Shantanu Jain
(he/him/his)
Associate Research Scientist
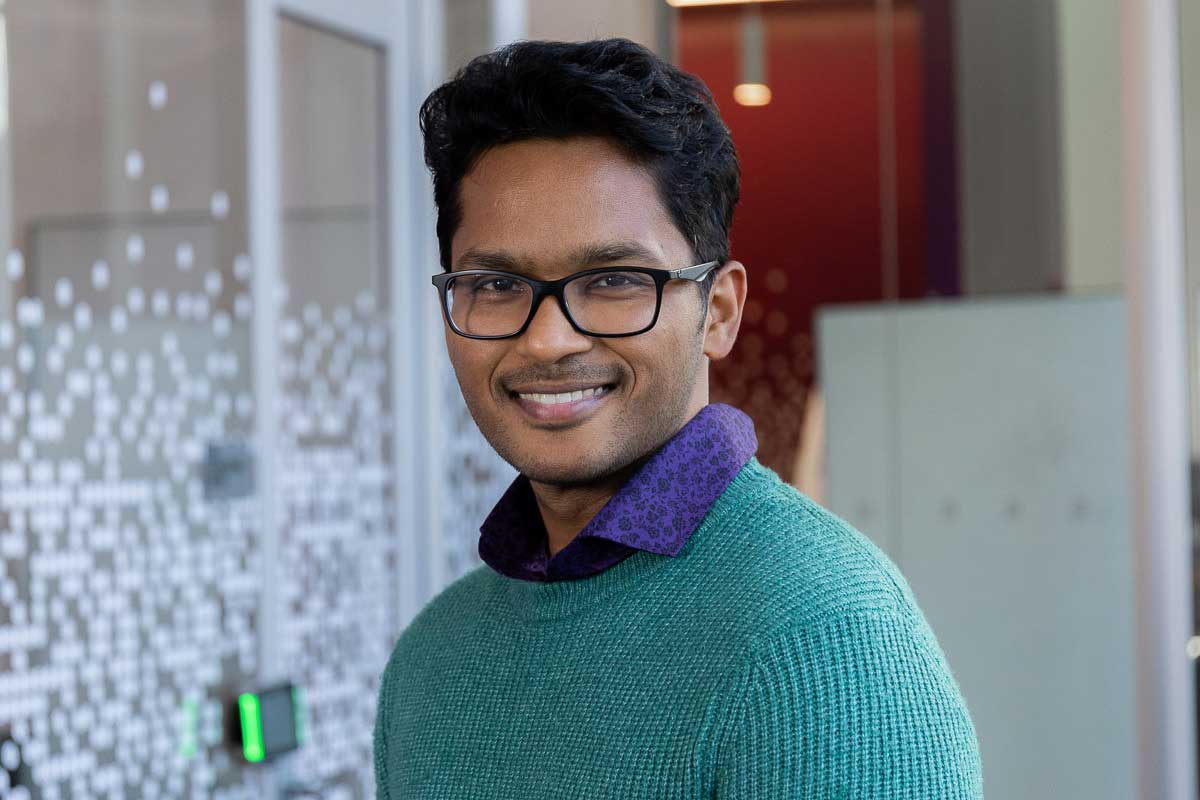
Research interests
- Machine learning
- Artificial intelligence
- Data science
- Statistics
Education
- PhD in Computer Science, Indiana University
- MS in Computer Science, Indiana University
- MS in Applied Statistics, Indiana University
- B-Tech in Computer Engineering, Nirma University — India
Biography
Shantanu Jain is an associate research scientist in the Khoury College of Computer Sciences at Northeastern University. He is interested in the field of statistical modeling and machine learning. Jain’s research focuses on developing semi-supervised methods under data constraints for which standard approaches lead to biased estimates.
Prior to joining Northeastern in 2018, Jain received his doctorate and master’s in computer science from Indiana University. His recent work has addressed issues in binary classification and its evaluation that arise due to the absence of labeled examples from one of the classes (positive-unlabeled learning) and incorrectly labeled examples and bias in the labeled examples. Jain’s research has been applied to many bioinformatics problems and mass spectrometry data, as well as published in journals including AAAI, Pacific Symposium on Biocomputing, and the Scandinavian Journal of Statistics. Outside of research, Jain enjoys solving puzzles, singing, and dancing.
Recent publications
-
An algorithm for decoy-free false discovery rate estimation in XL-MS/MS proteomics
Citation: Yisu Peng, Shantanu Jain, Predrag Radivojac. (2024). An algorithm for decoy-free false discovery rate estimation in XL-MS/MS proteomics Bioinform., 40, i428-i436. https://doi.org/10.1093/bioinformatics/btae233 -
Class Prior Estimation with Biased Positives and Unlabeled Examples
Citation: Jain S, Delano J, Sharma H, Radivojac P. Class Prior Estimation with Biased Positives and Unlabeled Examples. In Proceedings of the AAAI Conference on Artificial Intelligence 2020 Apr 3 (Vol. 34, No. 04, pp. 4255-4263). doi:10.1609/aaai.v34i04.5848 -
Estimating classification accuracy in positive-unlabeled learning: characterization and correction strategies
Citation: Ramola R, Jain S, Radivojac P. Estimating classification accuracy in positive-unlabeled learning: characterization and correction strategies. Pac. Symp. Biocomput. (2019) 24: 124-135. -
Identifiability of two‐component skew normal mixtures with one known component
Citation: Jain S, Levine M, Radivojac P, Trosset MW. Identifiability of two-component skew normal mixtures with one known component. Scand. J. Stat. (2019). -
Recovering true classifier performance in positive-unlabeled learning
Citation: Jain S, White M, Radivojac P. Recovering true classifier performance in positive-unlabeled learning. AAAI Conference on Artificial Intelligence, AAAI 2017, pp. 2066-2072, San Francisco, California, U.S.A., February 2017. -
Estimating the class prior and posterior from noisy positives and unlabeled data
Citation: Jain S, White M, Radivojac P. Estimating the class prior and posterior from noisy positives and unlabeled data. Advances in Neural Information Processing Systems, NIPS 2016, pp. 2693-2701, Barcelona, Spain, December 2016. -
The loss and gain of functional amino acid residues is a common mechanism causing human inherited disease.
Citation: Lugo-Martinez J, Pejaver V, Pagel KA, Jain S, Mort M, Cooper DN, Mooney SD, Radivojac P. The loss and gain of functional amino acid residues is a common mechanism causing human inherited disease. PLoS Comput. Biol. (2016) 12(8): e1005091. -
Nonparametric semi-supervised learning of class
Citation: Jain S, White M, Trosset MW, Radivojac P. Nonparametric semi-supervised learning of class proportions. (2016) arXiv:1601.01944.