an interest in using natural language processing and statistical methods to retrieve text. Matthew seeks to make the vast amount of information on the internet more directly and quickly accessible to users, while maintaining individualization and diversity. He earned his bachelor’s degree in electrical engineering and computer science at the University of California, Berkeley, and his master’s degree in computer science at Northeastern.
My main focus involves semi-supervised test collection creation for document retrieval and many areas of summarization, including time-constrained, multi-document, and space-constrained settings. As a side project, I have worked on mouse tracking for information salience to analyze the cost of annoying advertisements, automated parsing of news stories for bad entities, medical forum entity extraction, and technical advising for a tablet-based medical study in India, some of these projects being done while on internships at Microsoft Research and Google Research.
I am interested in applying natural language processing and statistical methods to web-scale data for text retrieval, rather than document retrieval. I have worked with the Text Retrieval Conference at the National Institute of Standards and Technology and NTCIR at the National Institute of Informatics in Japan for several years to create datasets for summarization evaluation and simultaneously encourage work around the world to tackle these problems, especially in temporal summarization and summarization for mobile devices.
I am trying to solve the problem of improving the reusability of test collections given the scale of the internet and difficulties in matching natural language text. To that end, I am trying to improve automatic matching of text for similarity and entailment using Neuro-Linguistic Programming, statistical methods such as word vectors, and learning algorithms to match the manually-identified gold set of information to arbitrary text returned by a summarization system.
The most interesting aspect of my work is the ever-present battle of the way we represent the formalisms of language versus the statistical methods for teaching computers to understand language using large quantities of data but limited supervision. Both sides have shown positive results, and I believe they will both be necessary for computers to truly understand language, but we have yet to unify them.
My current goal is to create evaluation collections and methodologies to serve as learning objectives for current and future learning methods to measurably improve summarization systems in a wide variety of areas. More broadly, I aim to make the vast amount of information on the internet more directly and quickly accessible to users, including through personalization and summarization, but maintaining diversification of content. I also love to teach, having instructed numerous courses at Northeastern.
Matthew Ekstrand-Abueg is a PhD student at Northeastern University’s College of Computer and Information Science, advised by Professor Javed Aslam. He focuses on information retrieval and summarization, with an interest in using natural language processing and statistical methods to retrieve text. Matthew seeks to make the vast amount of information on the internet more directly and quickly accessible to users, while maintaining individualization and diversity. He earned his bachelor’s degree in electrical engineering and computer science at the University of California, Berkeley, and his master’s degree in computer science at Northeastern.
My main focus involves semi-supervised test collection creation for document retrieval and many areas of summarization, including time-constrained, multi-document, and space-constrained settings. As a side project, I have worked on mouse tracking for information salience to analyze the cost of annoying advertisements, automated parsing of news stories for bad entities, medical forum entity extraction, and technical advising for a tablet-based medical study in India, some of these projects being done while on internships at Microsoft Research and Google Research.
I am interested in applying natural language processing and statistical methods to web-scale data for text retrieval, rather than document retrieval. I have worked with the Text Retrieval Conference at the National Institute of Standards and Technology and NTCIR at the National Institute of Informatics in Japan for several years to create datasets for summarization evaluation and simultaneously encourage work around the world to tackle these problems, especially in temporal summarization and summarization for mobile devices.
I am trying to solve the problem of improving the reusability of test collections given the scale of the internet and difficulties in matching natural language text. To that end, I am trying to improve automatic matching of text for similarity and entailment using Neuro-Linguistic Programming, statistical methods such as word vectors, and learning algorithms to match the manually-identified gold set of information to arbitrary text returned by a summarization system.
The most interesting aspect of my work is the ever-present battle of the way we represent the formalisms of language versus the statistical methods for teaching computers to understand language using large quantities of data but limited supervision. Both sides have shown positive results, and I believe they will both be necessary for computers to truly understand language, but we have yet to unify them.
My current goal is to create evaluation collections and methodologies to serve as learning objectives for current and future learning methods to measurably improve summarization systems in a wide variety of areas. More broadly, I aim to make the vast amount of information on the internet more directly and quickly accessible to users, including through personalization and summarization, but maintaining diversification of content. I also love to teach, having instructed numerous courses at Northeastern.