Deepfakes, speech recognition, and more at the Fall 2023 Khoury Research Apprenticeship Showcase
Author: Matty Wasserman
Date: 12.19.23
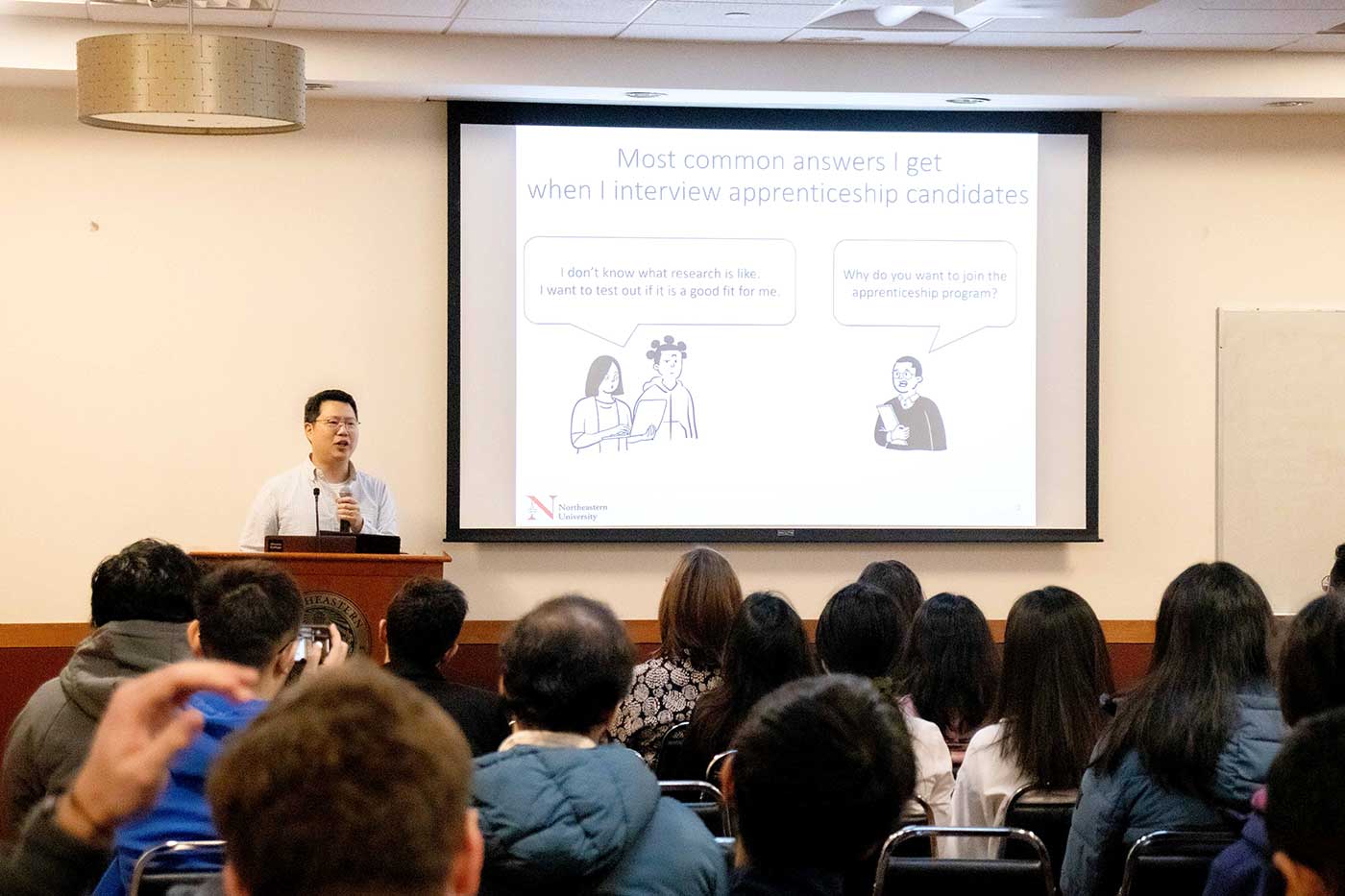
Since its inception in 2019, Khoury Research Apprenticeships have provided unique opportunities for master’s students to work on semester-long research projects of their choosing under the guidance of a faculty mentor. On November 28, this semester’s Boston cohort presented their work to faculty, staff, and fellow students at the Curry Student Center.
“Through education, you will learn enough knowledge to work in industry. But to really reach that edge [of adding knowledge to the world], you will need to make extra effort beyond what is simply taught to you,” said Ji Yong Shin, the event’s keynote speaker and an assistant professor at Khoury College. “But getting there yourself is very difficult. That’s why programs like this apprenticeship will help guide you to reach that edge.”
Click one of the names below to read about their project, or learn more about the program here.
Lauryn Fluellen
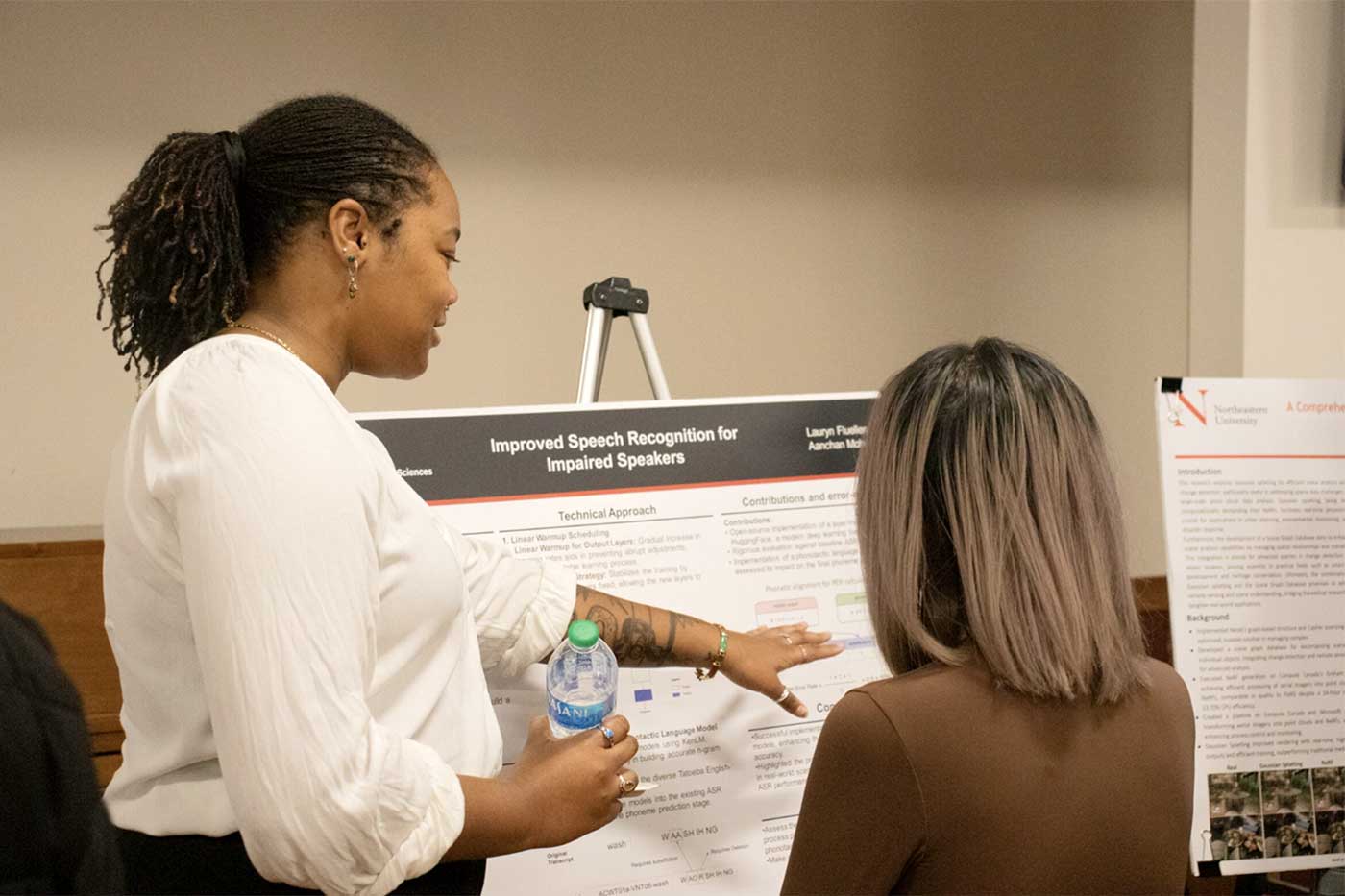
Lauryn Fluellen’s passion for natural language processing and speech recognition originated during her undergraduate years at the University of Rochester, where she majored in linguistics and cognitive science and worked as a research assistant in language acquisition and development labs on campus. As a master’s student at Khoury College, she’s continued to delve deep into the area — first through Northeastern’s Ethics in Computer Science Research Award, where she explored the word error rate of speech recognition software for users from marginalized groups, and now through a project with Aanchan Mohan, an assistant professor at Northeastern’s Vancouver campus.
