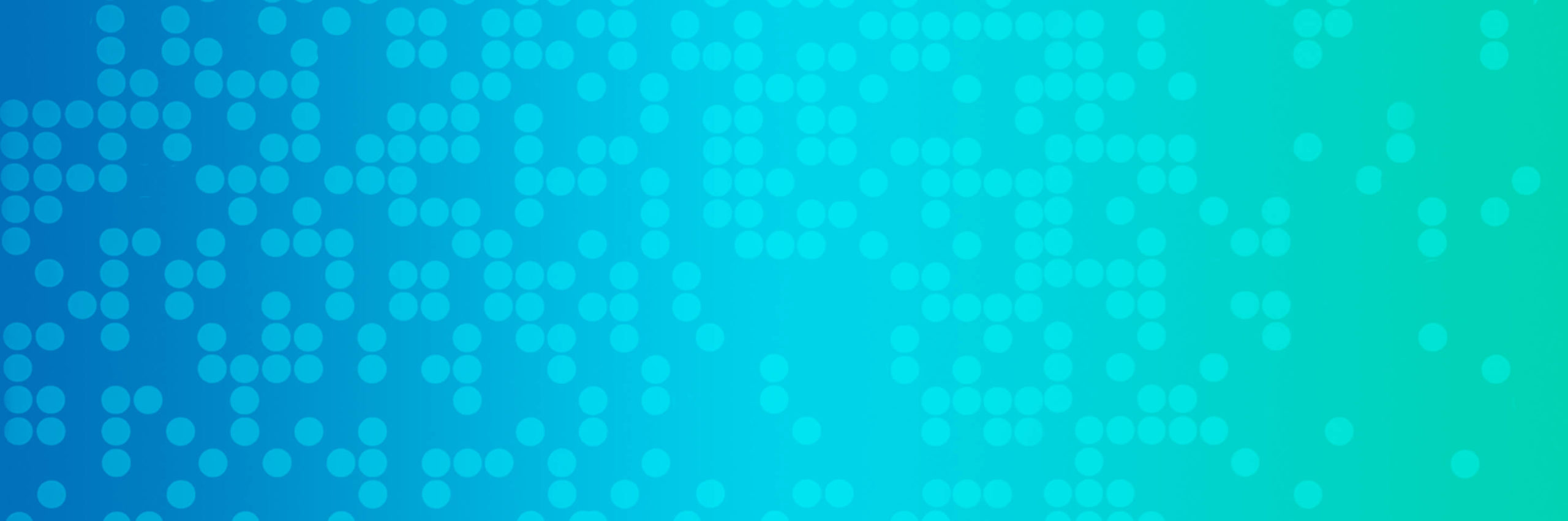
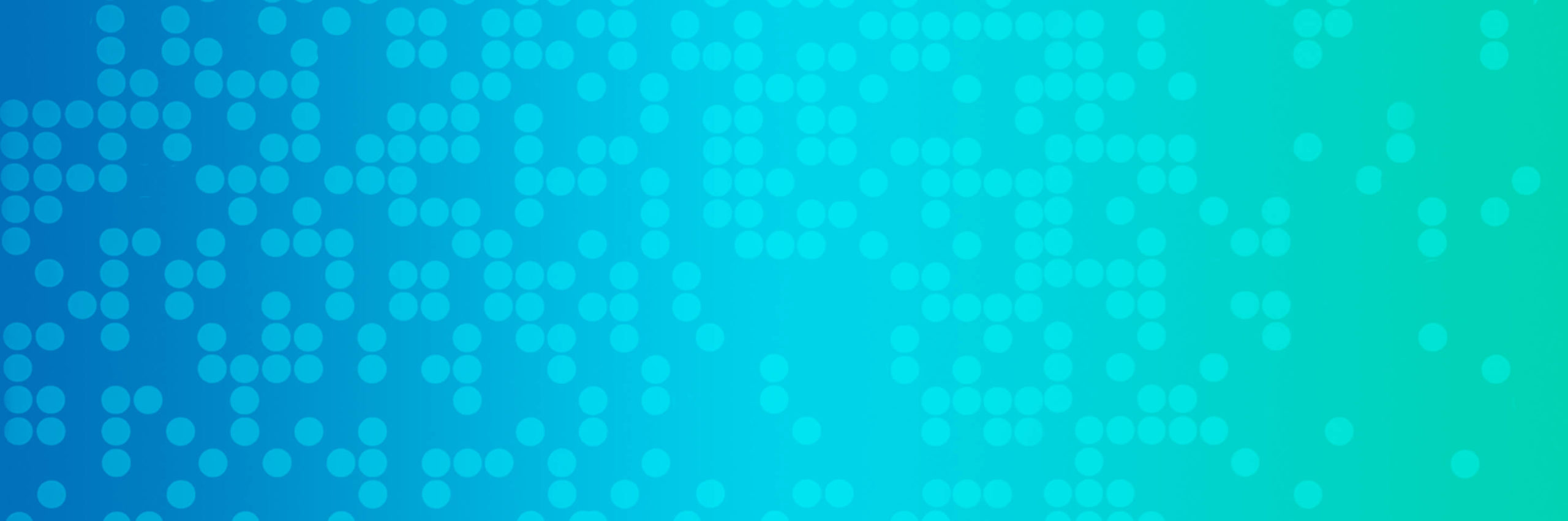
In the age of ChatGPT, AI models are massively popular … and easily compromised
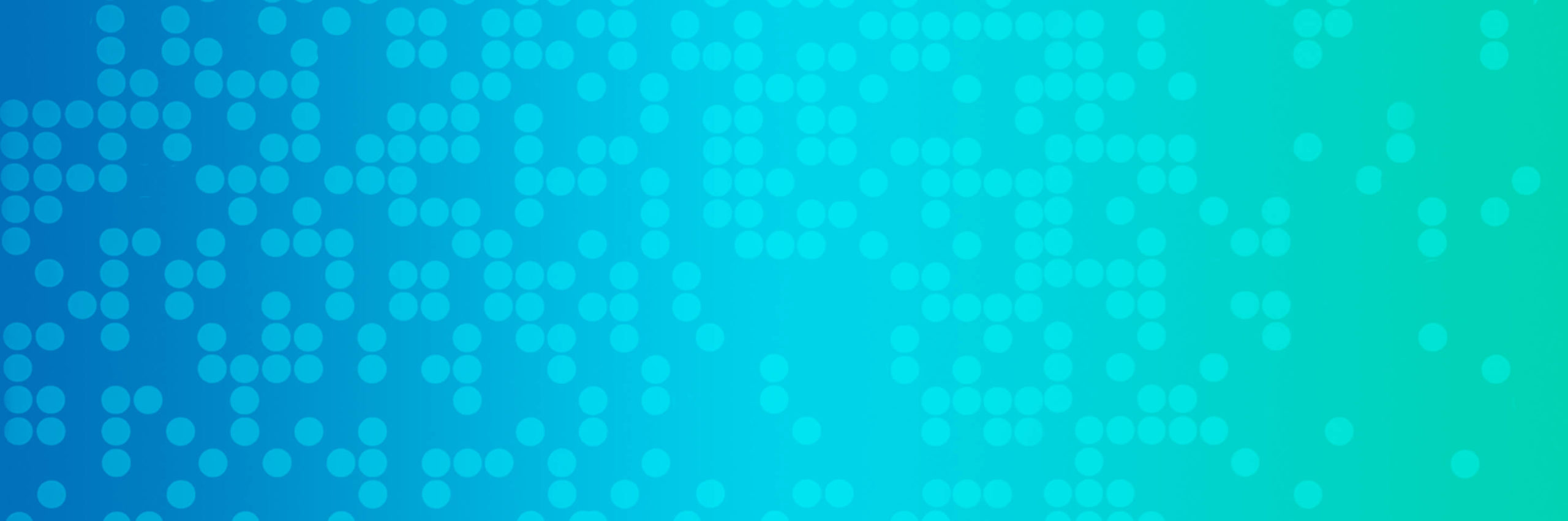
Thu 02.15.24 / Milton Posner
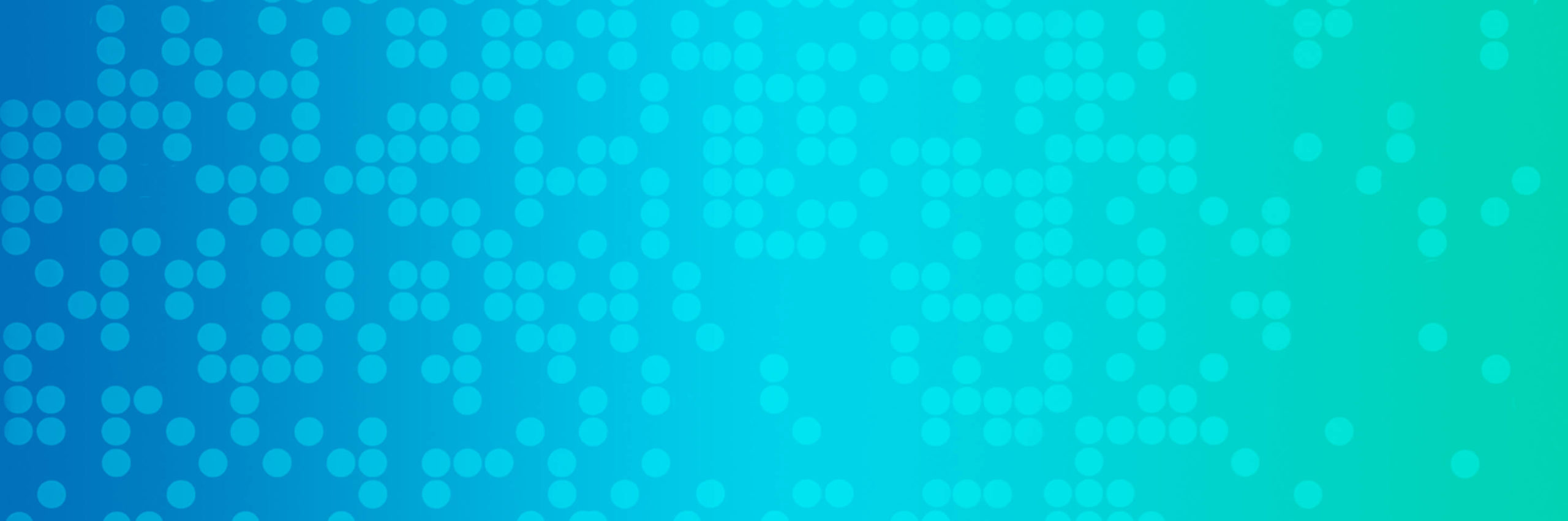
In the age of ChatGPT, AI models are massively popular … and easily compromised
Thu 02.15.24 / Milton Posner
Thu 02.15.24 / Milton Posner
Thu 02.15.24 / Milton Posner
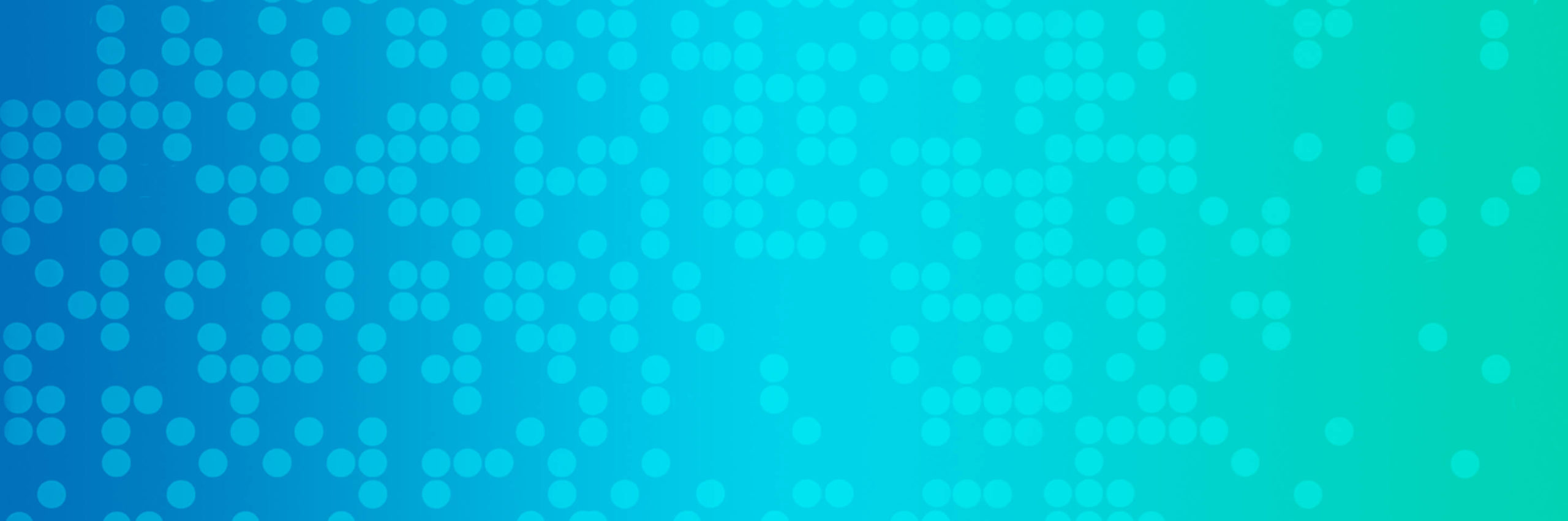
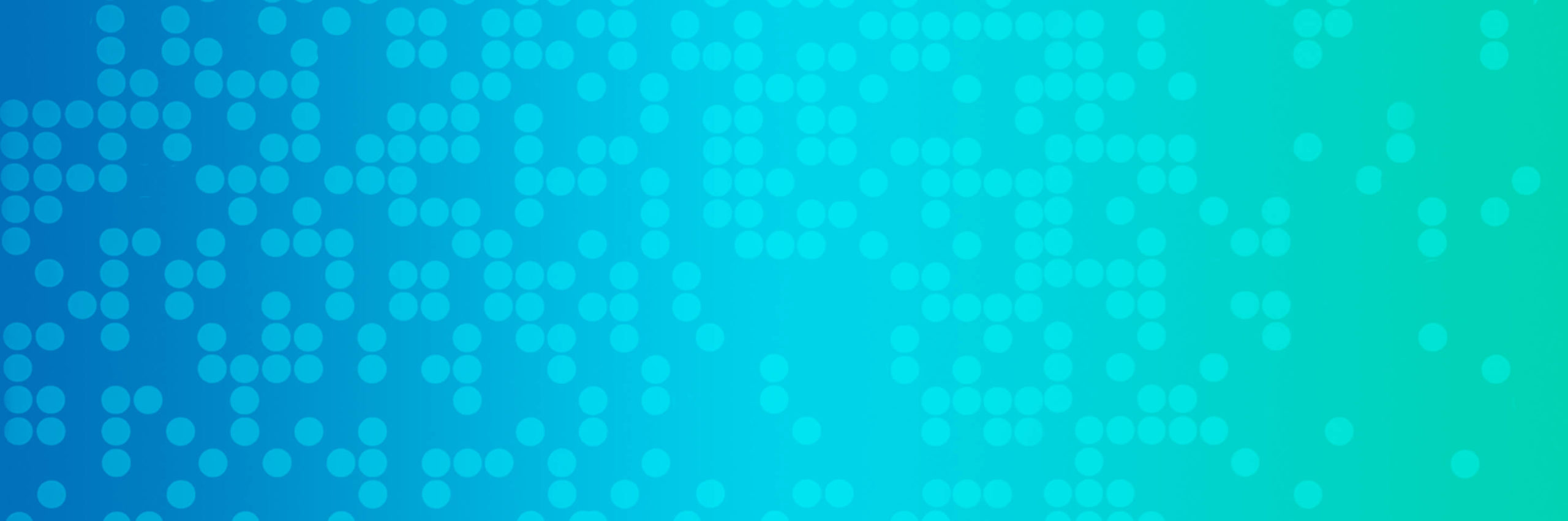
In the age of ChatGPT, AI models are massively popular … and easily compromised
