In clinics, classrooms, and conferences, Predrag Radivojac elevates computational genomics
Author: Milton Posner
Date: 03.06.23
For Predrag Radivojac, multiple multi-year efforts came to fruition in 2022 and solidified him as a leader in his field.
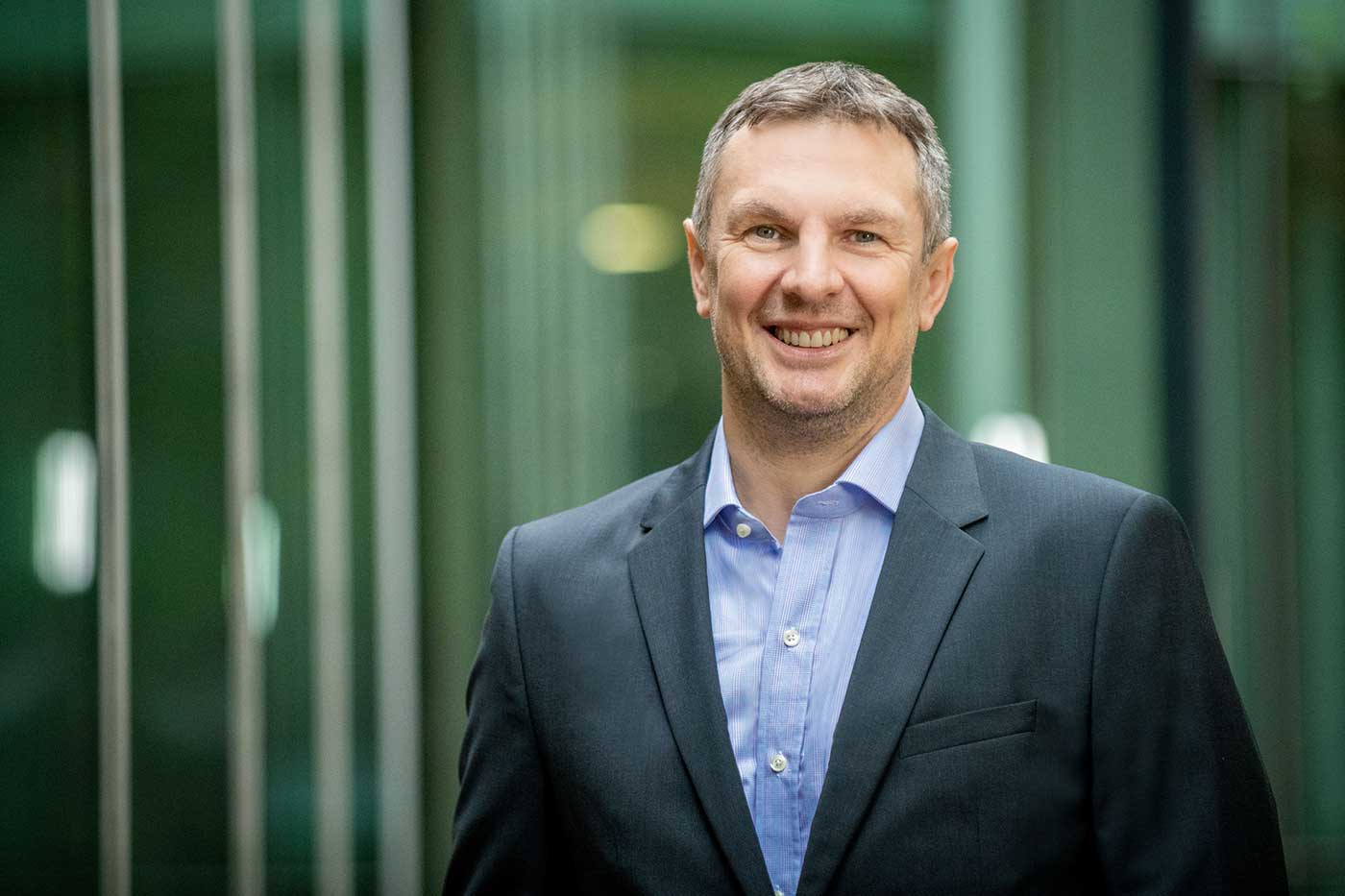
He published two major research projects — one on reducing the risk of gestational diabetes, another on incorporating algorithms into clinical practice. Participants at his genome interpretation conference diagnosed genetic diseases for patients left in limbo by conventional medical establishments. And after nearly a decade on the board of the International Society for Computational Biology, he was elected to captain the group into the field’s exciting future.
“Genomes are so big, and genomic variation is so extensive, that nobody can experimentally test all of it,” says Radivojac, associate dean of research at Khoury College. “It has to be driven by predictors. There are many machine learning challenges to solve these problems, and I would like to help solve those challenges.”
And with 2022 in the books, Radivojac is well on his way to doing so.
Frenetic genetics
An ultimate goal of any machine learning method is to translate it into practice to solve a societal problem. For Radivojac, this means leveraging AI to better understand how human genetic variants and mutations affect diseases and disorders. Understanding genetic variation helps physicians to diagnose patients early and correctly, customize treatments, and improve outcomes for conditions ranging from cancer to autism.
An example: Radivojac and his collaborators analyzed the genomes of over 3,500 women to measure their risk for gestational diabetes (GD). Their paper, published in August, found that about 30 minutes of moderate physical activity per day early in a pregnancy can curtail GD risk, including among women genetically predisposed to diabetes.
“It has an impact,” Radivojac says of the effort, which counted several of his students and postdocs among its lead authors. “That’s what we care about: that our research translates into clinical practice and advice to patients.”