Fluellen’s research examined anomic aphasia, a language disorder characterized by trouble naming objects and finding the right words to say — a condition often seen in stroke patients. Using a small data set, Fluellen aimed to improve the performance of existing automated speech recognition (ASR) systems for these users.
“This was a great way to combine my past research and interests,” Fluellen said. “[Mohan] is interested in increasing accessibility and creating accessible technology, and his focus is on the improvements of the ASR and voice conversion models. So it was a great fit.”
Debankita Basu
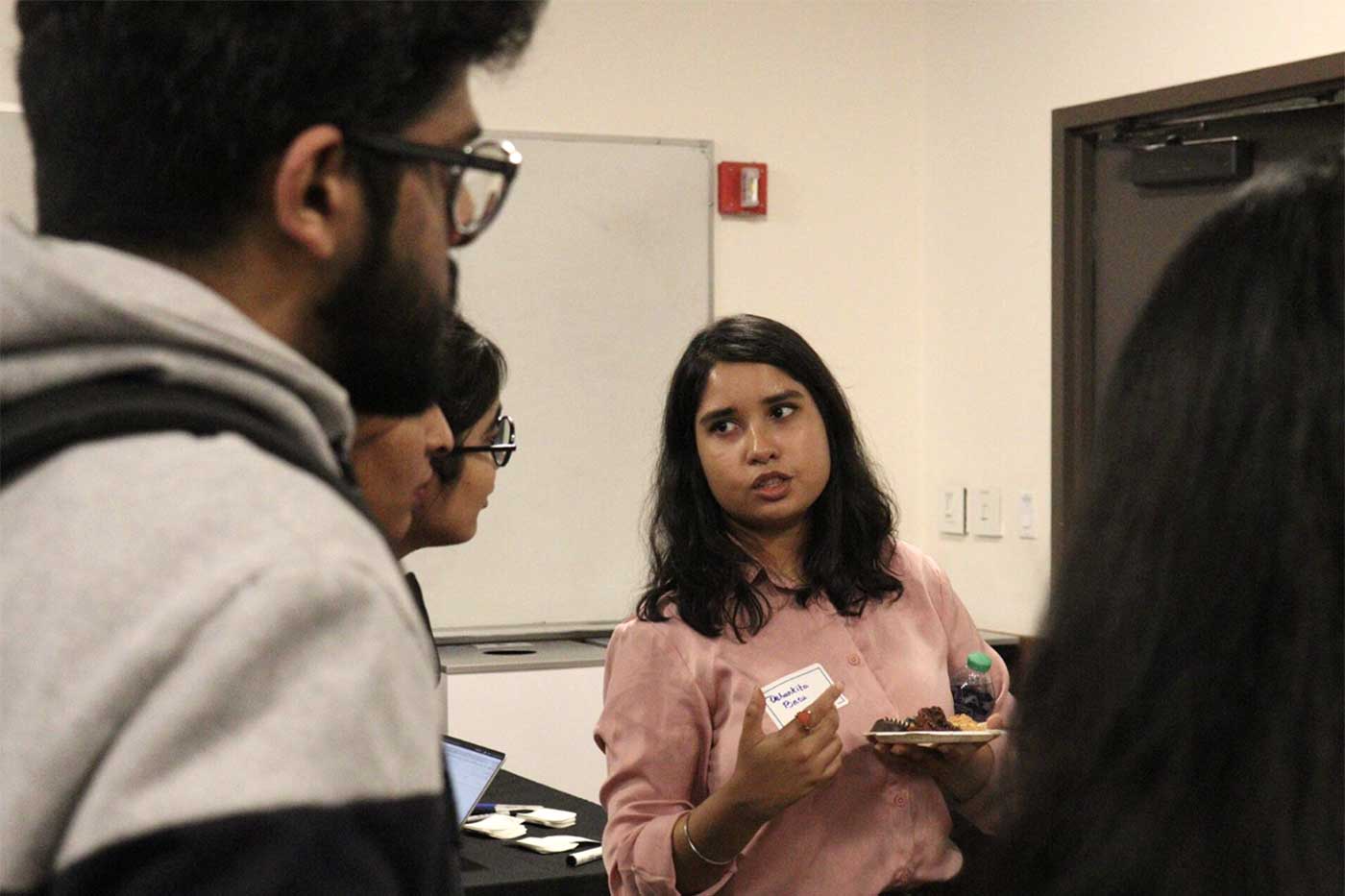
The first time Debankita Basu read about deepfake images was in 2020, and while the technology was relatively novel at the time, the subject has fascinated her ever since. As AI-generated fake images have become increasingly difficult to distinguish from real-life images, the need for reliable detection programs has also skyrocketed.
“I always wanted to work on a project where I could leverage my deep learning knowledge and build something that could be used to mitigate unethical practices in technology, helping the community while doing so,” she said.
In her study, Basu compared two well-known image-decoding models: the traditionally used Convolutional Neural Network (CNN), which uses filters to detect patterns or features in input data, and the Vision Transformer (ViT), a relatively new technology that makes use of revolutionary transformer architectures. Her study tested how effectively each model determined if the image was real or fake, and found that the CNN model was 70% accurate while the ViT model was 80% accurate, showing the promise of the new technology.
Shagun Saboo
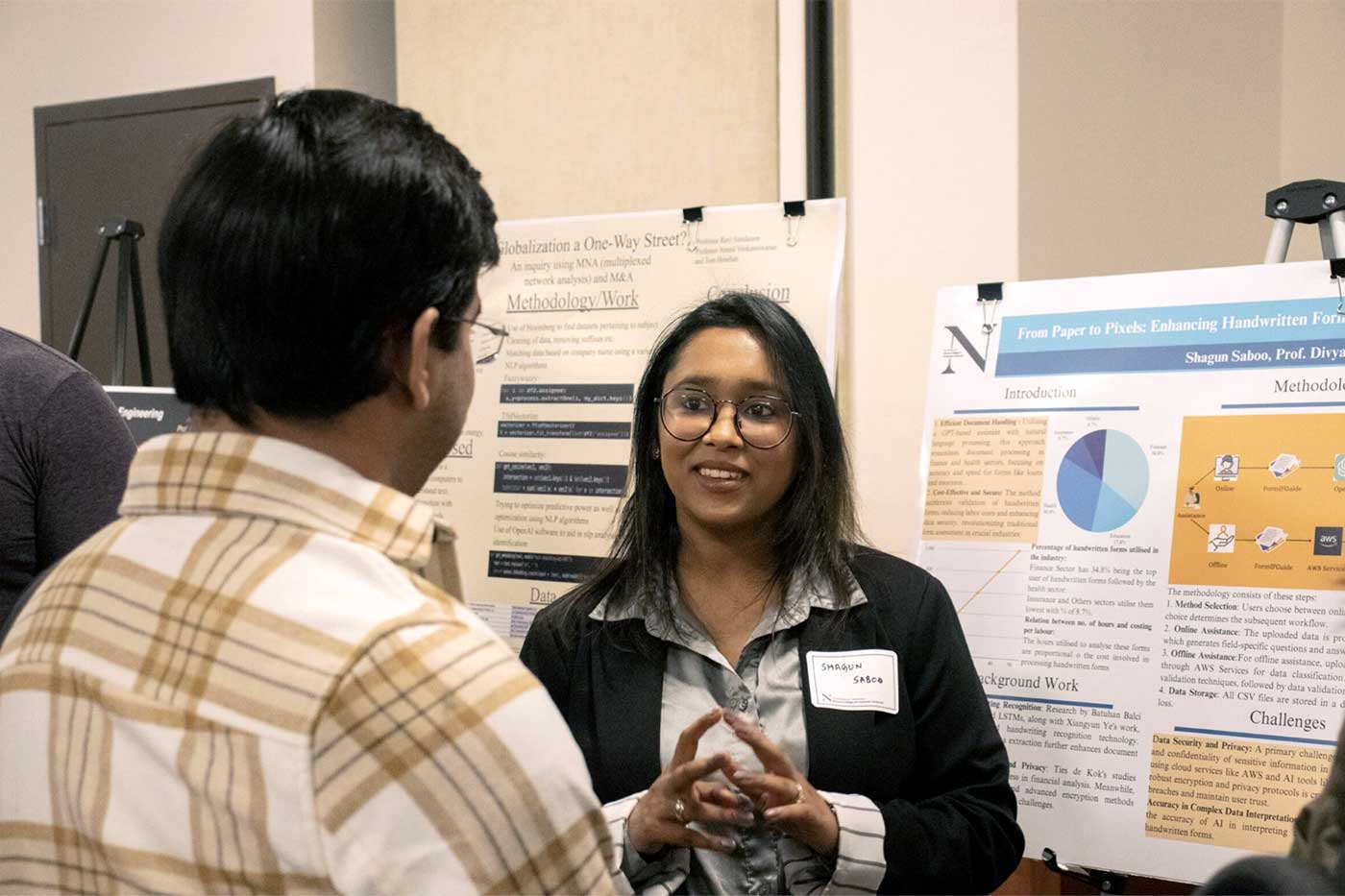
Even in today’s technology-driven world, companies still process huge volumes of handwritten forms. According to master’s student Shagun Saboo, in the finance sector, 35% of forms are written on paper, and in the health sector, it’s 30%. These forms present a challenge for companies given the difficulty of processing handwriting and the varying reliability of technologies.