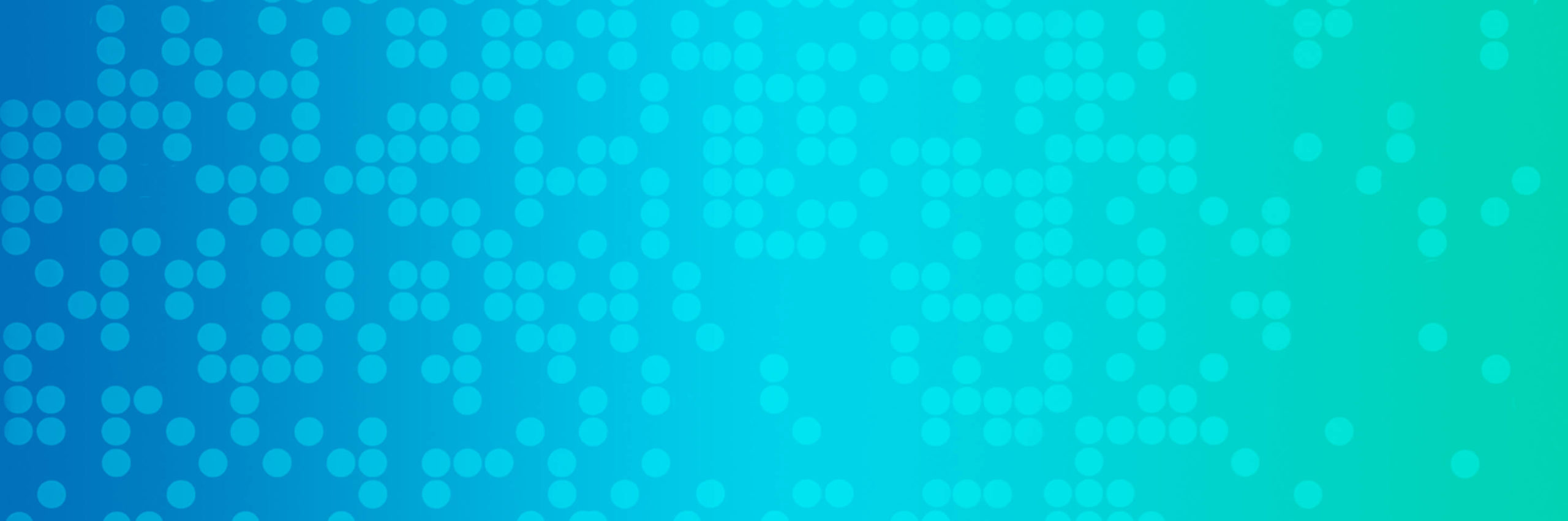
Thu 02.15.24 / Milton Posner
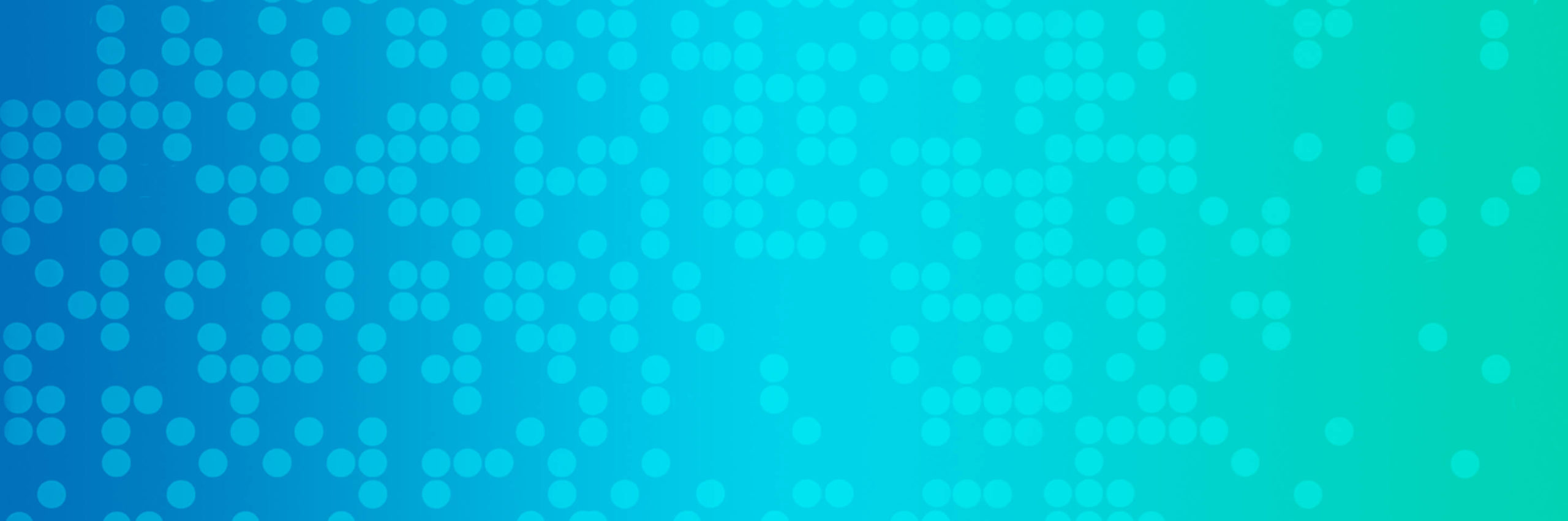
In the age of ChatGPT, AI models are massively popular … and easily compromised
Thu 02.15.24 / Milton Posner
Thu 02.15.24 / Milton Posner
Thu 02.15.24 / Milton Posner
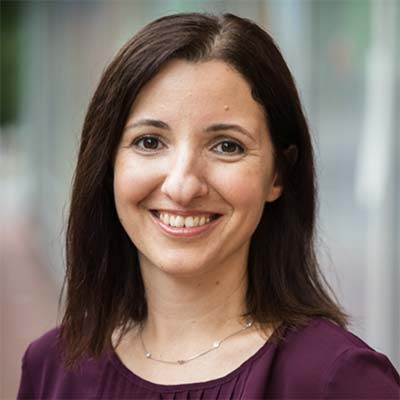
Long before 2023 ended, it had already been crowned as the year of generative AI. Spurred by the advent of models like ChatGPT that produced detailed, jarringly human replies to user prompts, experts and novices alike began musing on the technology’s potential impacts on work, education, and creativity.