The same ethos underpinned another of Radivojac’s former-student-studded collaborations, published in December. Previous academic and industry groups crafted guidelines for when an algorithmic output could help classify a genomic variant as “disease-causing,” and thus when clinicians could use the output as a basis for molecular diagnosis, treatment, and therapy.
READ: Millicent Li bridges the gap between theoretical AI and medical practice
Under previous guidelines, computers could provide only “supporting” evidence, the lowest tier of evidence used in establishing pathogenic status. Radivojac co-led the team that found computers can provide “strong” evidence, the second-highest tier. They successfully defended their findings to ClinGen, an NIH-funded consortium that aims to authoritatively define the clinical relevance of genes and variants.
“Often, algorithms were undervalued in clinical practice, so we’ve developed new guidelines,” Radivojac says. “I’m proud of this work because more people will be diagnosed. The evidence says that more variants can be called pathogenic, more diagnoses can be provided, and fewer of them will be false positives.
“To me, this paper is a game-changer.”
Conference and camp
In May, several hundred people participated in the sixth NIH-funded Critical Assessment of Genome Interpretation (CAGI), which was followed by a 100-person conference which Radivojac co-chaired. CAGI works to assess the quality of genome interpretation algorithms, use genomic sequences to predict a person’s risk for common diseases, and find genomic explanations for rare genetic disorders. To discover which variant is responsible for the characteristics they’re seeing, participants examine everything from standalone protein mutations to entire genomes.
The conference exists in part because genomics lacks an authoritative body to evaluate the accuracy of algorithmic diagnostic methods and disseminate the results. For instance, physicians may lack the time or mathematical foundation to understand how an algorithm works, but CAGI works to ensure they can still understand how accurate it is. Additionally, an increasing number of companies are selling computational diagnostic services to patients, and their performance needs independent evaluation.
“We assess the quality of these methods through a series of 14 challenges,” Radivojac says. “For clinicians, the conference provides information about the quality and applications of the methods. For us computational researchers, it shows what works, which ideas win, what the bottlenecks in the field are, and what is relevant to work on.”
May’s conference had plenty of significance for individuals with rare genetic diseases and disorders too. These patients often seek etiological diagnoses — explanations not of what they have, but of why they have it — and they may wait years for results. Radivojac believes computational tools can reduce this wait by telling us which genomic variants are causing their disorders.
Working with 30 genomic trios previously evaluated by the Broad Institute of MIT and Harvard, the CAGI challenge participants correctly identified disease-causing variants on most of the cases that Broad had solved, and solved two new ones by using different methods than Broad did. Because of that, two families received etiological diagnoses to help them to pursue the right therapies for their children and gauge the likelihood of the same condition happening in another child.
CAGI followed this up with its first-ever virtual summer bootcamp, attended by 17 high-school and undergraduate students from five continents. It blended lectures on genome interpretation and biology with hands-on training in Python programming and machine learning, and even sent two students — UTEP’s Luis Cedillo and Tuskegee University’s Kaiya Jones — to the Annual Biomedical Research Conference for Minoritized Scientists. Like the class’s geographic spread, this trip reflected the students’ push for greater diversity in — and deeper knowledge of — the field.
“They’re pushing us to teach them, and when you see how competitive those kids are, it’s unbelievable — it’s beautiful,” Radivojac says. “They just keep coming.”
Mighty society
This summer, Radivojac was elected president of the 3,000-member International Society for Computational Biology (ISCB), the preeminent body dedicated to understanding life at the molecular level through computation. He’ll begin his three-year term in January 2024, with plans to expand the society and bolster ties across borders.
Radivojac was instrumental in establishing Bioinformatics Advances, the ISCB’s open-access, peer-reviewed journal now in its second year. It’s important to showcase computational biology excellence, he says, because the field’s researchers sometimes find support lacking in their own departments. Computer science colleagues may write them off for not working with sufficiently “hardcore” CS systems and architectures, while biologists may view CS as “too technical.”
“I understand the challenges our researchers face, and how our students go to find careers,” Radivojac says. “The Society has a voice, and it’s important to keep that voice going to advocate for necessary changes.”
The ISCB lobbies for increased support for computational biology in research grants and promotes the role of computation in solving clinical problems. It also promotes standards for computational biology curricula and career credentials, and recognizes excellence in the field through its awards and fellow selection.
But for all the progress the field’s researchers have made, large hurdles remain. Some minority groups are reluctant to donate their genomes to research projects given historical injustices, and are underrepresented in research populations as a consequence. Some countries refuse to allow data sharing across borders, citing concerns over data protection or human rights records. And many physicians wary of false positives are reluctant to make AI a pillar of disease diagnosis, reasoning that they can’t just tell patients “the algorithm said so.”
But the field’s potential defies reckoning. Polygenic risk scores could gauge your risk for complex diseases like cancer and diabetes. Computationally enhanced newborn screening could identify autism early, increasing the effectiveness of targeted behavioral therapy. Genomic diagnoses could help patients preemptively adjust their lifestyle to earn their best chance at long-term health. Radivojac wants to be in the middle of it all, and to do it the right way.
“In the end, a computer scientist is a computer scientist, and I get satisfaction from important technical solutions,” Radivojac says. “But we need time to do them right. That’s where ethical challenges come in; you can do damage — impacting specific populations, diagnosing incorrectly, or missing diagnoses — if you rush things, aren’t careful, or don’t know the material.
“I’d like my research to continue contributing to interpretation of genomic variants. It’s an extremely powerful field that makes a difference in the clinic.”
Subscribe to Khoury News
The Khoury Network: Be in the know
Subscribe now to our monthly newsletter for the latest stories and achievements of our students and faculty