That’s where Saboo’s work comes in. With the help of Assistant Professor Divya Chaudhary, Saboo aimed to streamline document processing in the finance and health sectors by using generative AI. Her work specifically focused on improving the accuracy and speed of form validation software, which, if reliable, can boost a company’s efficiency and reduce the need for manual labor to process forms.
“Firstly, I did a deep dive into understanding the problem and making it more concrete,” Saboo said. “Then, I interacted with the banks and health care centers to understand what they needed. The next step was researching the problems — extracting the handwritten data from the key forms, and then securing them. Then, we created validation techniques to know whether these forms were correctly filled out.”
Subhankar Shah
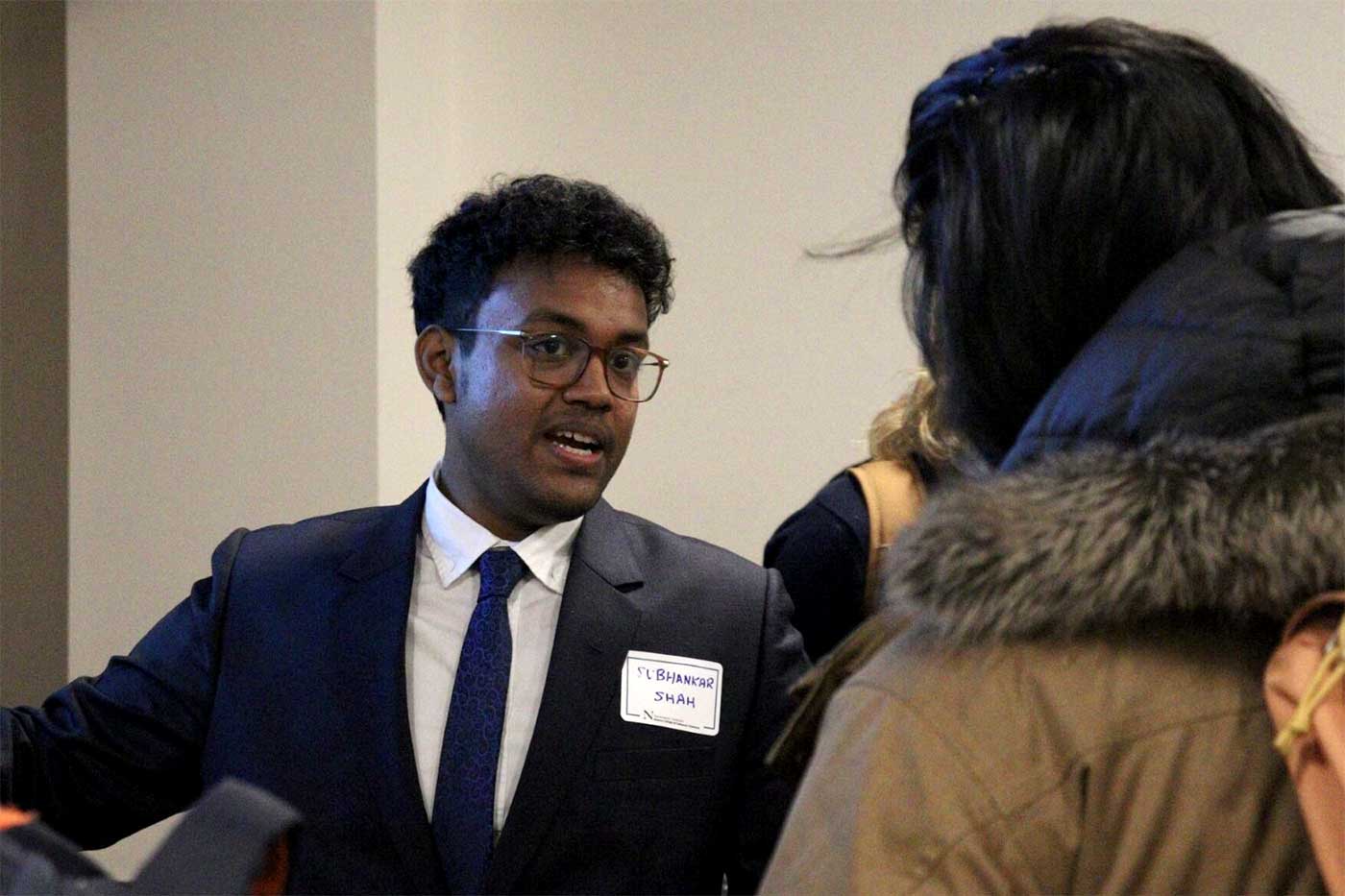
Back in 2005, Mario Nascimento conducted a study on how to efficiently search a high-dimensional data set. Now, in the era of ChatGPT when models boast added complexity and dimensionality, master’s student Subhankar Shah teamed with Nascimento to build on that past work and find more efficient ways for large language models to search for data using vector databases.
“When you give a prompt to ChatGPT, it searches for something in a huge data set,” Shah said. “We wanted to look at how large language models query these trillions of data efficiently, then come up with an approach where the searching of the data set is efficient and quick.”
To sort the large datasets containing many dimensions, Shah and Nascimento applied a novel technique called M-Grid, which functioned well across several parameters and could perform nearest neighbor queries more than 20 times faster than a sequential scan — a typical method for querying large swaths of data. The technique clusters data by pinpointing specific parts of the search as the central query point, then narrows the scope of the search until it optimizes the results.
“We will only query the nearest clusters, and not every cluster. That’s how we are saving time,” Shah said.