But while today’s large language models (LLMs) are stunningly capable, they’re also shockingly vulnerable, says Khoury professor Alina Oprea. She’s been studying AI in a cybersecurity context for more than a decade, and recently co-authored a report that delves into these attacks on AI — how they work, how they’re classified, and how they can (and can’t) be mitigated.
“It’s really difficult to keep generative AI secure,” Oprea says. “The scale of these models and their training data will grow over time, which only makes these attacks easier. And once you start talking about generative AI that goes beyond text to images and speech, security becomes a very open question.”
The report, published by the Department of Commerce’s National Institute of Standards and Technology (NIST), is an update of the report Oprea co-authored last year with NIST’s Apostol Vassilev. That initial report dealt with more traditional predictive AI, but with generative AI exploding in popularity since then, Opera and Vassilev welcomed generative AI experts Alie Fordyce and Hyrum Anderson from Robust Intelligence to expand the project’s remit.
“Now we have academics, government, and industry working together,” Oprea noted, “which is the intended audience for the report.”
According to the report, generative AI models owe their vulnerability to a variety of factors. For one, Oprea notes, most attacks are “fairly easy to mount and require minimal knowledge of the AI system.” For another, the models’ enormous training data sets are too large for humans to monitor and validate. And the code underpinning the models isn’t automated; it relies on human moderation and is exposed to malicious human meddling.
The upshot, say the quartet of researchers, is four major types of attacks that confuse AI systems and cause them to malfunction: evasion attacks that alter the model’s inputs to change its responses, poisoning attacks that corrupt the model’s underlying algorithms or training data, privacy attacks that coax the model into revealing sensitive training data such as medical information, and abuse attacks that feed incorrect information into legitimate sources that the model learns from. By manipulating the model’s inputs, attackers can choose its outputs in advance.
“This can be used for commercial purposes, for advertisement, for generating malware spam or hate speech — things the model wouldn’t usually generate,” Oprea explains.
Without overtaxing themselves, malicious actors can control the web data an AI model trains on, introduce a backdoor, and then stealthily steer the model’s behavior from there. Given the exploding popularity of these models, such backdoors would be concerning enough on their own. But the damage doesn’t stop there.
“We now have these integrated applications that use LLMs. For example, a company builds an email agent that integrates with an LLM in the background, and it can now read your emails and send emails on your behalf,” Oprea says. “But attackers could use the same tool to send malware and spam to thousands of people. The attack surface has increased because we’re integrating LLMs into these applications.”
As destructive and dangerous as hate speech and mass spam are, there are even bigger security concerns on the horizon.
“Some applications are safety-critical, like self-driving cars,” Oprea says. “If those models make incorrect predictions, they can’t be used.”
So what can be done? The team prepared the report, which they plan to update annually, for a few audiences — policymakers, AI developers, and academics who can use the report’s taxonomy as a foundation or context for their own work. All of these groups, Oprea says, have work to do to ensure that AI models align to human values, preserve privacy, and operate in the best interest of users. But she acknowledges that addressing every issue raised in the report is challenging, and that anyone hawking solutions rather than mitigations is sorely mistaken.
“There are many more attacks than mitigations, and for every mitigation we mention, there is a tradeoff or a performance overhead, including degradation of model accuracy,” Oprea cautions. “The mitigations don’t come for free and securing AI is a really challenging endeavor, but we hope that the report provides a useful starting point for understanding the attacks.”
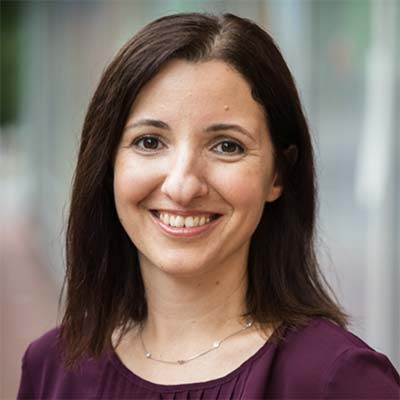
Long before 2023 ended, it had already been crowned as the year of generative AI. Spurred by the advent of models like ChatGPT that produced detailed, jarringly human replies to user prompts, experts and novices alike began musing on the technology’s potential impacts on work, education, and creativity.
But while today’s large language models (LLMs) are stunningly capable, they’re also shockingly vulnerable, says Khoury professor Alina Oprea. She’s been studying AI in a cybersecurity context for more than a decade, and recently co-authored a report that delves into these attacks on AI — how they work, how they’re classified, and how they can (and can’t) be mitigated.
“It’s really difficult to keep generative AI secure,” Oprea says. “The scale of these models and their training data will grow over time, which only makes these attacks easier. And once you start talking about generative AI that goes beyond text to images and speech, security becomes a very open question.”
The report, published by the Department of Commerce’s National Institute of Standards and Technology (NIST), is an update of the report Oprea co-authored last year with NIST’s Apostol Vassilev. That initial report dealt with more traditional predictive AI, but with generative AI exploding in popularity since then, Opera and Vassilev welcomed generative AI experts Alie Fordyce and Hyrum Anderson from Robust Intelligence to expand the project’s remit.
“Now we have academics, government, and industry working together,” Oprea noted, “which is the intended audience for the report.”
According to the report, generative AI models owe their vulnerability to a variety of factors. For one, Oprea notes, most attacks are “fairly easy to mount and require minimal knowledge of the AI system.” For another, the models’ enormous training data sets are too large for humans to monitor and validate. And the code underpinning the models isn’t automated; it relies on human moderation and is exposed to malicious human meddling.
The upshot, say the quartet of researchers, is four major types of attacks that confuse AI systems and cause them to malfunction: evasion attacks that alter the model’s inputs to change its responses, poisoning attacks that corrupt the model’s underlying algorithms or training data, privacy attacks that coax the model into revealing sensitive training data such as medical information, and abuse attacks that feed incorrect information into legitimate sources that the model learns from. By manipulating the model’s inputs, attackers can choose its outputs in advance.
“This can be used for commercial purposes, for advertisement, for generating malware spam or hate speech — things the model wouldn’t usually generate,” Oprea explains.
Without overtaxing themselves, malicious actors can control the web data an AI model trains on, introduce a backdoor, and then stealthily steer the model’s behavior from there. Given the exploding popularity of these models, such backdoors would be concerning enough on their own. But the damage doesn’t stop there.
“We now have these integrated applications that use LLMs. For example, a company builds an email agent that integrates with an LLM in the background, and it can now read your emails and send emails on your behalf,” Oprea says. “But attackers could use the same tool to send malware and spam to thousands of people. The attack surface has increased because we’re integrating LLMs into these applications.”
As destructive and dangerous as hate speech and mass spam are, there are even bigger security concerns on the horizon.
“Some applications are safety-critical, like self-driving cars,” Oprea says. “If those models make incorrect predictions, they can’t be used.”
So what can be done? The team prepared the report, which they plan to update annually, for a few audiences — policymakers, AI developers, and academics who can use the report’s taxonomy as a foundation or context for their own work. All of these groups, Oprea says, have work to do to ensure that AI models align to human values, preserve privacy, and operate in the best interest of users. But she acknowledges that addressing every issue raised in the report is challenging, and that anyone hawking solutions rather than mitigations is sorely mistaken.
“There are many more attacks than mitigations, and for every mitigation we mention, there is a tradeoff or a performance overhead, including degradation of model accuracy,” Oprea cautions. “The mitigations don’t come for free and securing AI is a really challenging endeavor, but we hope that the report provides a useful starting point for understanding the attacks.”
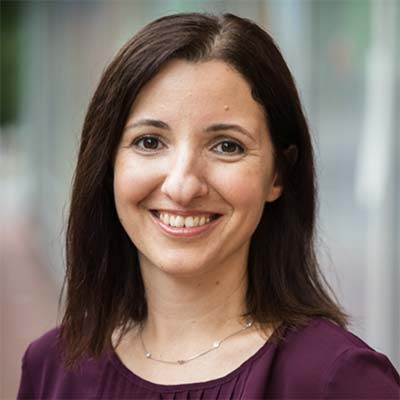
Long before 2023 ended, it had already been crowned as the year of generative AI. Spurred by the advent of models like ChatGPT that produced detailed, jarringly human replies to user prompts, experts and novices alike began musing on the technology’s potential impacts on work, education, and creativity.
But while today’s large language models (LLMs) are stunningly capable, they’re also shockingly vulnerable, says Khoury professor Alina Oprea. She’s been studying AI in a cybersecurity context for more than a decade, and recently co-authored a report that delves into these attacks on AI — how they work, how they’re classified, and how they can (and can’t) be mitigated.
“It’s really difficult to keep generative AI secure,” Oprea says. “The scale of these models and their training data will grow over time, which only makes these attacks easier. And once you start talking about generative AI that goes beyond text to images and speech, security becomes a very open question.”
