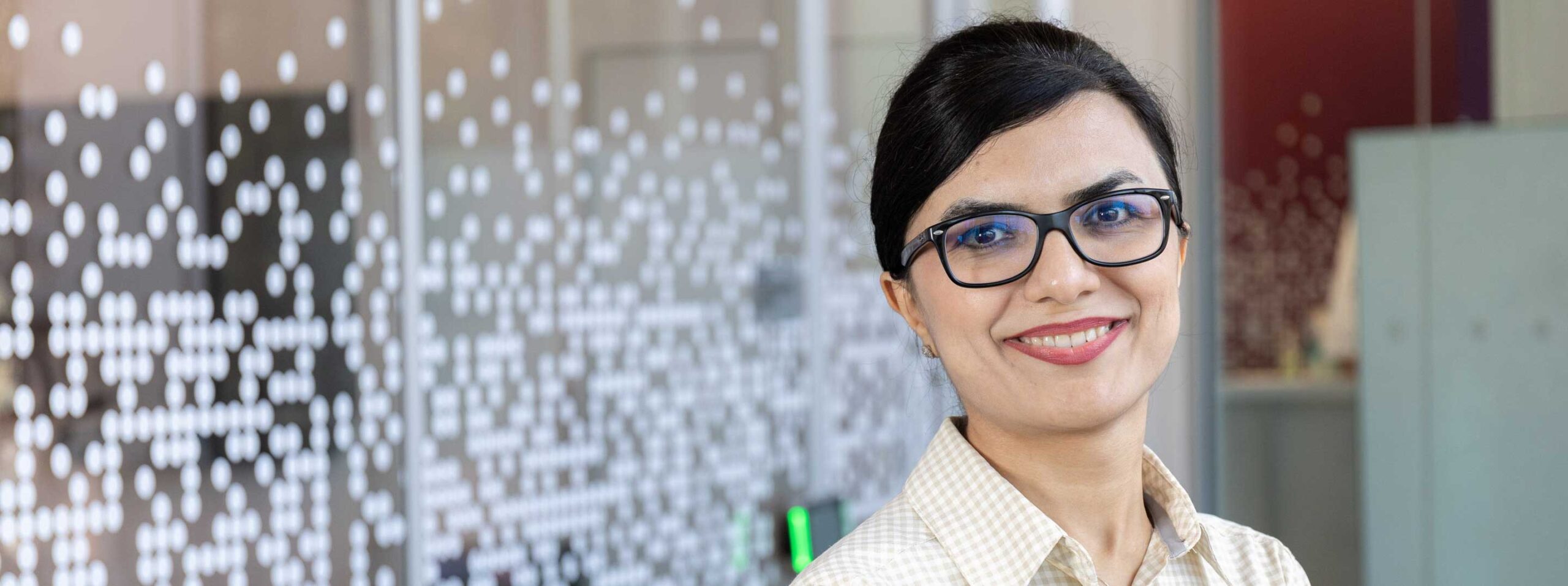
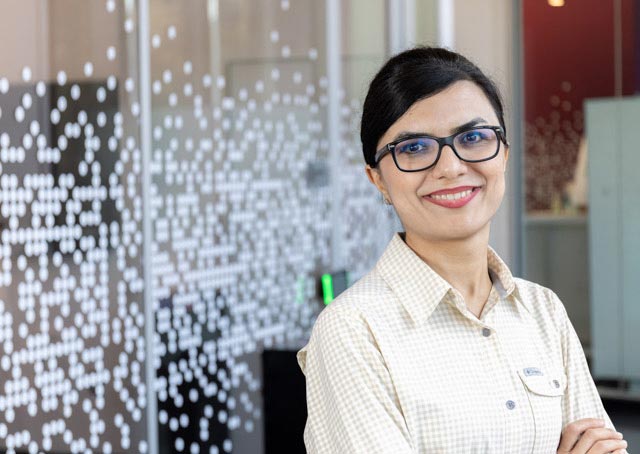
Fatemeh Ghoreishi
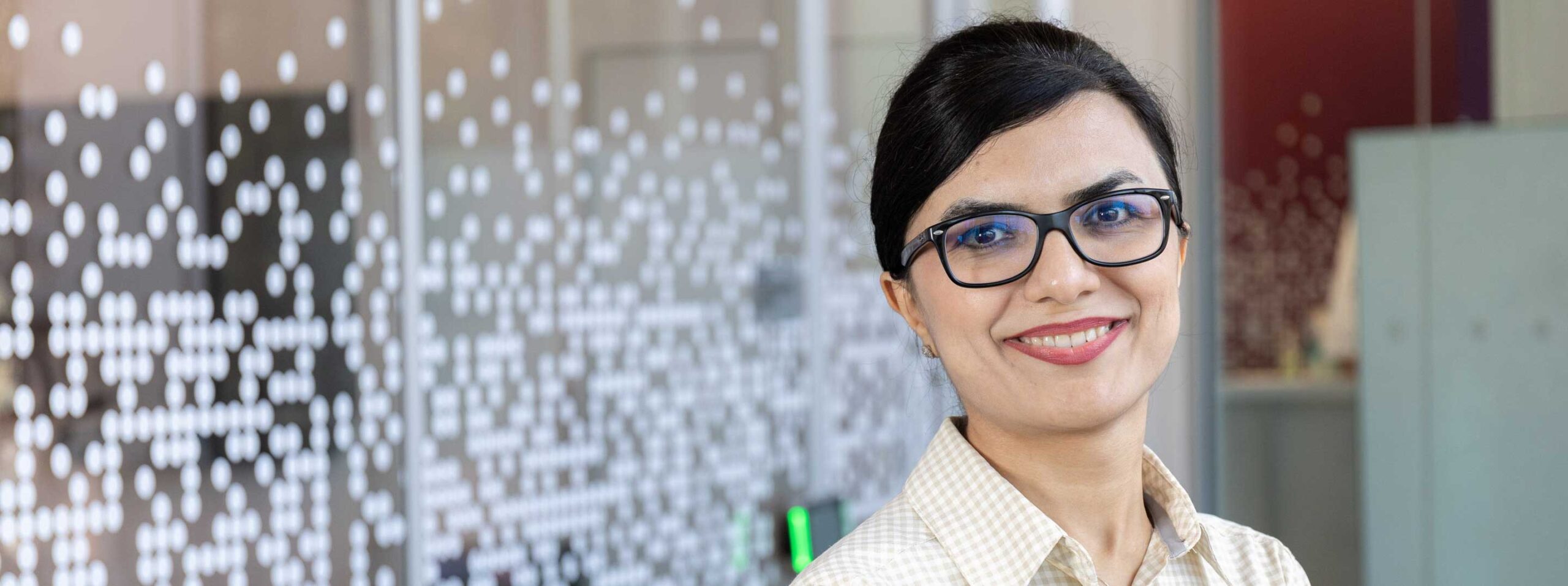
Assistant Professor
Interdisciplinary With COE
Boston
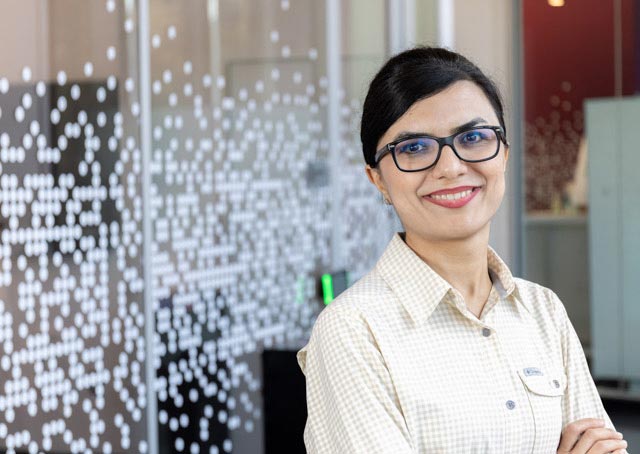
Fatemeh Ghoreishi
Assistant Professor
Interdisciplinary With COE
Boston
Assistant Professor
Interdisciplinary With COE
Boston
Assistant Professor
Interdisciplinary With COE
Boston
Assistant Professor
Interdisciplinary With COE
Boston
Assistant Professor
Interdisciplinary With COE
Boston
she/her/hers
Seyede Fatemeh Ghoreishi is an assistant professor in the Department of Civil and Environmental Engineering and the Khoury College of Computer Sciences at Northeastern University. She teaches machine learning, as well as probabilities and statistics.
Before joining Northeastern in 2021, Ghoreishi was a postdoctoral research fellow at the University of Maryland’s Institute for Systems Research. During her time there, she was selected for the Rising Stars in Mechanical Engineering in 2019 (at the University of Texas at Austin) and 2020 (at the University of California, Berkeley).
Ghoreishi’s research focuses on machine learning and Bayesian statistics for design and decision-making under uncertainty, and she has given talks and led workshops on these subjects at IEEE conferences, Virginia Tech, Georgia Tech, Penn State University, the University of Southern California, the University of Wisconsin-Madison, and the University of California San Diego. She also co-chaired the 2021 American Control Conference.
she/her/hers
Seyede Fatemeh Ghoreishi is an assistant professor in the Department of Civil and Environmental Engineering and the Khoury College of Computer Sciences at Northeastern University. She teaches machine learning, as well as probabilities and statistics.
Before joining Northeastern in 2021, Ghoreishi was a postdoctoral research fellow at the University of Maryland’s Institute for Systems Research. During her time there, she was selected for the Rising Stars in Mechanical Engineering in 2019 (at the University of Texas at Austin) and 2020 (at the University of California, Berkeley).
Ghoreishi’s research focuses on machine learning and Bayesian statistics for design and decision-making under uncertainty, and she has given talks and led workshops on these subjects at IEEE conferences, Virginia Tech, Georgia Tech, Penn State University, the University of Southern California, the University of Wisconsin-Madison, and the University of California San Diego. She also co-chaired the 2021 American Control Conference.