Keerthana Velilani
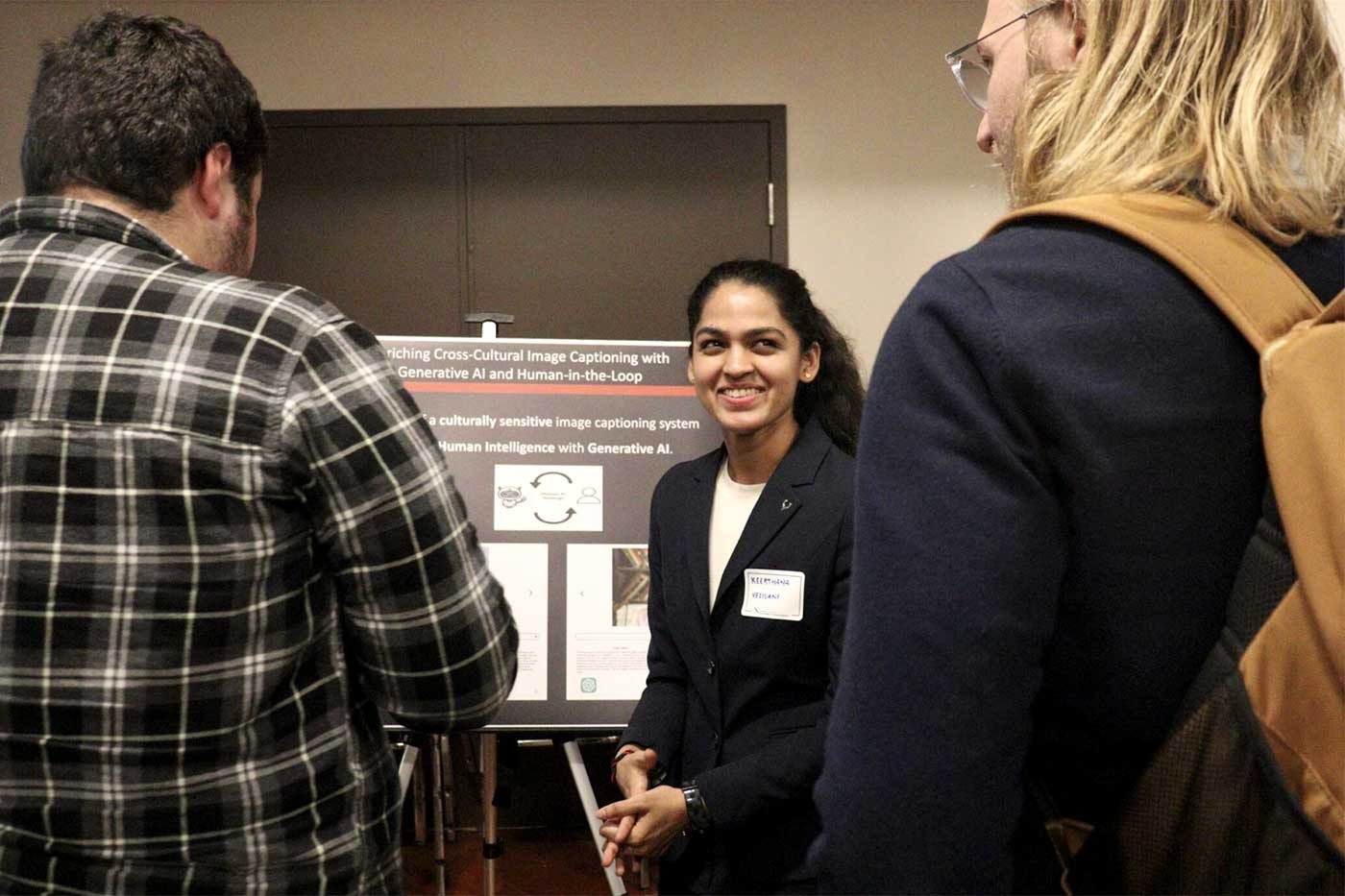
As Keerthana Velilani was familiarizing herself with ChatGPT Vision — which allows users to include images in their prompts to the chatbot — she observed a frequent issue. The AI struggled to accurately caption images that reflected different cultures, especially in pop culture.
“For example, here’s Swami [from the popular Indian novel series Swami and Friends] and he’s wearing a cap from Hindu culture in India,” Velilani explained, pointing to her poster display. “But the way the AI-generated caption describes the meaning of this cap is totally off, suggesting the cap is from Southeast Asia and from a different religion altogether…This kind of image captioning requires cultural sensitivity, so we bring humans into the loop to infuse culture-related inputs.”
Working with Assistant Professor Saiph Savage, Velilani set out to design a culturally sensitive image recognition and captioning system that integrated both human intelligence and generative AI to more accurately capture the images.
“What we found was that GPT-4V came very close to identifying the English-based series, but we saw bias against non-English TV series and pop culture references,” Velilani said. “That’s because it’s not trained on that data. It was often hallucinating and just getting the information wrong.”
In Velilani’s system, GPT-4V produces the initial captions for culturally resonant images. Then crowd workers query the AI system about the image through a question-answering interface. The AI’s response to the queries are incorporated into the caption draft and crowdworkers provide the AI with feedback on the image, including culturally relevant information that the initial caption may have lacked. Over time, Velilani hopes this model can make large language models more equitable.
Abdulaziz Suria
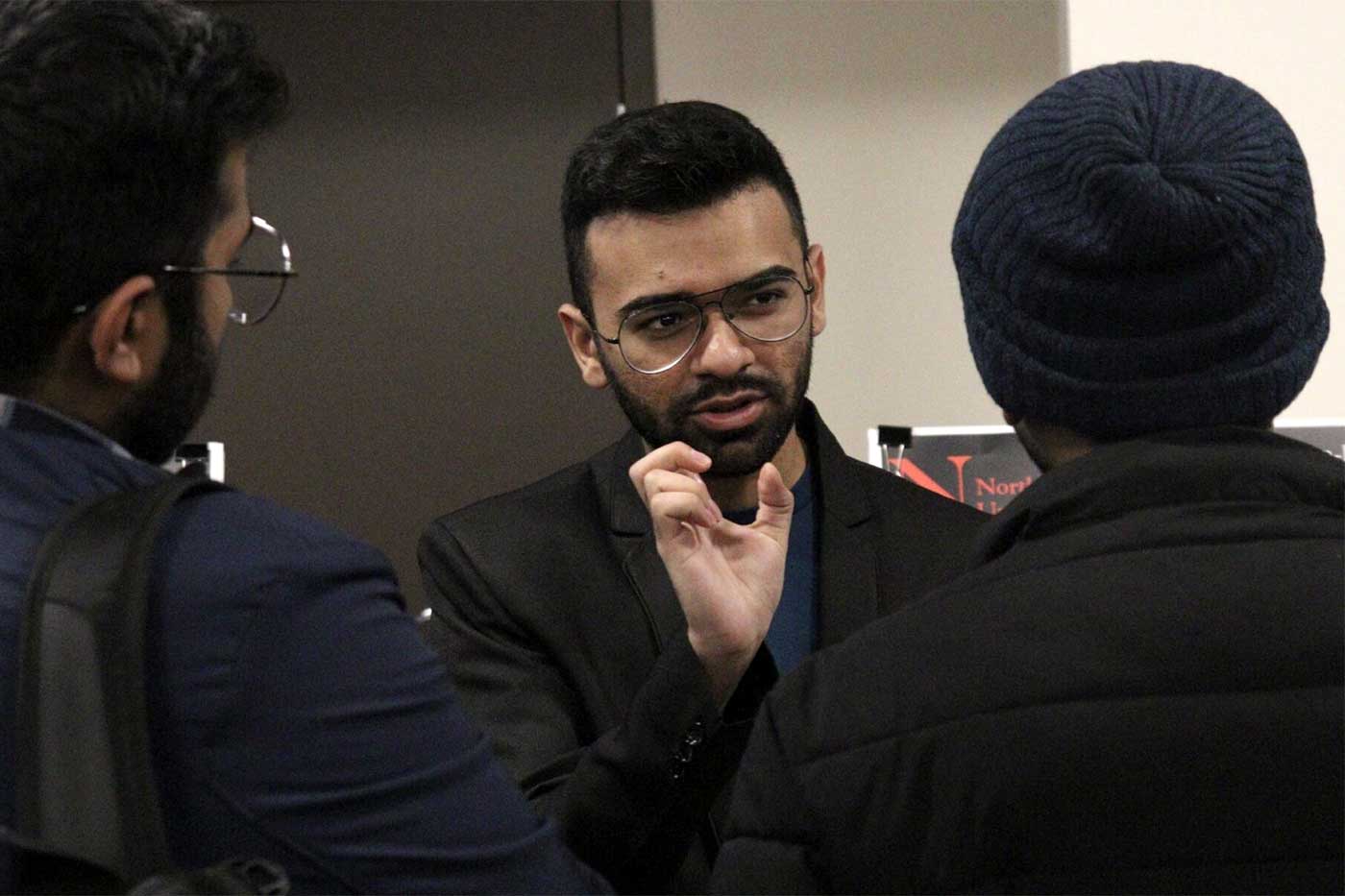
Abdualaziz Suria is an avid player of VRChat, a popular multiplayer virtual universe of self-imagined worlds and customized avatars. But he was frequently frustrated by one aspect of the game: the non-player characters, or NPCs. These are the bots that give predetermined responses based on user input. For example, Suria explains, if you ask an NPC, “Do you like pizza?” it will always give the same response regardless of context.
So Suria set out to build an AI model that made NPC interactions more like the dynamic interactions human players have with one another. In essence, if a user asks the same question to the NPC in separate instances, rather than giving the same predetermined response, the AI will generate more human-like responses. Likewise, it will take its own personality and past conversations with the user into account and provide a more creative and engaging answer. While the system was designed specifically with VRChat in mind, Suria hopes the concept can extend to other video games as well.
“When we have conversations in real life, we make observations about each other. You learn something about me; I learn something about you,” Suria said. “This is just like that. Every time you interact with a character, we store these observations, calculate the most important observations relevant to the current conversation, pass these observations to GPT, and GPT generates a response. This [creates] a new set of observations that are stored in the avatar’s memory.”