The report, published by the Department of Commerce’s National Institute of Standards and Technology (NIST), is an update of the report Oprea co-authored last year with NIST’s Apostol Vassilev. That initial report dealt with more traditional predictive AI, but with generative AI exploding in popularity since then, Opera and Vassilev welcomed generative AI experts Alie Fordyce and Hyrum Anderson from Robust Intelligence to expand the project’s remit.
“Now we have academics, government, and industry working together,” Oprea noted, “which is the intended audience for the report.”
According to the report, generative AI models owe their vulnerability to a variety of factors. For one, Oprea notes, most attacks are “fairly easy to mount and require minimal knowledge of the AI system.” For another, the models’ enormous training data sets are too large for humans to monitor and validate. And the code underpinning the models isn’t automated; it relies on human moderation and is exposed to malicious human meddling.
The upshot, say the quartet of researchers, is four major types of attacks that confuse AI systems and cause them to malfunction: evasion attacks that alter the model’s inputs to change its responses, poisoning attacks that corrupt the model’s underlying algorithms or training data, privacy attacks that coax the model into revealing sensitive training data such as medical information, and abuse attacks that feed incorrect information into legitimate sources that the model learns from. By manipulating the model’s inputs, attackers can choose its outputs in advance.
“This can be used for commercial purposes, for advertisement, for generating malware spam or hate speech — things the model wouldn’t usually generate,” Oprea explains.
