Assistant teaching professor Divya Chaudhary pioneers novel melanoma detection method
Author: Emily Spatz
Date: 1.7.25
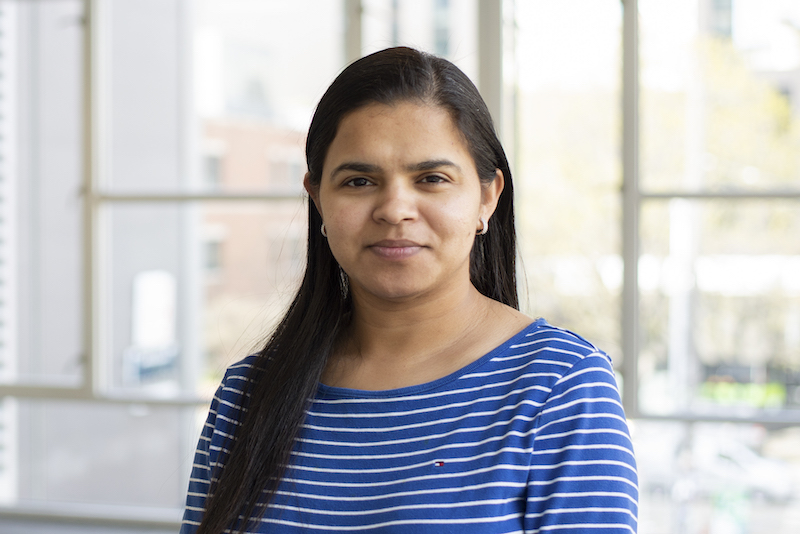
Divya Chaudhary, an assistant teaching professor at Khoury College in Seattle, has developed an advanced and more accurate method for detecting melanoma. This innovative approach enhances segmentation accuracy and classification efficiency, aiding medical practitioners in diagnosing and treating skin cancer while preserving surrounding healthy tissue.
Along with Peng Zhang, a master’s student in computer science at Northeastern’s Seattle campus, Chaudhary published her findings at BIOKDD 2024 — held in conjunction with SIGKDD 2024 — in Barcelona, Spain. Together, they leveraged existing deep learning models to create a hybrid technique for detecting and identifying melanoma, a form of skin cancer.
“The hybrid technique could allow medical practitioners to more accurately classify cancerous and noncancerous cells in the body,” Chaudhary said. The technique can help damage fewer cells and localize the target of radiation so healthy cells are not impacted. Its performance highlights the potential of deep learning models to enhance early detection, providing a promising pathway to improve patient outcomes and shrink mortality rates associated with skin cancer.
While Chaudhary spends most of her time teaching, she has always been motivated to pursue research. Her main goal is to inspire both herself and her students to enact positive change in society, so she has worked on transphobia, depression, suicidal tendencies before and after COVID-19, hidradenitis suppurativa, and Alzheimer’s disease.
“I have always been fascinated by artificial intelligence and its potential to benefit society,” she said. “My goal was to pursue innovative and impactful research that not only supports students but also contributes to society. This work inspires both my students and me, fostering a research-driven mindset on the Seattle campus and across Northeastern, encouraging students to consider research as a career path.”
In the melanoma study, Chaudhary and Zhang utilized two existing convolutional neural network architectures, U-Net and EfficientNet. U-Net was employed for image segmentation, dividing the image into pixel groups, while EfficientNet was used to classify whether the abnormal cells were cancerous.
“We used the power of both models to generate a hybrid model, and fine-tuned it,” Chaudhary said.
The hybrid technique boasts 99% accuracy with both models for melanoma detection. Individually, EfficientNet and U-Net have addressed various classification and segmentation problems like pneumonia, tuberculosis, and lung cancer, but together, they had never achieved such an accurate binary melanoma classification.
“If doctors know the cancer’s exact location, they can target that specific region and … minimize damage to the body,” Chaudhary said. “So, our goal was to have a more accurate diagnosis that will effectively help doctors and practitioners target which regions are cancerous.”
To expand the accessibility of the model, Chaudhary plans to build an application and submit it to Northeastern’s Center for Research Innovation, which supports researchers in securing intellectual property protection and building corporate relationships. She is also organizing a workshop on generative AI and large language models for health at the 2025 AAAI Conference on Artificial AI, to be held this coming February and March.
The Khoury Network: Be in the know
Subscribe now to our monthly newsletter for the latest stories and achievements of our students and faculty