Ameya Santosh Gidh
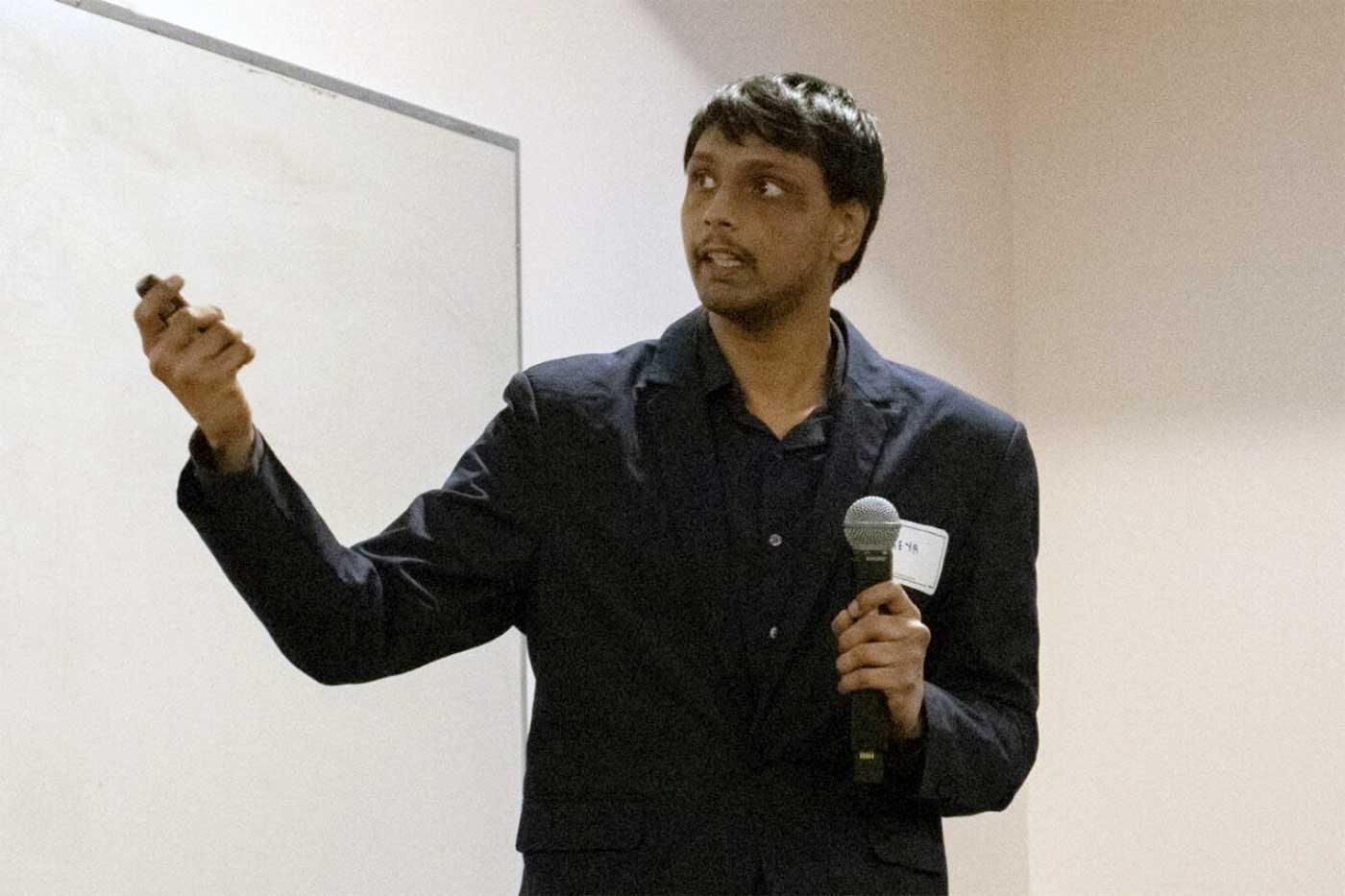
Machine knitting is a fabrication technique which uses specialized equipment to create a versatile stitch pattern. The technology is a preferred method in textile manufacturing, as it enables versatile stitch patterns, intricate designs, and a speedy, precise manufacturing process.
But machine knitting is also often convoluted, and making intricate patterns can yield errors. To help, KnitScript, a domain-specific programming language for knitting, streamlines the creation of intricate knitting patterns and simplifies the complex code into a unified and efficient output.
Working with Professor Megan Hofmann, master’s student Ameya Santosh Gidh took an open-source KnitScript library — which was created by Hofmann — and integrated it with a machine state diagram, which visually describes state-dependent behavior for an object. Gidh hopes the holistic approach will create a user-friendly environment for precise and intricate knitting instructions.
“The machine state diagram helps to address visual debugging and error handling,” Gidh said.
Leigh-Riane Amsterdam
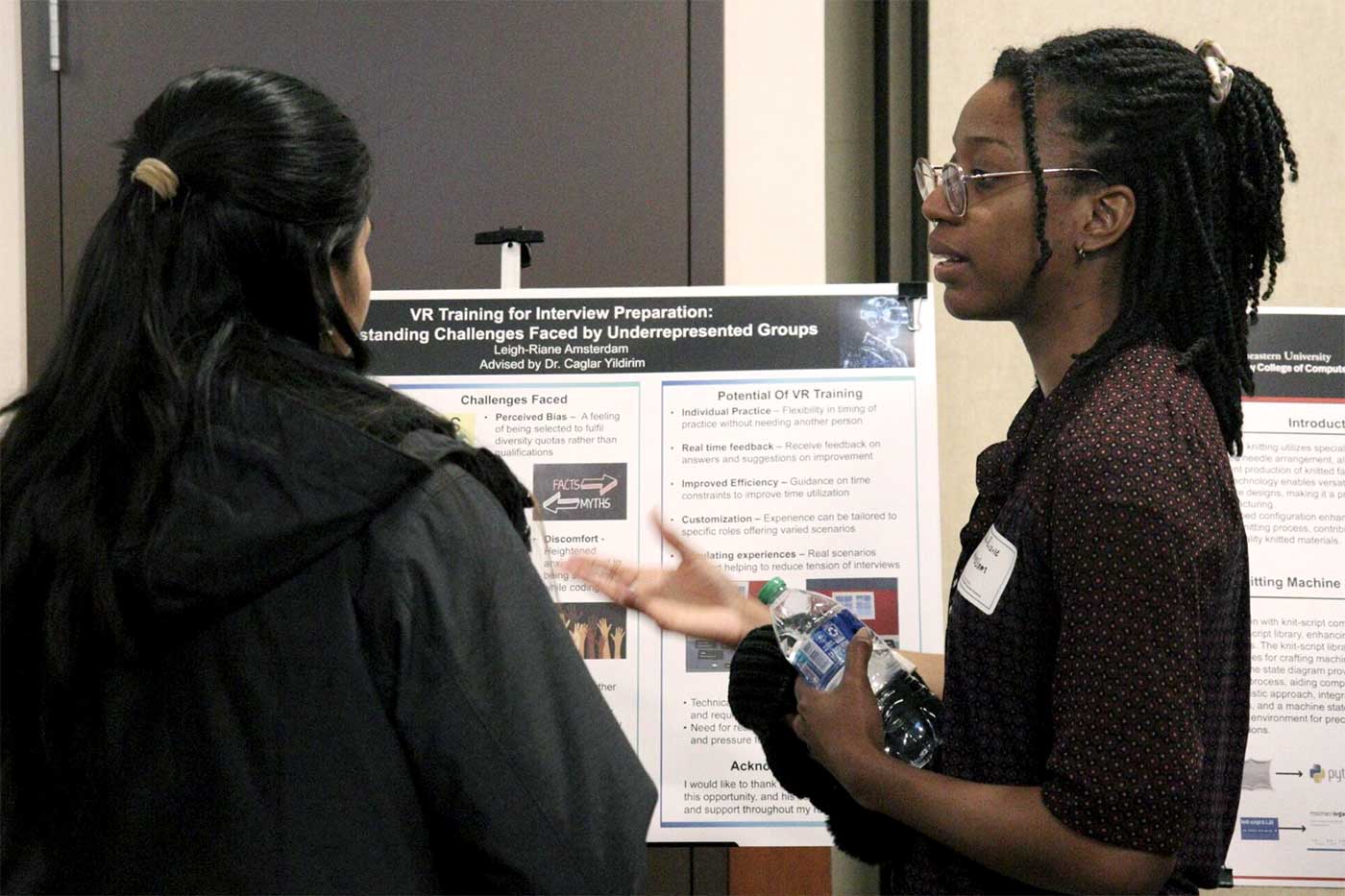
In the computer science field, job candidates from underrepresented groups often face bias and challenges during the technical interview process — a problem that Leigh-Riane Amsterdam is hoping to address with new technology.