Without overtaxing themselves, malicious actors can control the web data an AI model trains on, introduce a backdoor, and then stealthily steer the model’s behavior from there. Given the exploding popularity of these models, such backdoors would be concerning enough on their own. But the damage doesn’t stop there.
“We now have these integrated applications that use LLMs. For example, a company builds an email agent that integrates with an LLM in the background, and it can now read your emails and send emails on your behalf,” Oprea says. “But attackers could use the same tool to send malware and spam to thousands of people. The attack surface has increased because we’re integrating LLMs into these applications.”
As destructive and dangerous as hate speech and mass spam are, there are even bigger security concerns on the horizon.
“Some applications are safety-critical, like self-driving cars,” Oprea says. “If those models make incorrect predictions, they can’t be used.”
So what can be done? The team prepared the report, which they plan to update annually, for a few audiences — policymakers, AI developers, and academics who can use the report’s taxonomy as a foundation or context for their own work. All of these groups, Oprea says, have work to do to ensure that AI models align to human values, preserve privacy, and operate in the best interest of users. But she acknowledges that addressing every issue raised in the report is challenging, and that anyone hawking solutions rather than mitigations is sorely mistaken.
“There are many more attacks than mitigations, and for every mitigation we mention, there is a tradeoff or a performance overhead, including degradation of model accuracy,” Oprea cautions. “The mitigations don’t come for free and securing AI is a really challenging endeavor, but we hope that the report provides a useful starting point for understanding the attacks.”