“Being a person of color in the field, I was interested in this project because it utilized virtual reality — which is an interest of mine — and combined it with an examination of the challenges experienced by underrepresented groups,” Amsterdam said. “Many individuals from these groups are technically competent; they just may not have the tools or the experiences to navigate the technical interview hiring process, which can be difficult and stressful.”
Using a VR prototype to simulate technical interview settings, Amsterdam analyzed the challenges faced by underrepresented groups in computing. She also researched the potential for a VR technical interview training tool, which would provide real-time feedback on how users can improve their answers to technical questions, and which could be customized to specific roles.
“There are going to be challenges, but that’s where the VR training tool comes in,” she explained. “It allows participants a lot of flexibility; they don’t need to coordinate with someone else, since they can practice on their own. They can simulate a variety of different experiences and are provided with examples of what they could be asked in a technical interview, which is really important because it allows them to practice how they could respond, reducing the anxiety in the real-life scenario.”
Diptendu Kar
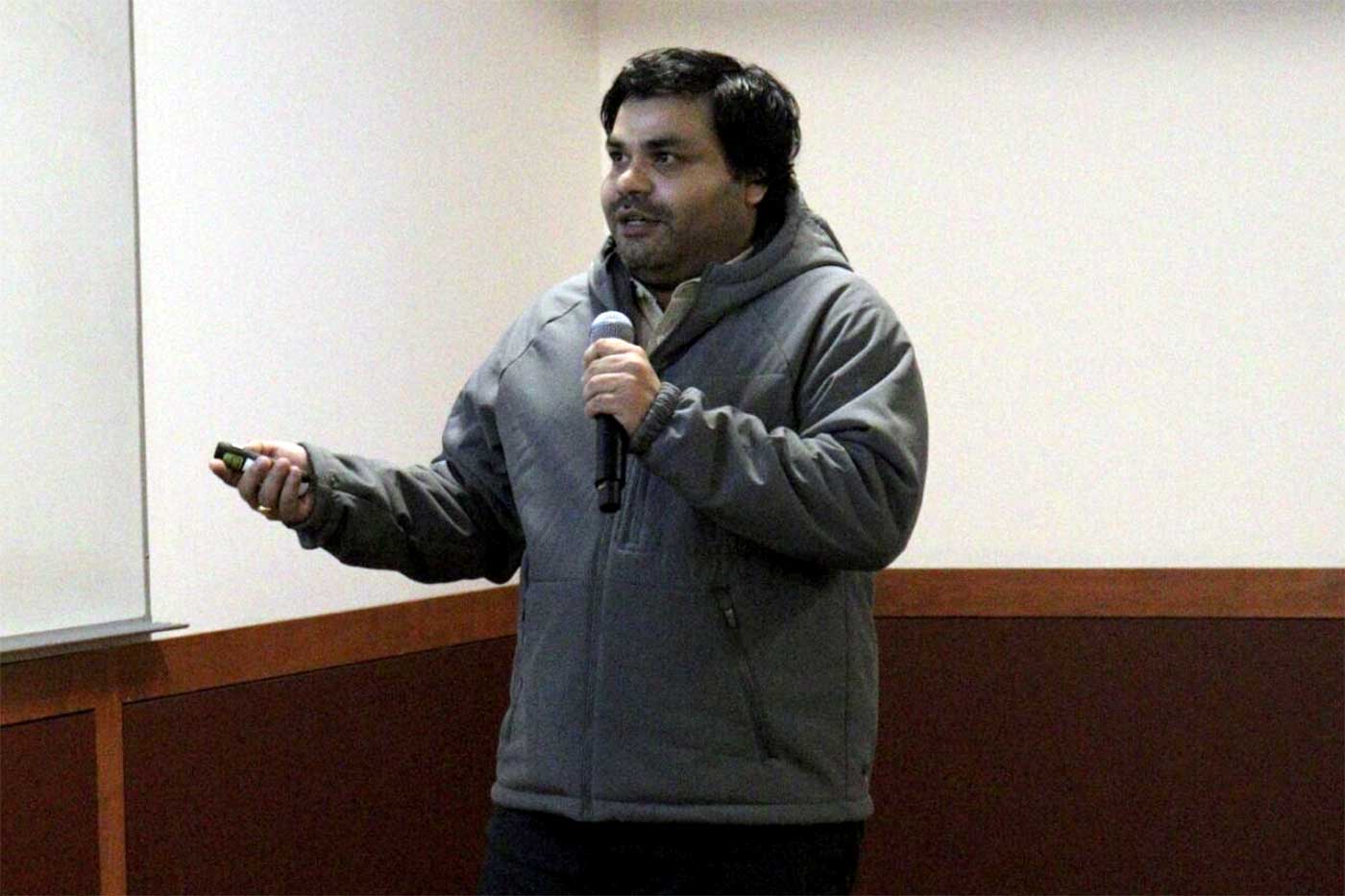
Capture the Flag (CTF) is a dynamic cybersecurity competition where teams engage in various challenges, such as finding hidden text strings in intentionally vulnerable systems, breaking into websites, or identifying software vulnerabilities. CTF competitions have long been a popular method to test technical knowledge and build problem-solving skills, including in cybersecurity courses at Northeastern.
But with the recent rise of large language models such as ChatGPT, is the playing field still level? That’s what Dipendu Kar set out to determine.
“The question we asked is, ‘If students are using large language models to solve the challenges, how do students learn anything? Or how do we assess their skills?’” Kar said. “These models have been exposed to traditional cybersecurity questions and answers, but they have not been exposed to capture the flag, so we wanted to determine the capability of large language models in solving capture the flag challenges.”
In his research, Kar tested three models, GPT 4, GPT 3.5, and Bard. He compared their performance along three parameters: the challenge domain, the amount and extent of feedback, and the year of the challenge. Of the 30 simulations run, Kar found that GPT 4 solved 22 challenges, GPT 3.5 solved 14 challenges, and Bard solved five — meaning that each of the models had a measure of success in solving the challenges, with GPT 4 being the most successful.
Juan Diego Dumez Garcia
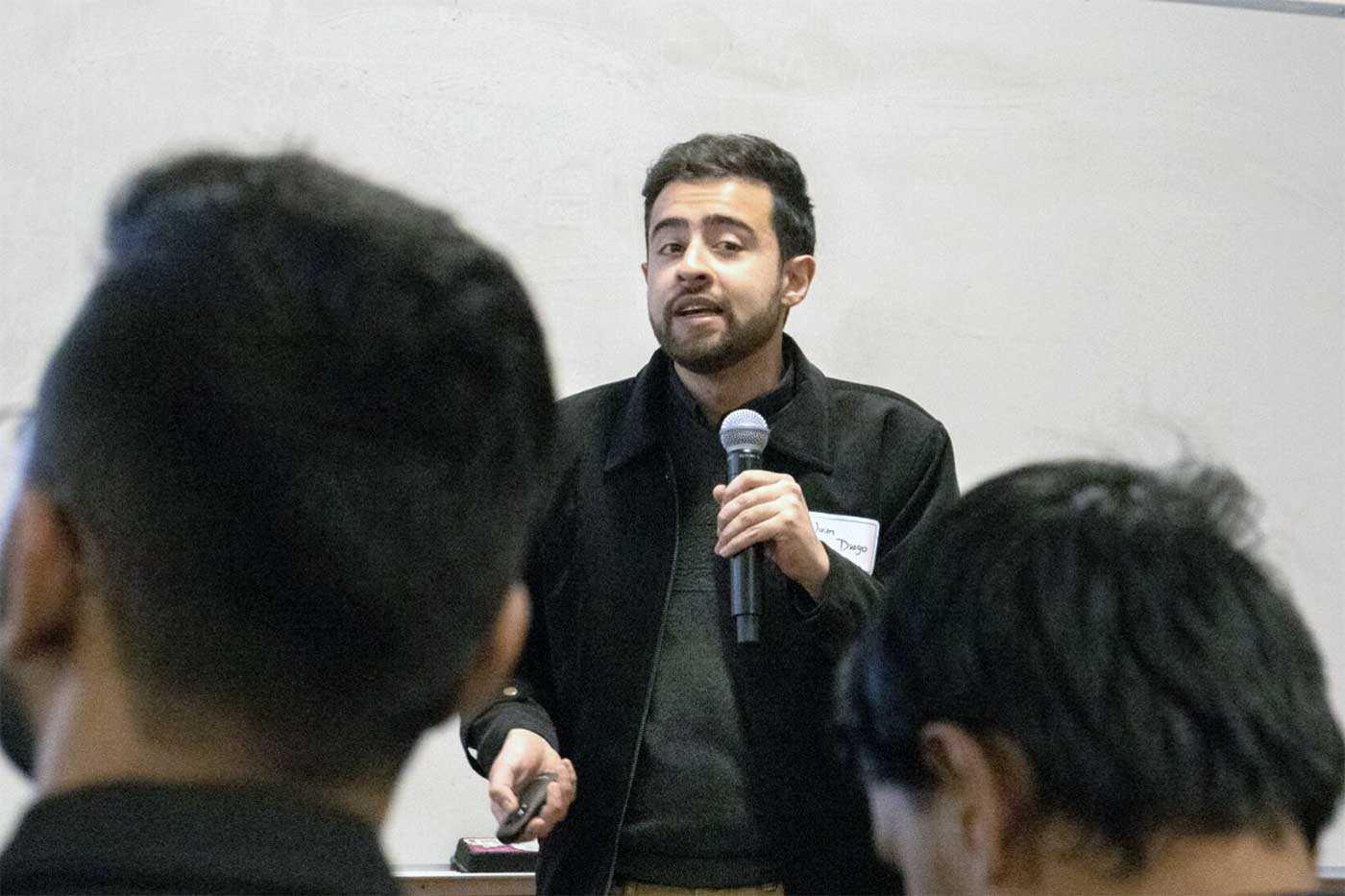
Technology that captures 3D images, such as Google Maps’ 360-degree street view, comes with a major challenge: finding space to store the ocean of images and data. As Juan Diego Dumez Garcia explains, because these panoramic images are composed of different pictures infused together rather than video technology, they necessitate workaround solutions.
“If I’m walking on a street and want to capture it in 3D, I could just take a video of all the scenes, and it’s that easy. But the burden is, if I want to store that video, just imagine the amount of data and space it will take,” Dumez Garcia said. “So nowadays … we just take pictures at different distances, and at different angles.”
The traditional model used for this process is NeFRs, a machine learning technique which represents the images in a point-cloud data, then regenerates the images together in a singular space. However, Dumez Garcia’s research attempts to apply Gaussian splatting — a classic computer graphics technique that allows for the same real-time processing, but is less computationally demanding and doesn’t require the same model training process.
After applying a detection software called YOLO to identify objects, Dumez Garcia built a scene graph database to represent scenes and objects, which the model could store and differentiate. Then he integrated spatial relationships and bounding boxes to form the finished product: an advanced query system with the back-end capability to manage complex scene data efficiently.
Tom Henehan
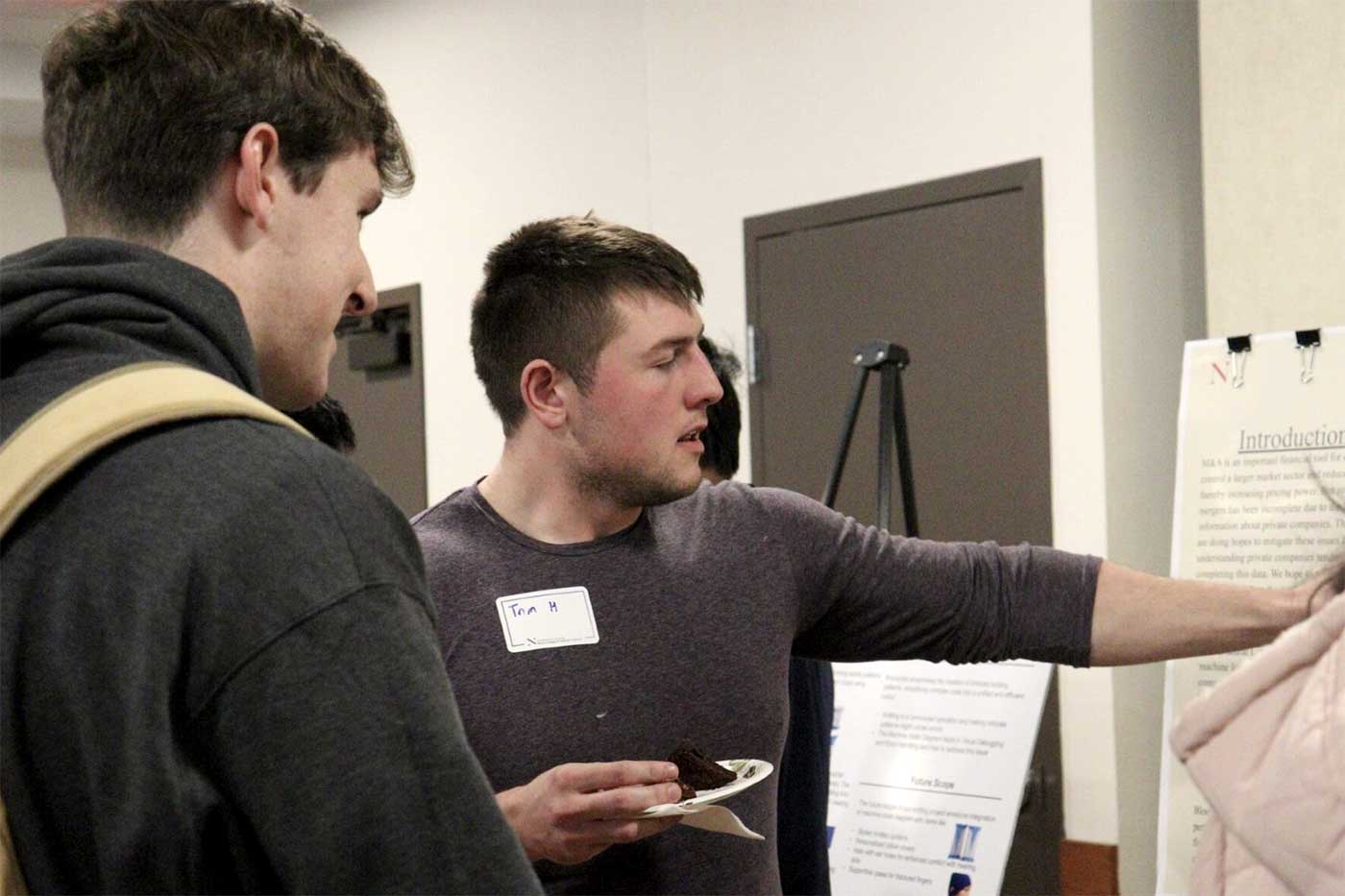
After pivoting from his undergraduate degree in economics to his master’s in data science, Tom Henehan was looking for a way to combine the two fields through a research project. By teaming up with Professor Ravi Sundaram, he found a perfect match.
Their research explored the ties between mergers and acquisitions, patents, globalization, and green energy — all in the hope of better equipping companies with information to determine whether an acquisition makes sense.
“Private companies make up a large share of the total pool of companies, but we don’t understand them well because their data is not publicly available,” Henehan said. “So because we don’t know much, we have to do all this data collection and tie it into innovation surrounding mergers and acquisitions.”
The duo focused on patents and intellectual property held by private companies, using natural language processing and Bloomberg software to locate and value them. Through this, they determined which companies were innovating with new patents and which areas of the market remained untapped. The research is still in the data collection phase, and Henehan plans to stay on board with the project in the spring.