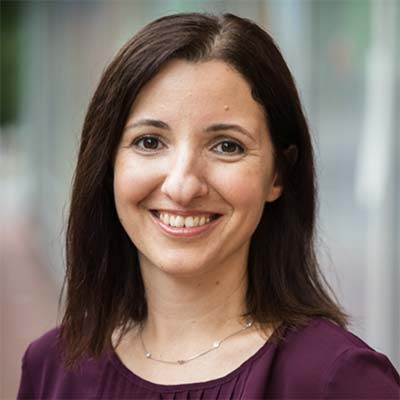
Long before 2023 ended, it had already been crowned as the year of generative AI. Spurred by the advent of models like ChatGPT that produced detailed, jarringly human replies to user prompts, experts and novices alike began musing on the technology’s potential impacts on work, education, and creativity.
But while today’s large language models (LLMs) are stunningly capable, they’re also shockingly vulnerable, says Khoury professor Alina Oprea. She’s been studying AI in a cybersecurity context for more than a decade, and recently co-authored a report that delves into these attacks on AI — how they work, how they’re classified, and how they can (and can’t) be mitigated.
“It’s really difficult to keep generative AI secure,” Oprea says. “The scale of these models and their training data will grow over time, which only makes these attacks easier. And once you start talking about generative AI that goes beyond text to images and speech, security becomes a very open question.”
The report, published by the Department of Commerce’s National Institute of Standards and Technology (NIST), is an update of the report Oprea co-authored last year with NIST’s Apostol Vassilev. That initial report dealt with more traditional predictive AI, but with generative AI exploding in popularity since then, Opera and Vassilev welcomed generative AI experts Alie Fordyce and Hyrum Anderson from Robust Intelligence to expand the project’s remit.
“Now we have academics, government, and industry working together,” Oprea noted, “which is the intended audience for the report.”