Ruochen Liu
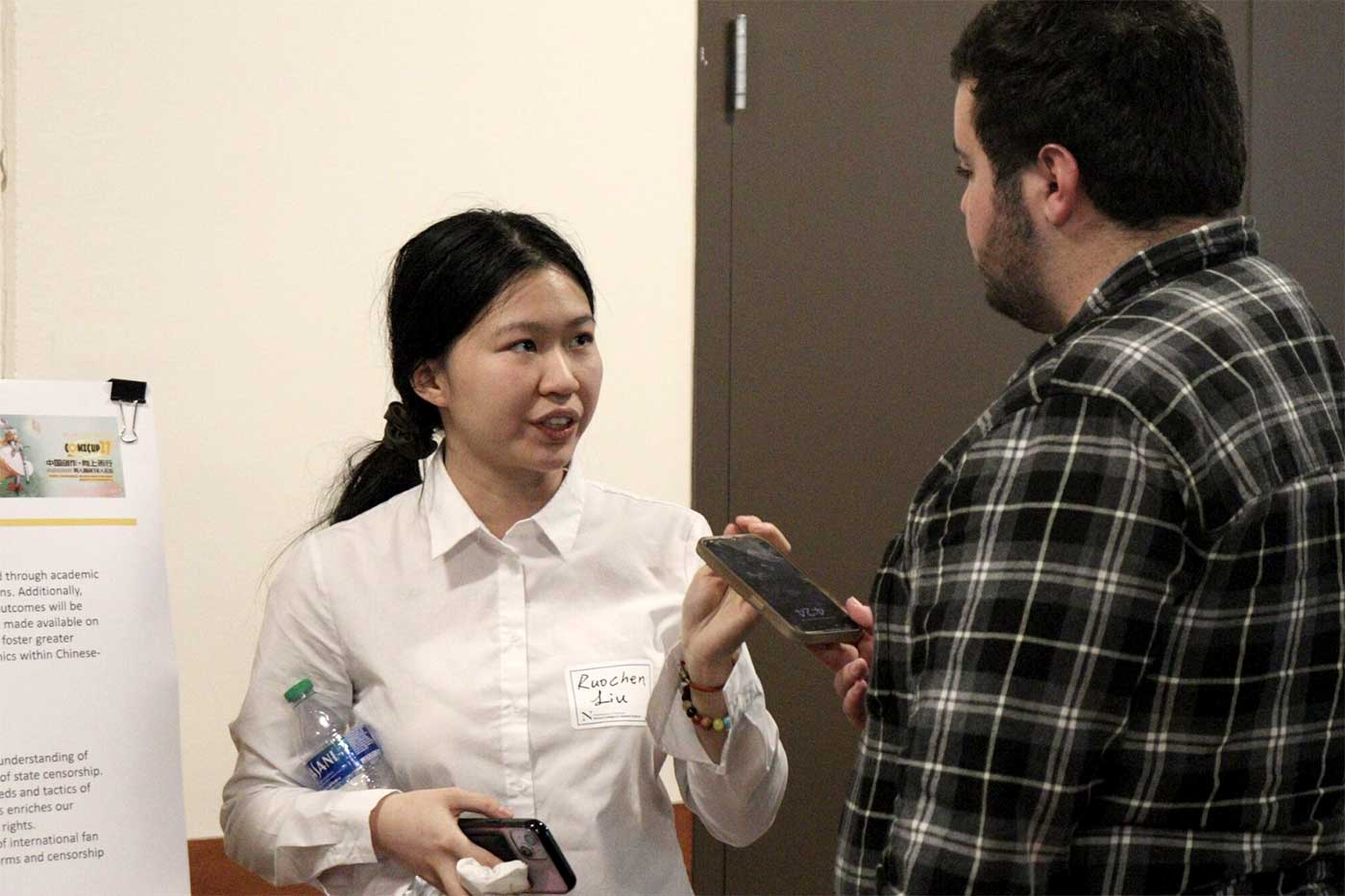
Growing up in China, Ruochen Liu experienced the challenges associated with online fandom on a state-censored internet. Chinese superfan communities are much like Taylor Swift’s “Swifties” or any other devoted American fandom, but they face content restrictions, surveillance on their posts and feeds, and limits on their internet usage.
“Every time you hit the publish button on a post [in China], there is a machine that checks if there are any key words that violate their regulations. If there is, it stops you from publishing,” Liu said. “Sometimes you can get around that by just changing a word, but if the content you make becomes widely spread, a person from the government will review and decide if they want to delete your post or even your whole account.”
In her research, Liu set out to investigate the Chinese government’s specific censorship tactics and how they impact these fandoms — all while comparing the communities to their English-language equivalents which enjoy freedom of expression. Liu interviewed 40 participants from Chinese-language online fandoms, hoping to gain a nuanced perspective on how these fandoms are suppressed and how they evolve in spite of it.
“I think this really gives me a new angle to look at user and computer interactions,” Liu said. “We are now always talking about privacy with AI, but it’s actually about every activity you do on the internet, and how it’s being shaped.”
Siddharth Chakravorty
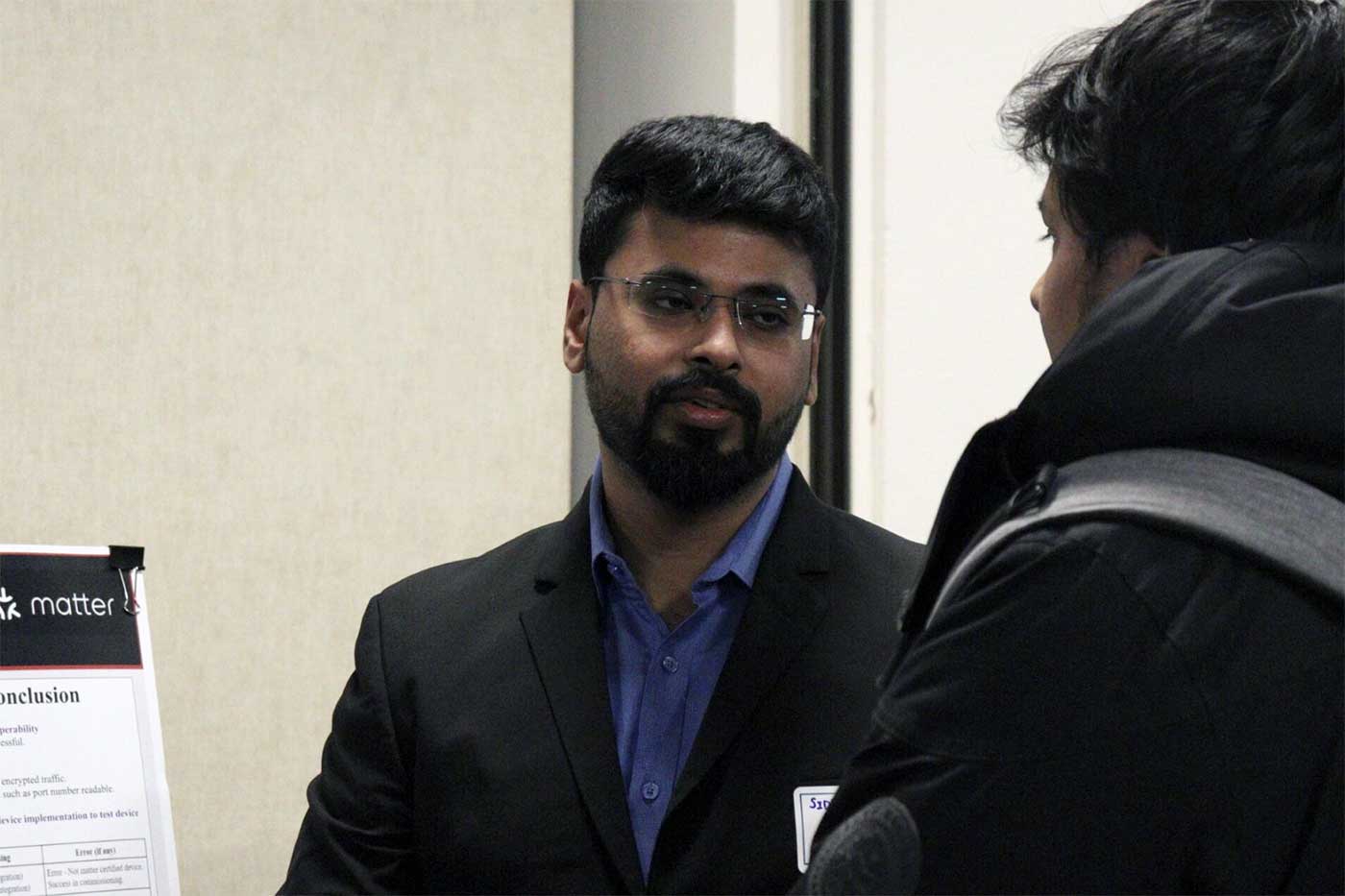
Today, Internet of Things (IoT) devices include everything from watches and medical devices to home heating systems to fridges and remotes. But what if you have a Google Home and an Apple TV? Or an Apple Watch and an Amazon Alexa?
As IoT devices spike in popularity, this issue of cross-compatibility and secure communication between brands has come to the fore. Matter, an open-source connectivity standard for smart home devices published last year, has aimed to help solve this issue by providing a common framework for vendors to interconnect. In his research, Siddharth Chakravorty set out to test Matter’s effectiveness in promoting this interoperability between devices, along with how well each prominent IoT smart home product performed.
“The most important step is the commissioning process, where a device gets provisioned into the fabric [of the network],” Chakravorty explained. “My main research was focused on whether the vendors follow what the Matter standard says. And I got pretty much the results I expected; these are big players, and they almost all comply well with the standards.”