According to the report, generative AI models owe their vulnerability to a variety of factors. For one, Oprea notes, most attacks are “fairly easy to mount and require minimal knowledge of the AI system.” For another, the models’ enormous training data sets are too large for humans to monitor and validate. And the code underpinning the models isn’t automated; it relies on human moderation and is exposed to malicious human meddling.
The upshot, say the quartet of researchers, is four major types of attacks that confuse AI systems and cause them to malfunction: evasion attacks that alter the model’s inputs to change its responses, poisoning attacks that corrupt the model’s underlying algorithms or training data, privacy attacks that coax the model into revealing sensitive training data such as medical information, and abuse attacks that feed incorrect information into legitimate sources that the model learns from. By manipulating the model’s inputs, attackers can choose its outputs in advance.
“This can be used for commercial purposes, for advertisement, for generating malware spam or hate speech — things the model wouldn’t usually generate,” Oprea explains.
Without overtaxing themselves, malicious actors can control the web data an AI model trains on, introduce a backdoor, and then stealthily steer the model’s behavior from there. Given the exploding popularity of these models, such backdoors would be concerning enough on their own. But the damage doesn’t stop there.
“We now have these integrated applications that use LLMs. For example, a company builds an email agent that integrates with an LLM in the background, and it can now read your emails and send emails on your behalf,” Oprea says. “But attackers could use the same tool to send malware and spam to thousands of people. The attack surface has increased because we’re integrating LLMs into these applications.”
As destructive and dangerous as hate speech and mass spam are, there are even bigger security concerns on the horizon.
“Some applications are safety-critical, like self-driving cars,” Oprea says. “If those models make incorrect predictions, they can’t be used.”
So what can be done? The team prepared the report, which they plan to update annually, for a few audiences — policymakers, AI developers, and academics who can use the report’s taxonomy as a foundation or context for their own work. All of these groups, Oprea says, have work to do to ensure that AI models align to human values, preserve privacy, and operate in the best interest of users. But she acknowledges that addressing every issue raised in the report is challenging, and that anyone hawking solutions rather than mitigations is sorely mistaken.
“There are many more attacks than mitigations, and for every mitigation we mention, there is a tradeoff or a performance overhead, including degradation of model accuracy,” Oprea cautions. “The mitigations don’t come for free and securing AI is a really challenging endeavor, but we hope that the report provides a useful starting point for understanding the attacks.”
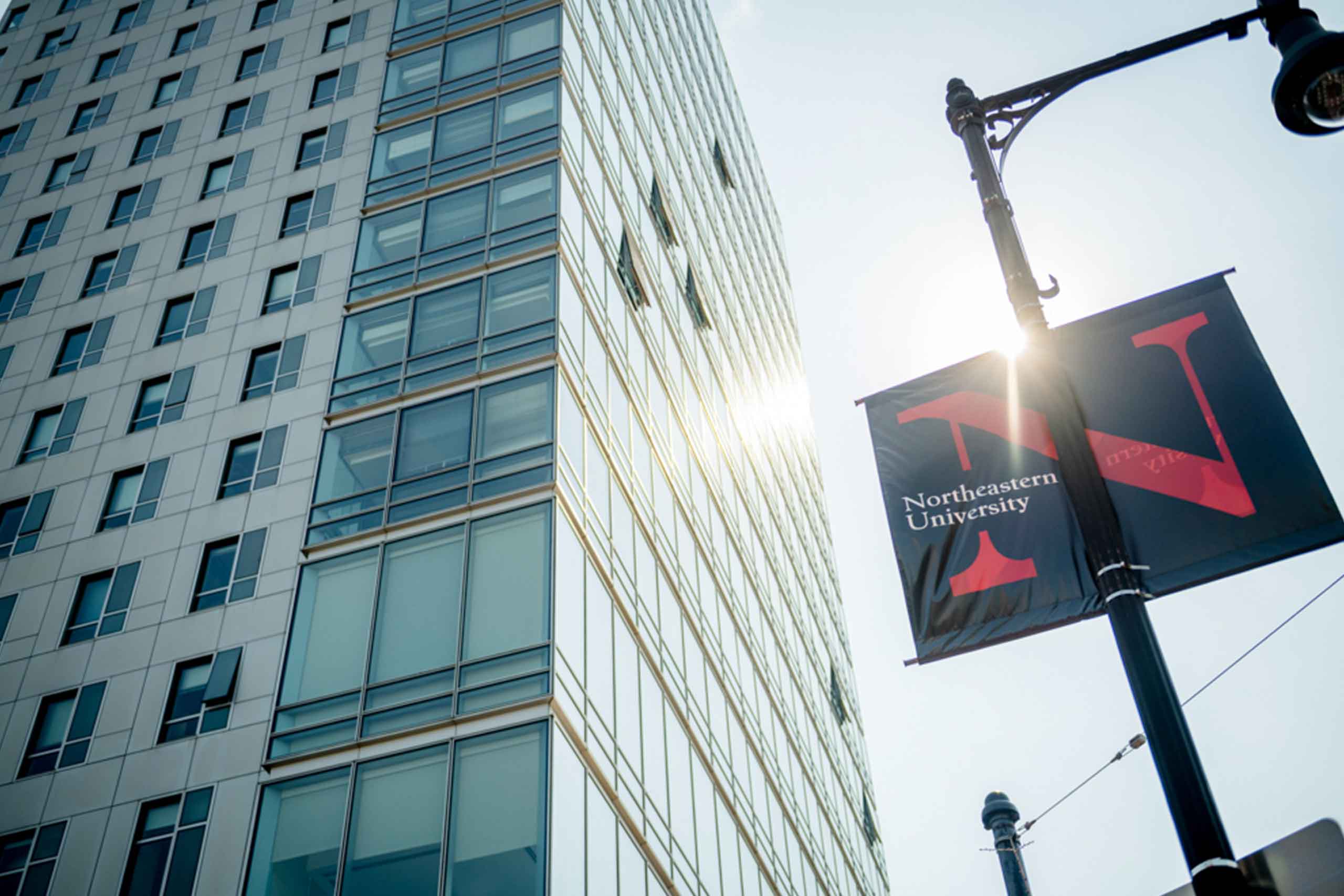
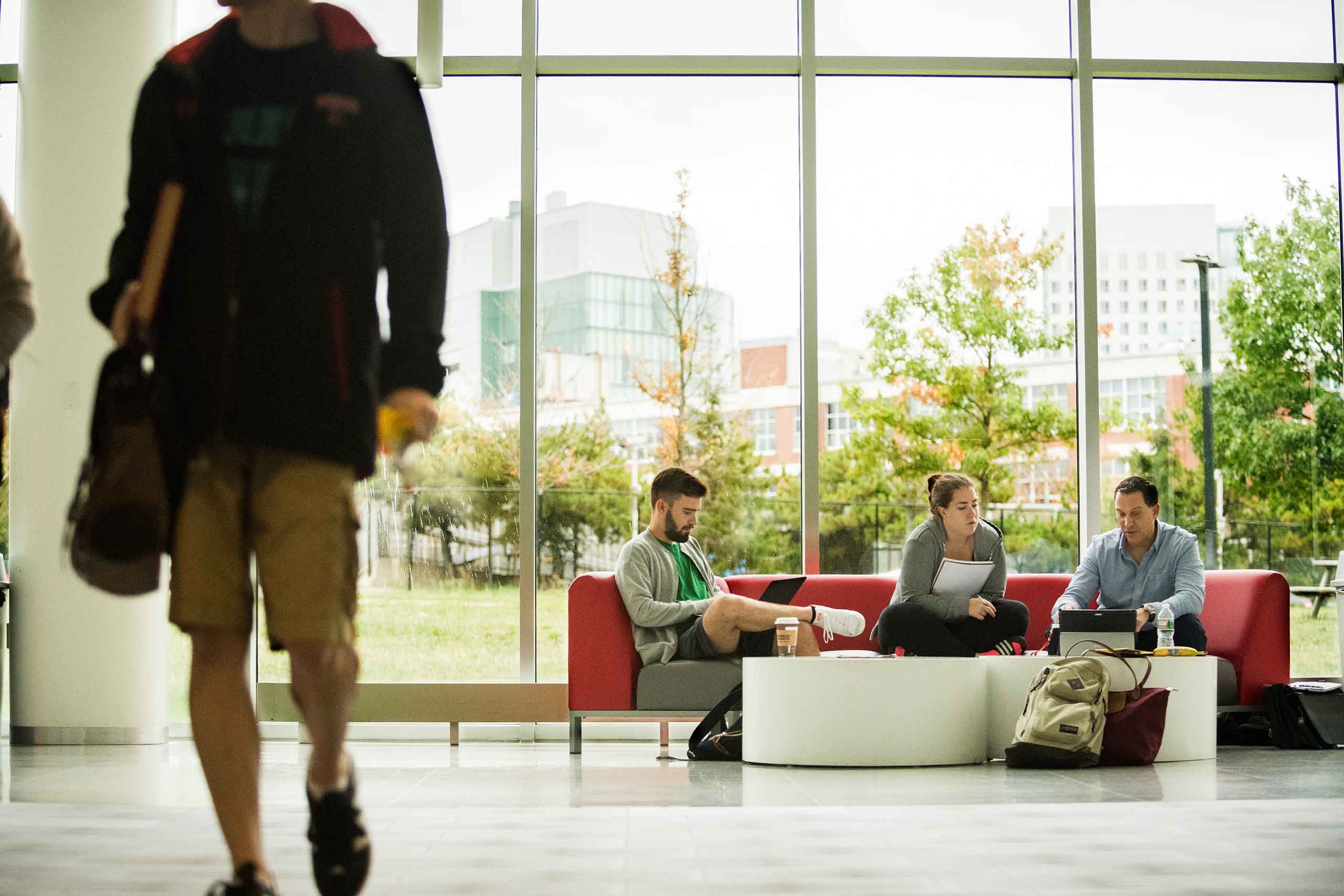
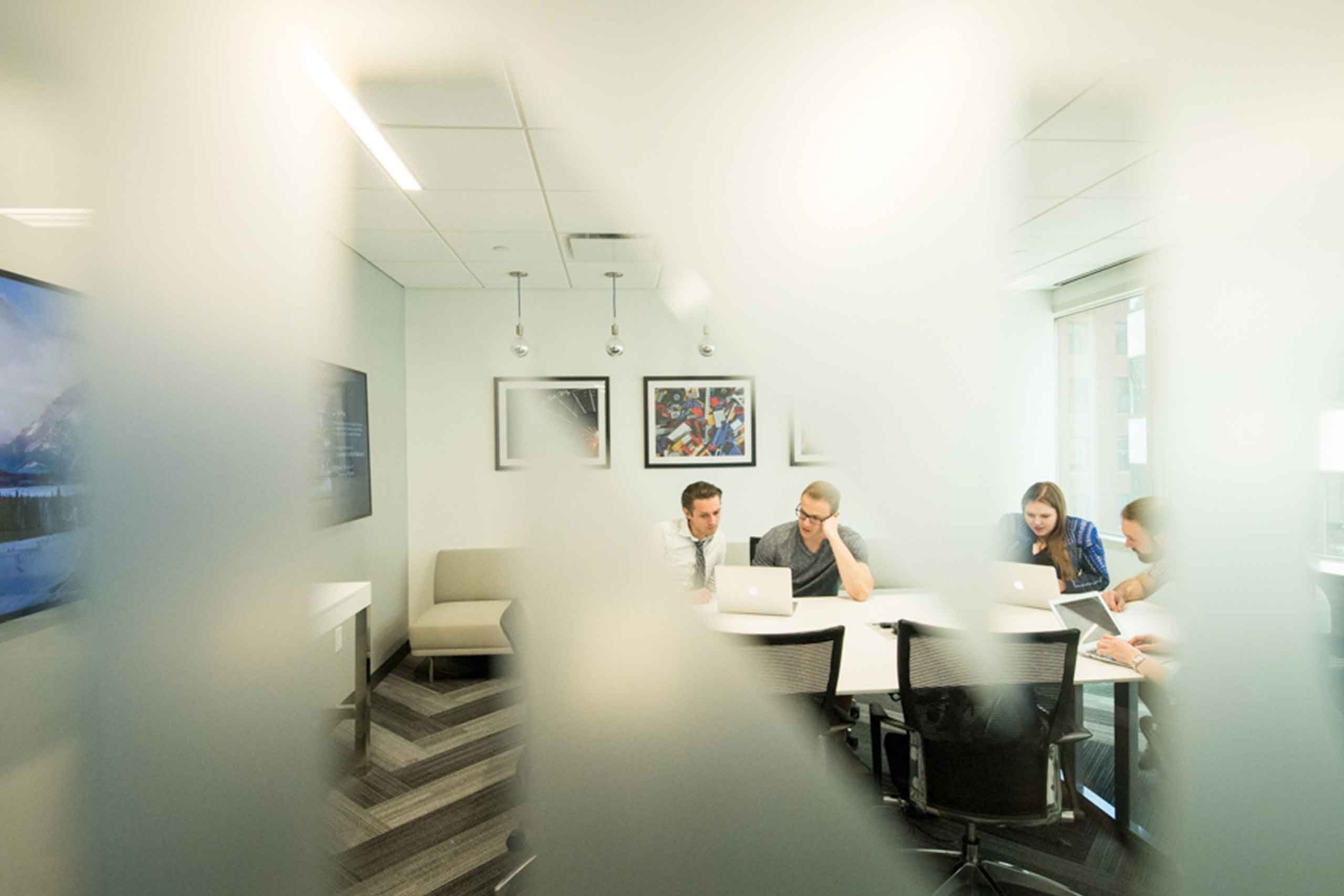
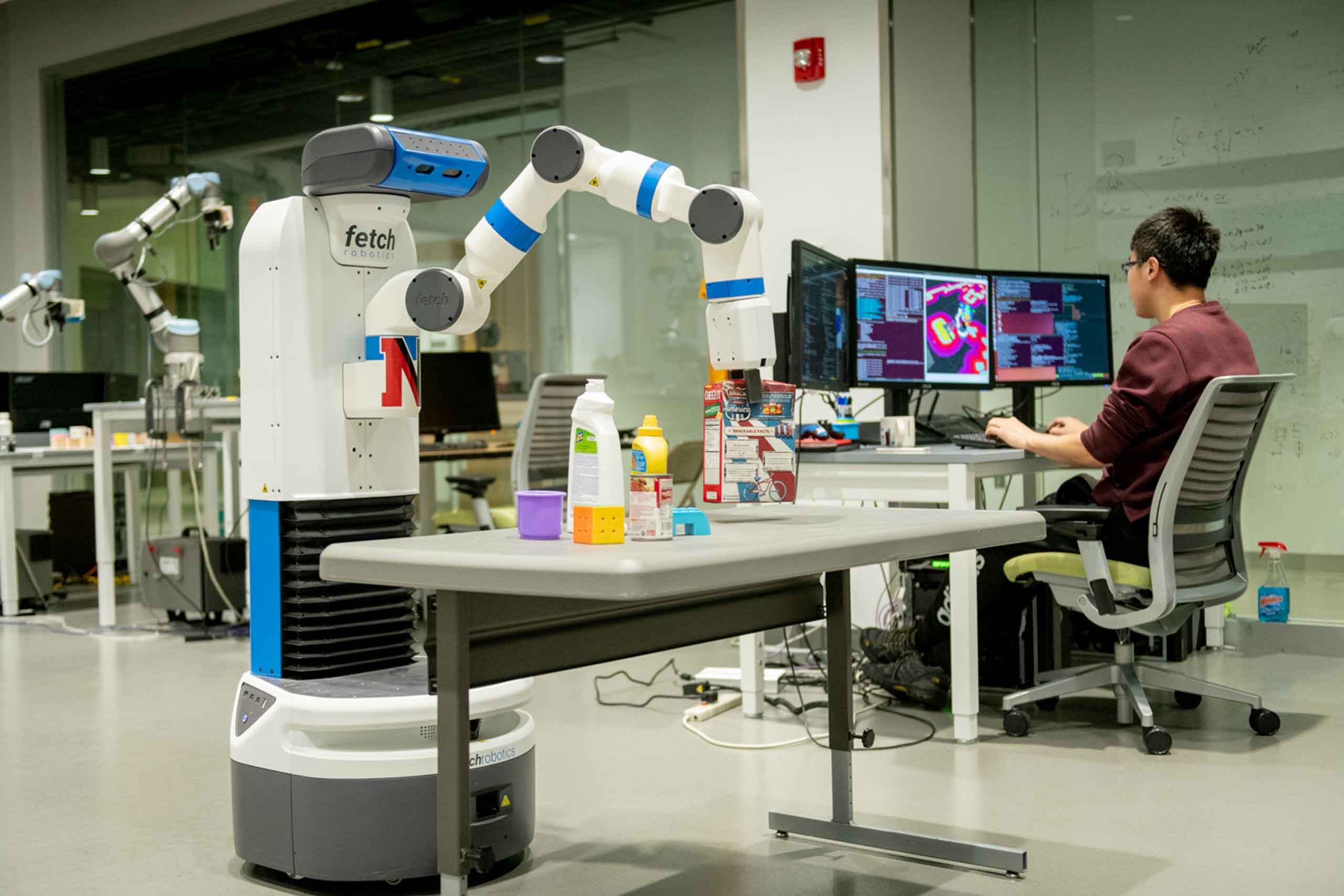
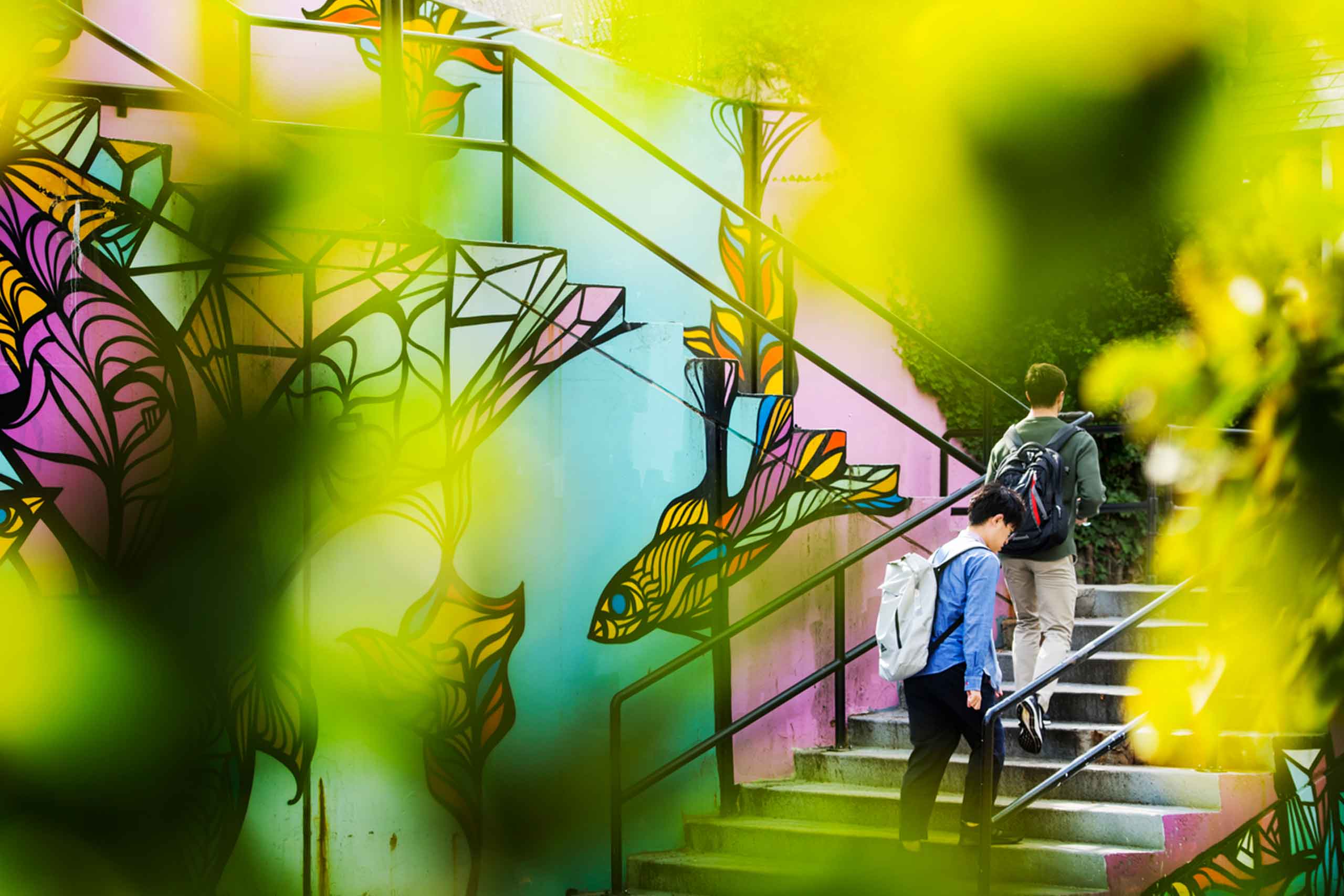
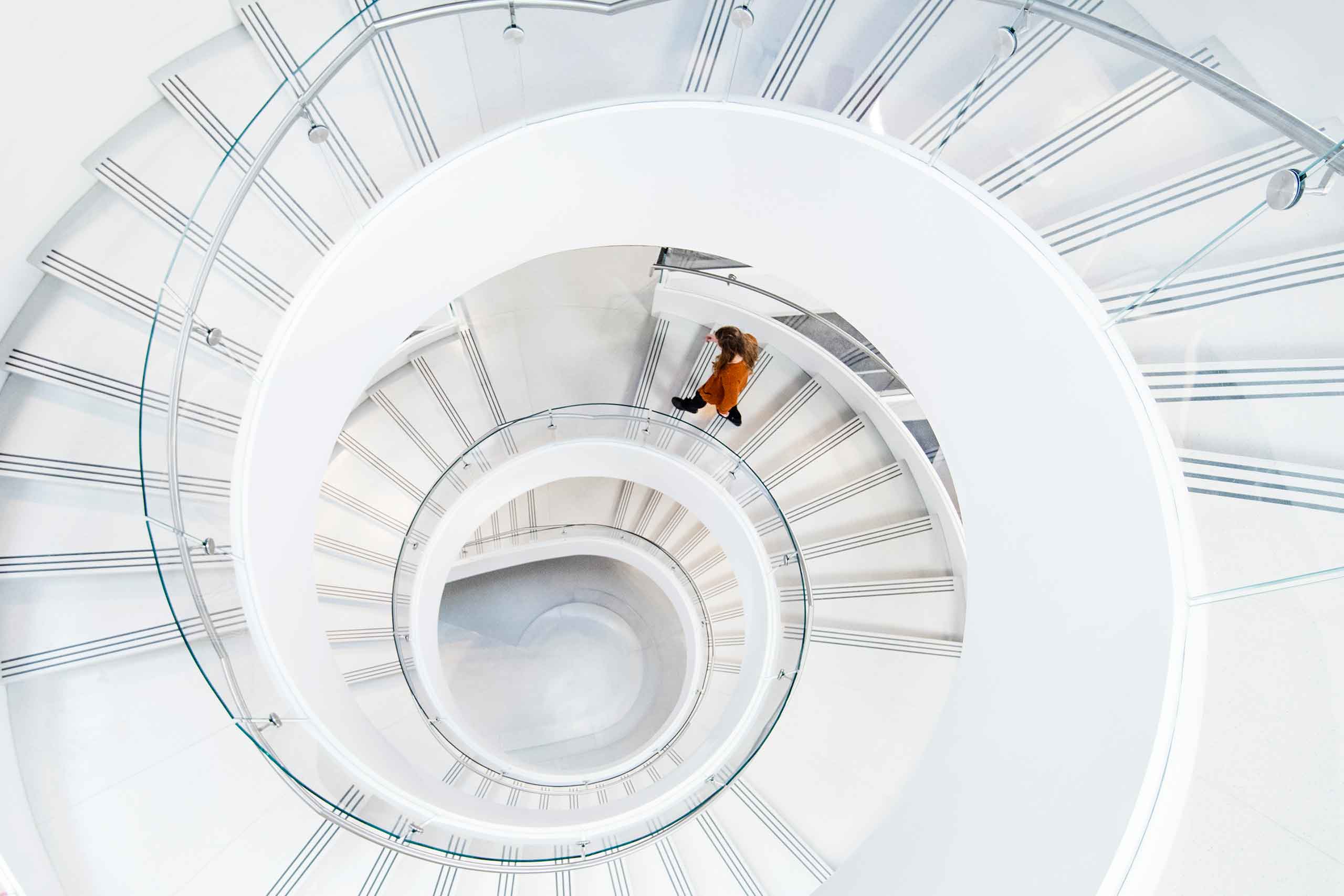
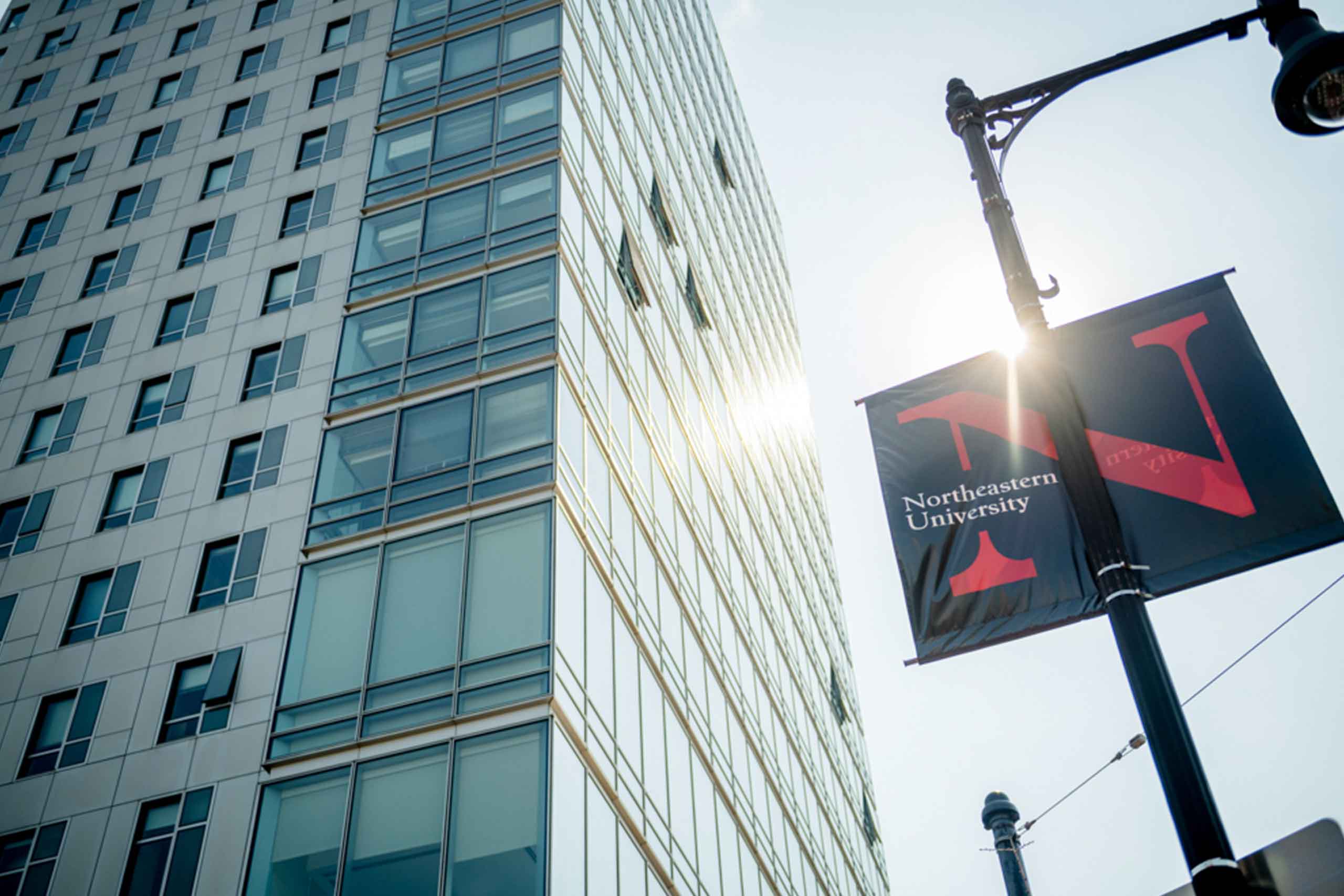
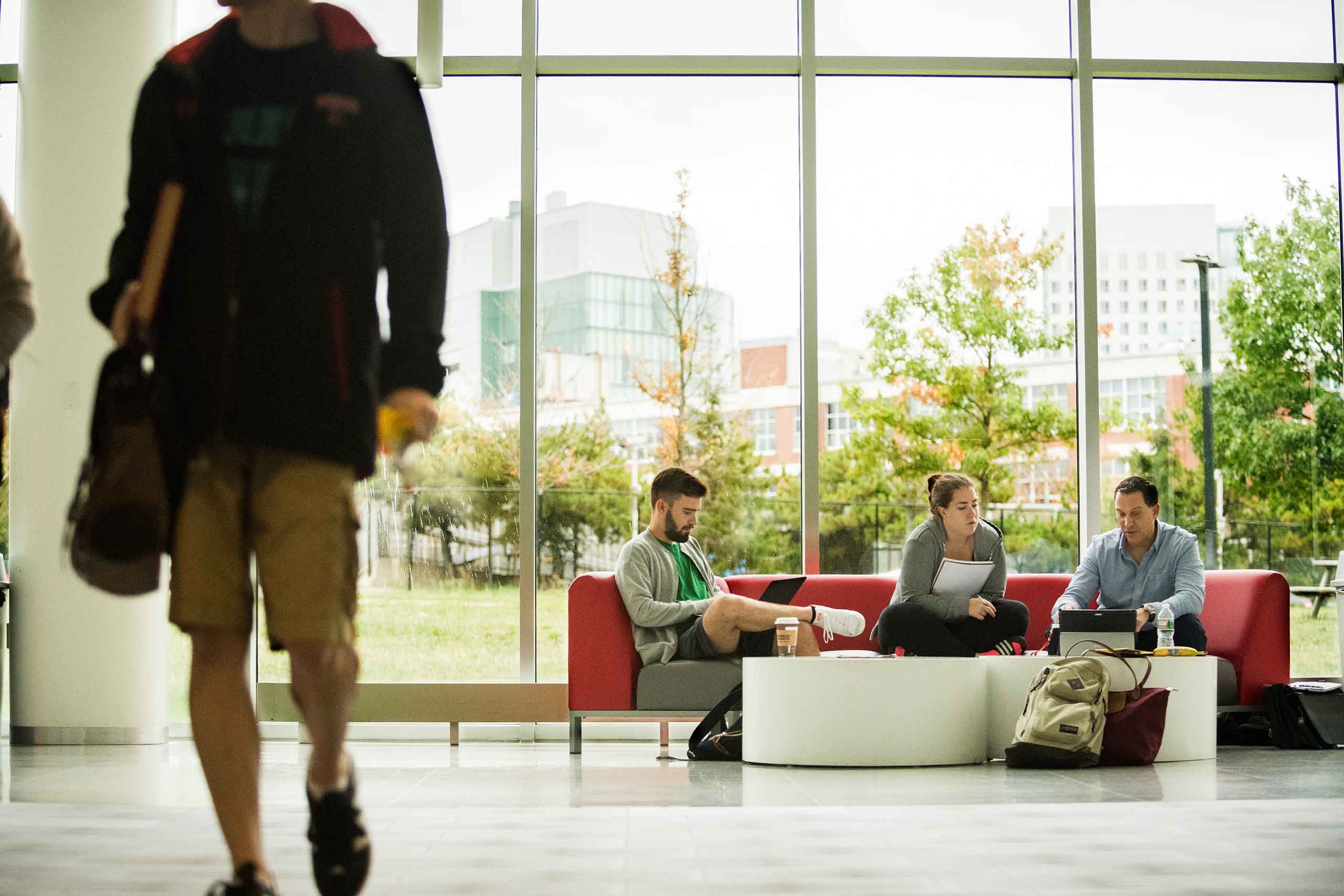
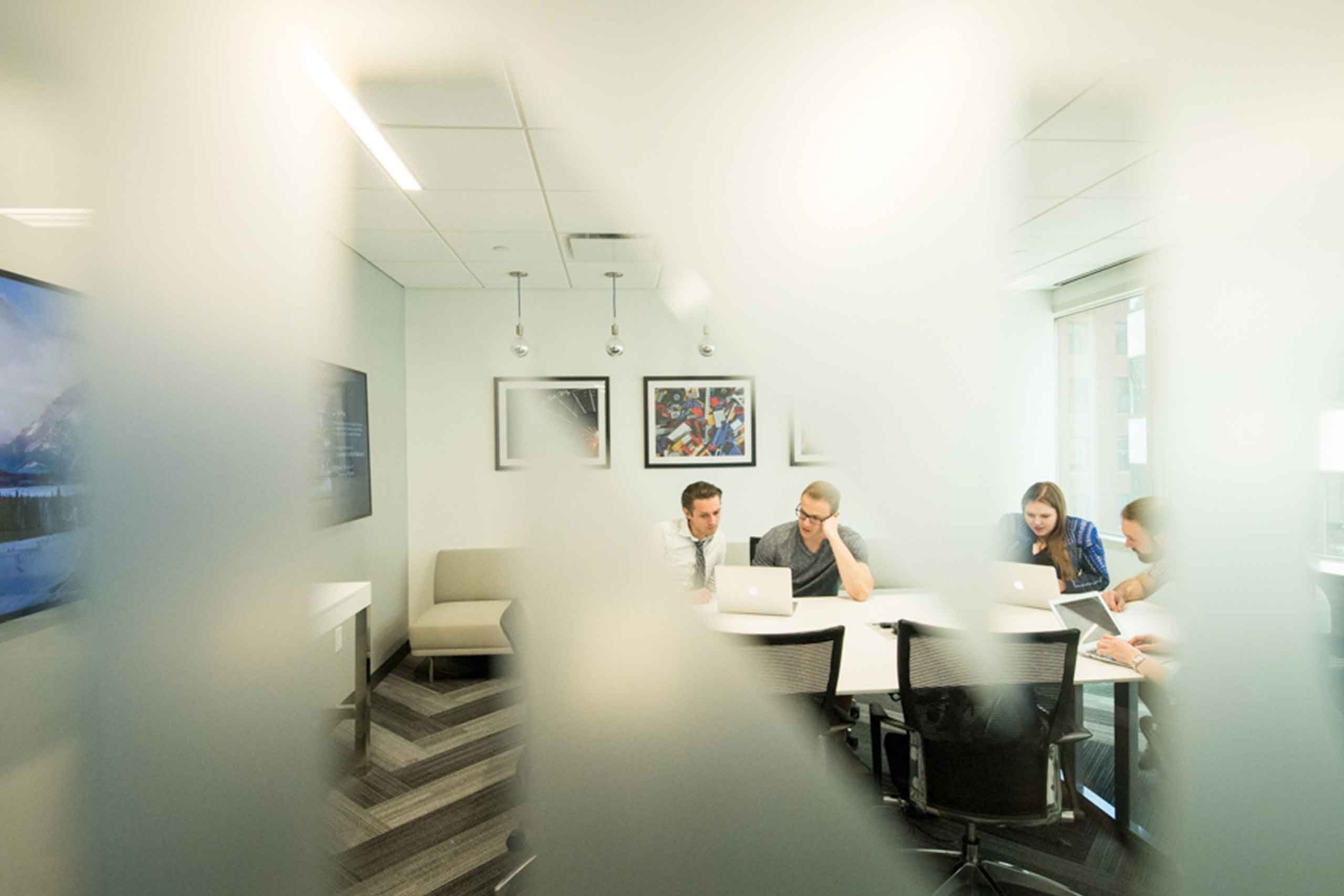
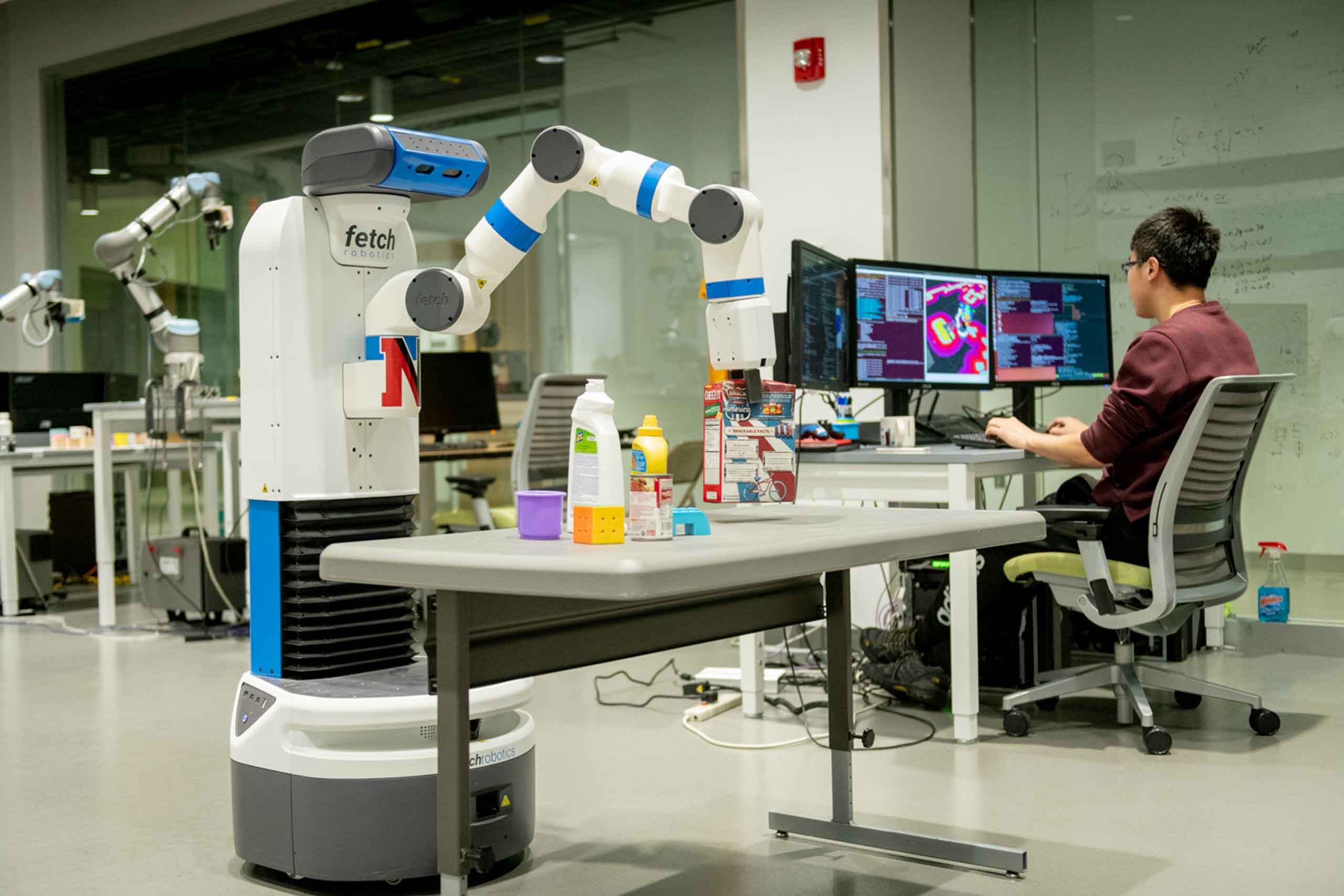
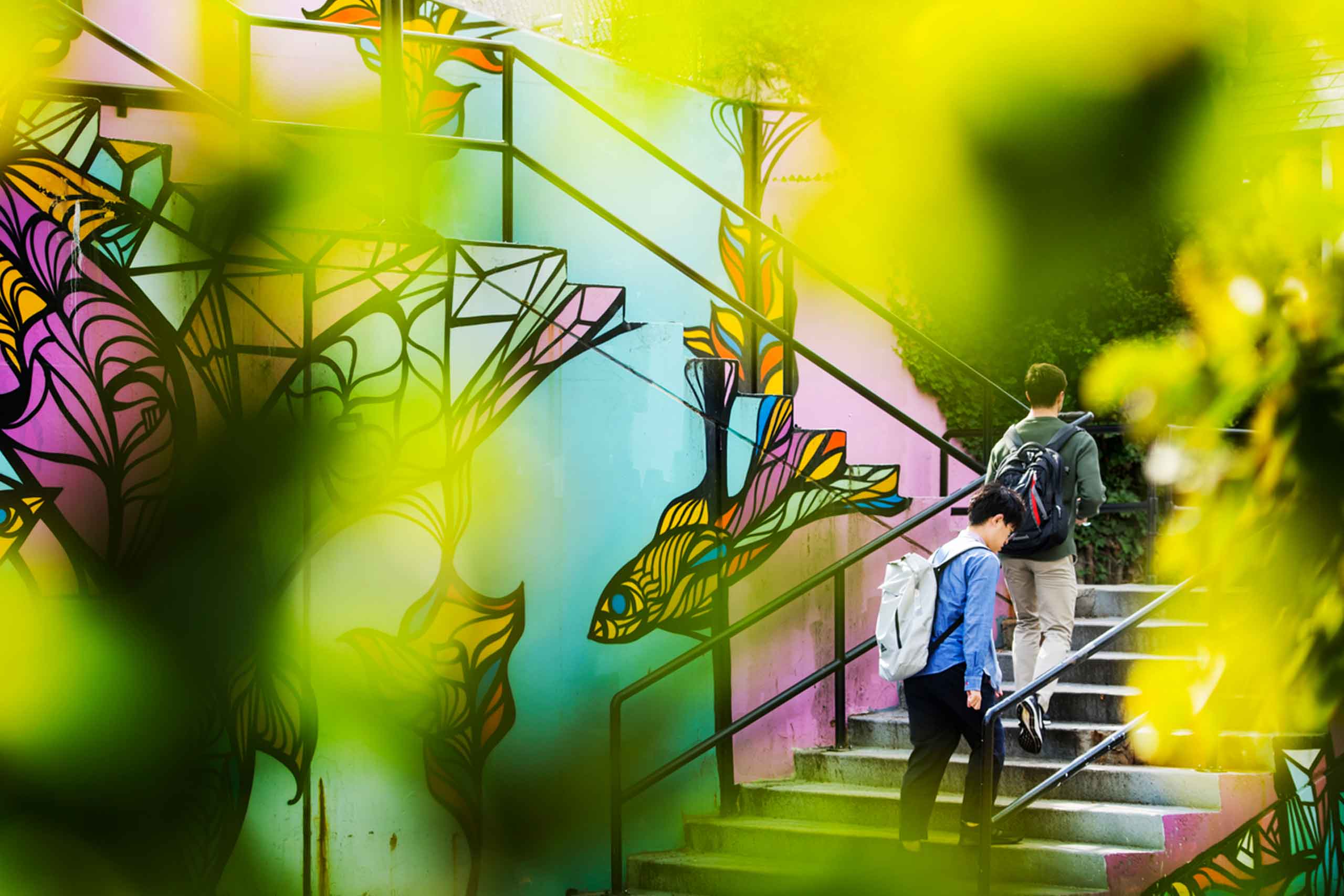
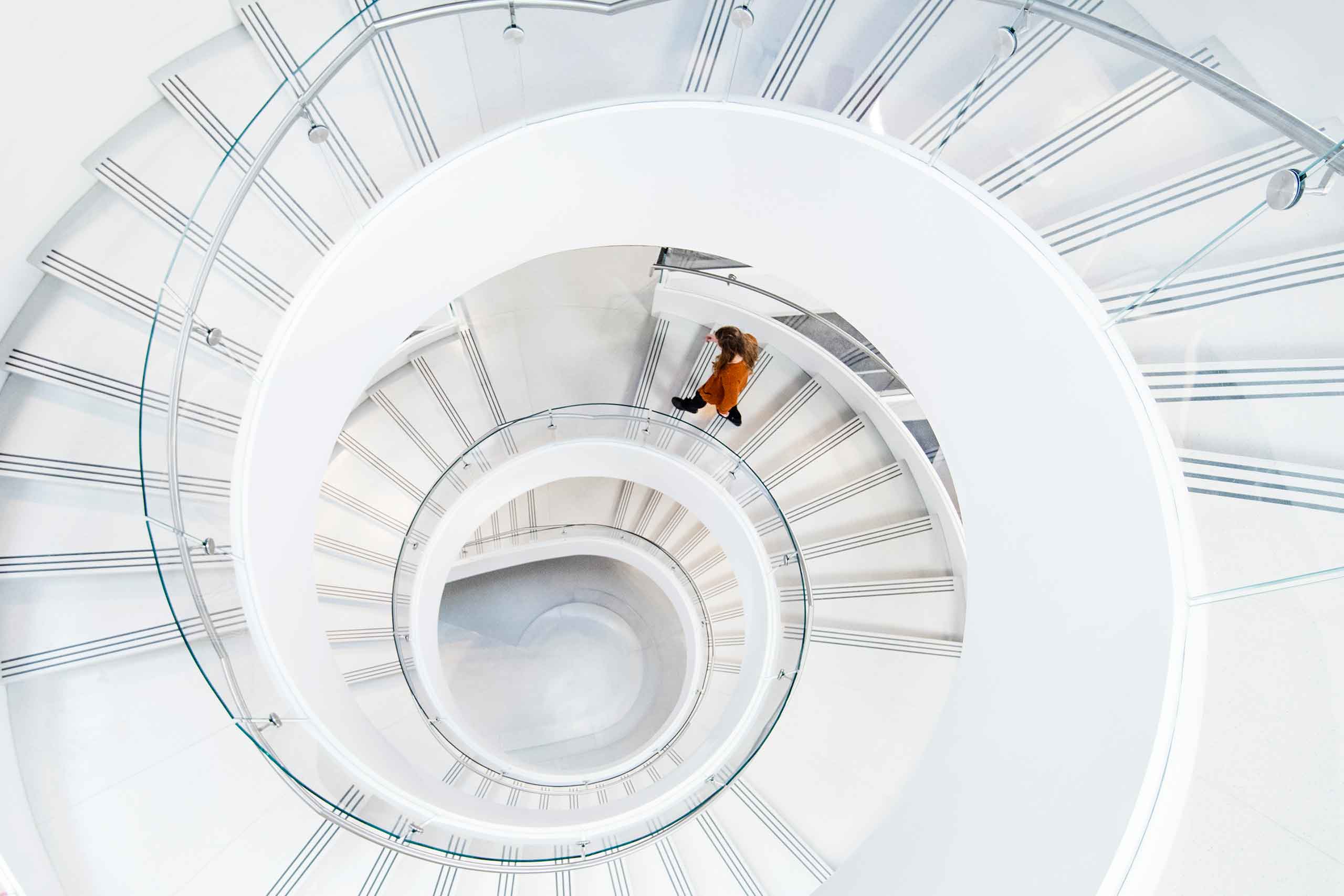